How to Maximize the Value of Your Metadata
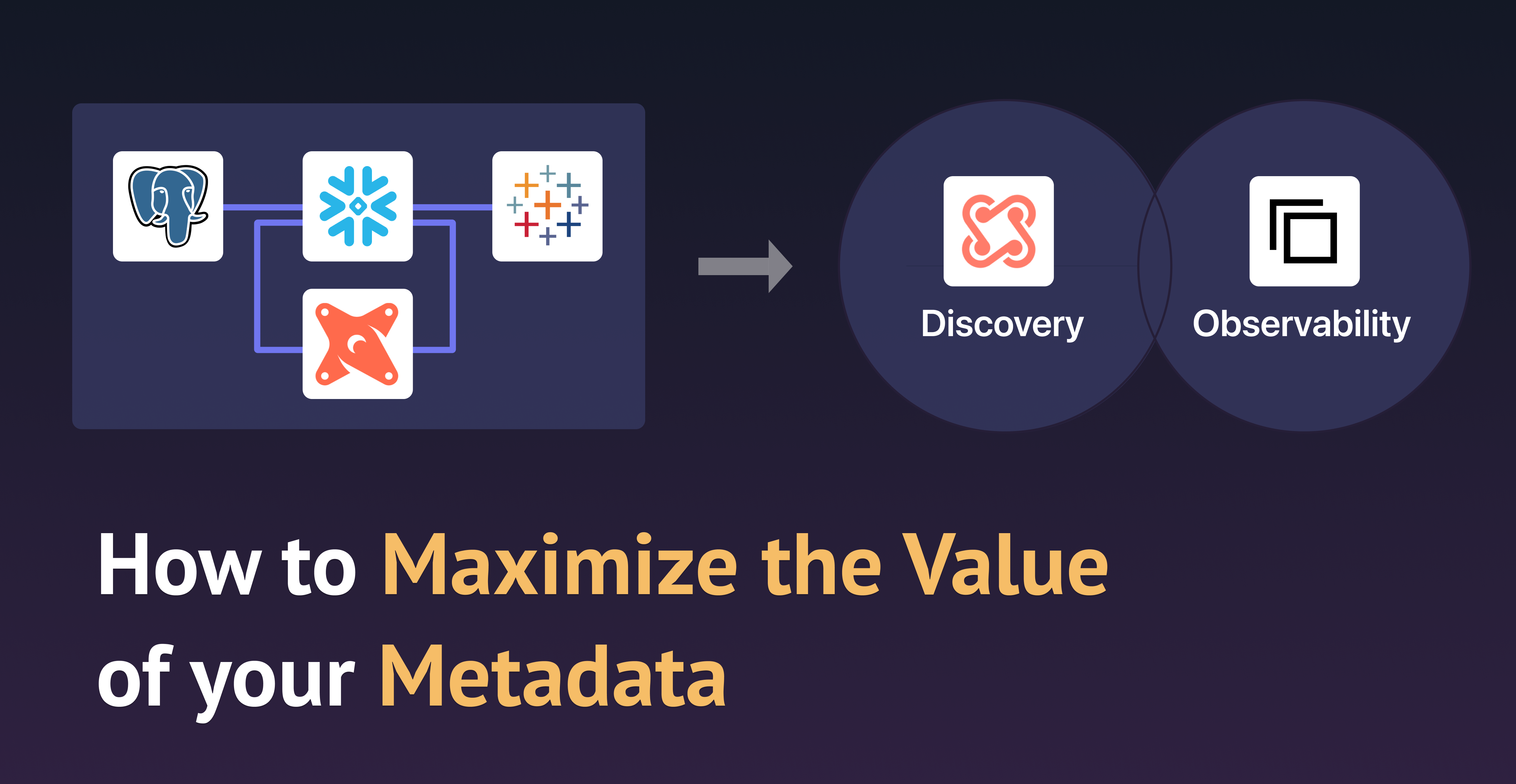
What is metadata and why does it matter?
Your data team provides data for other teams to make decisions and take action. That could mean using a Reverse ETL tool to send product usage data for a customer support team to provide better support, a dashboard to help executives make decisions, or providing features for training machine learning models.
Ironically, data teams frequently don’t have the information to help ourselves to make decisions and take action in a data-driven way. We need data about the data we provide, also called metadata. For example, which tables are being relied upon the most by end users? What is the business definition of this metric? Are any ETL pipelines delayed?
Answers to these sorts of questions are increasingly important as data is becoming a product used beyond simple reporting to power a wide surface area of applications. But most teams today are building products without documentation or analytics. Without documentation, it’s as if the data team is a product team who also handles support tickets. Without analytics, the usage and impact of your product is unknown, as are the results of your efforts.
The first problem that metadata can help solve is that of inbound requests. If you’ve worked in a data org for even one day, you know how relentless and disruptive these requests can be, whether they’re questions about the definition of a field, how to access a certain piece of data, a request to create an extract, or anything else. Some of these requests are important and are fundamental to a data team’s purpose: to empower the rest of the organization. Many of them are unnecessary.
The second problem that metadata can help solve is that of incident management. Data can have bugs just like software, whether it’s a metric being skewed or a table arriving late. Unlike for software engineers, data teams frequently don’t have the tools to identify incidents, diagnose the root cause, and analyze downstream impact.

What problems can metadata solve?
Trying to find an error in a database is like trying to find a needle in a haystack. While it is possible to search through the data until you find what you're looking for, this could take a long time and will likely be inaccurate. Instead, metadata helps us view our data more clearly, enabling us to find the root of problems and develop an understanding of our data quickly.
Instead of manually searching for the answer, metadata can reveal problems we didn't know existed. Sometimes, these problems are blindingly obvious, but sometimes it takes a bit of thinking and investigation to figure out what's wrong.
In addition to metadata, lineage provides visibility into where and by whom our data is being accessed. Metrics can also give us insight into how our data is being used. Usage metadata displays details of each time the data was accessed, how often it was accessed, and by whom. Employees can learn to use data faster and be confident that the most relevant data is being used to make decisions.
Metadata also helps us cut down on the amount of time we spend debugging observability logs for each problem. Without observability tools, it's difficult to determine what's broken, how it affects other systems, or troubleshoot the problem. With metadata, teams can be confident that the data they're working with is accurate.
How do you actually use metadata productively?
Metadata management can be effectively managed with the "people, process, technologies" framework. Before getting started on the process portion of the framework, it’s important to work collectively with your team to identify what you actually want to improve
using metadata. Some common use cases are data discovery, data observability, data lineage, and data governance. Depending on your team size and on the size of your data sets, you may find yourself needing to focus on one of these areas more than others.
To begin to use metadata productively, you will need to collect all the right metadata from different data sources. Even though most metadata resides in the data warehouse, looking at metadata generated by your warehouse alone will not give you the complete picture. Prior to analyzing metadata, teams should gather all the information from their business intelligence, transformation, warehousing, and orchestration tools into one central location. Once the metadata has been collected into a central location, teams should think about documenting as much of the metadata as possible. Many tools automate documentation directly from metadata, which simplifies this process. In spite of great tools, there will always be a portion of metadata that requires manual documentation.
Keeping your data documentation up-to-date and relevant for your team requires data platform leads and data product managers to assign ownership to different stakeholders. When documentation or schema in any resource they are responsible for changing, certain tools help notify these resource owners. Adding metadata to workflows is one way some teams have gotten started using metadata productively. If metadata is integrated into your workflow, you can keep track of changes to metadata and data directly in tools like Slack. Teams that have been successful in using metadata productively have also created processes around updating and using metadata that involve multiple stakeholders. For example, one data lead might be responsible for tracking the incidents caught by an observability tool, while another would be responsible for logging key metrics in a data discovery tool. We recommend creating ownership by domain, schema or tool
, depending on the size of your data organization. At the end of the day, as long as it’s someone's problem it’s probably good
thing.
Having created a process for managing metadata and assigned ownership to different team members, you can begin to measure the impact of incorporating metadata into your workflow. Observability tools can be used to measure the amount of data issues or downtime that your team encounters as a result of bad data arising during a given cycle. If your goal is to improve data discovery throughout the organization, you might also consider measuring the data NPS, as we suggested in one of our previous articles. By holding yourself and others accountable to this process, your team will greatly benefit from adding metadata into your workflow.
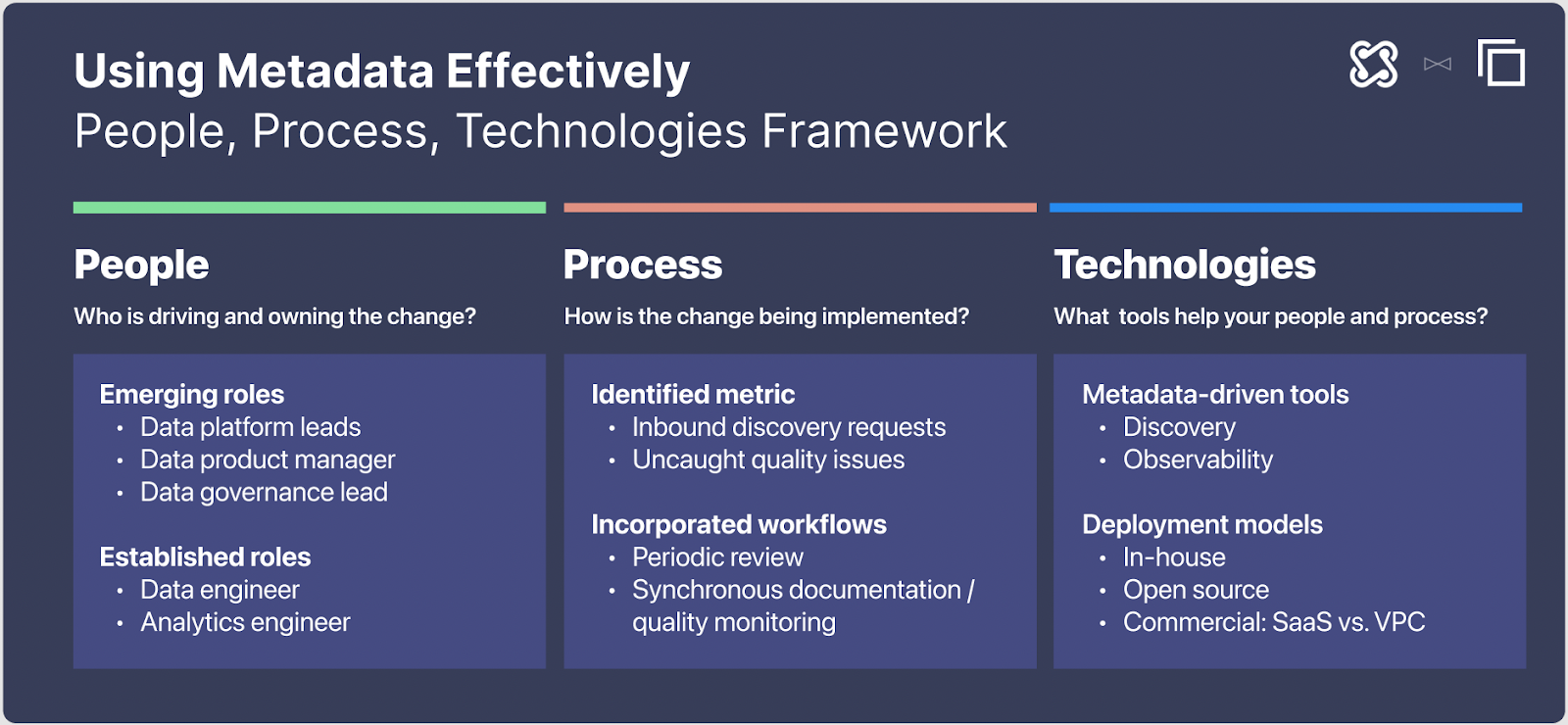
What are the different kinds of metadata-driven tools (MDT)?
The foundation of the modern data stack is built from tools such as data warehouses and business intelligence tools that extract, load, transform, and operationalize data. In contrast, metadata-driven tools are powered from metadata generated from the data stack.
The two categories of these metadata-driven tools (MDTs) are data discovery and data observability tools. The first category of data discovery tools address the use cases of documenting the meaning of different pieces of data in a sustainable and automated way, as well as compliance with security or privacy standards.
The second category of data observability tools address the use case of monitoring for data quality issues, helping analyze downstream impact, and presenting enough context and upstream dependencies to help debug.
Data discovery and data observability tools are not mutually exclusive. At their core are the same four pillars: metrics describing the internal characteristics of data, metadata describing the external characteristics of data, lineage describing the dependencies within data, and logs describing interactions of data systems with the external world.
Ultimately, the goal of these two categories of tools are the time: to help data teams save engineering team and increase organizational trust in data.
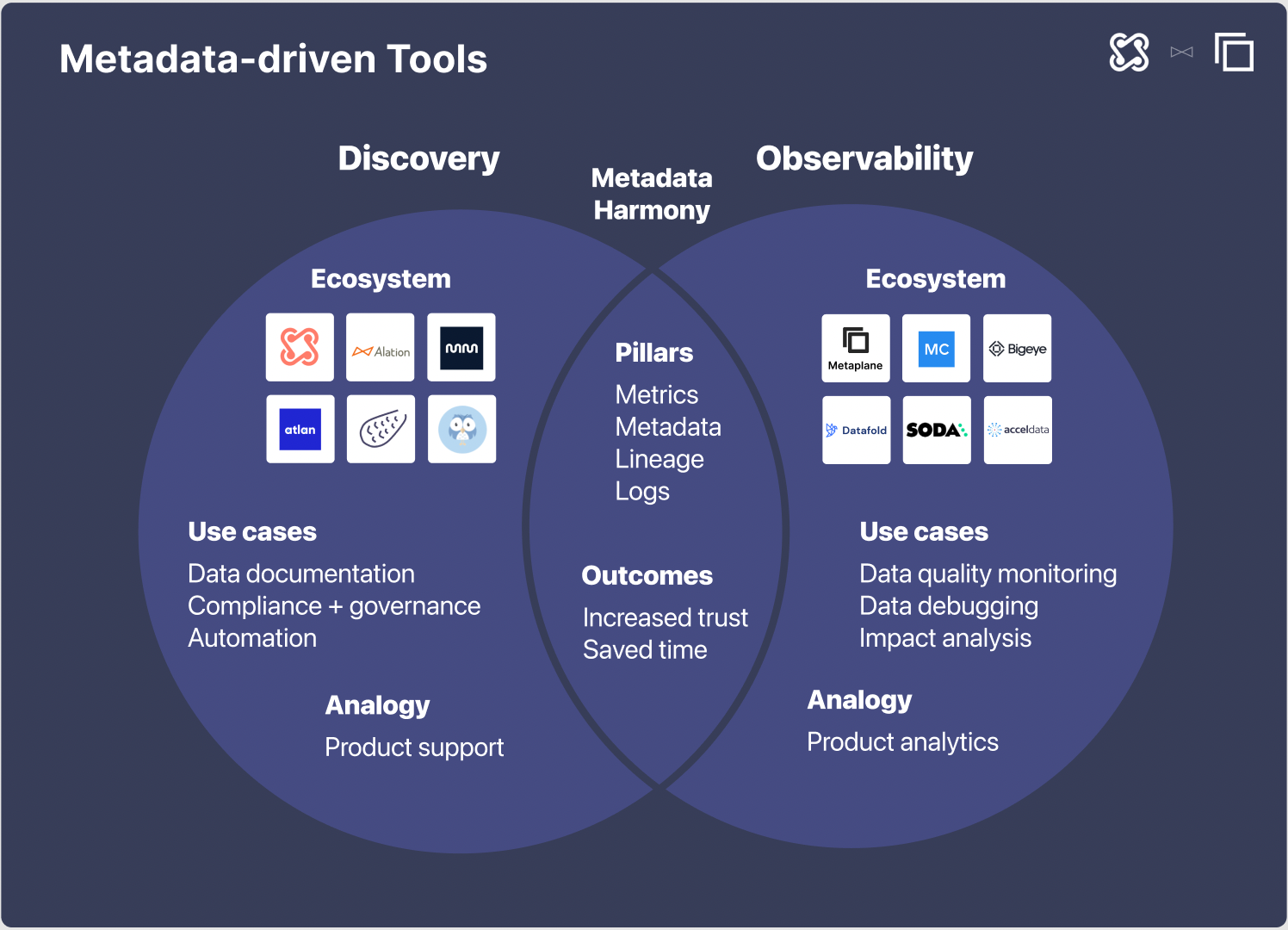
What should you look for in metadata-driven tools?
There are several dimensions along which MDTs can be evaluated. The first dimension is how much time and effort the tool saves. One component is the ease with which the tool can be adopted. Is the tool self-service so you can implement it yourself? Or are there hurdles to jump through before seeing the tool on your own data stack? There are significant differences between how easily different MDTs can be adopted, so be sure to find the right fit for your team.
Another aspect of time saving is the degree to which the tool automates the tasks of collecting and synthesizing metadata. Most MDTs propose to automate tasks such as syncing information schema from a database, but may require manual annotation of other pieces of metadata, such as lineage.
The second dimension is how the MDT integrates with relevant tools and workflows. Does the MDT provide a holistic view over all relevant tools in your data stack, from data sources to transformation tools to the warehouse to BI tools? Or does it only integrate with a subset of your data stack? You may also have different workflow tools such as Slack, e-mail, Datadog, and Pagerduty. Does the MDT integrate with all of the places that your organization works? Lastly, does the MDT provide an API to integrate with your development.
The third dimension is how the MDT fits into our organization. If your team has security requirements such as SOC 2, GDPR, or HIPAA, it’s important for all tools that touch your data to be in compliance. Note that unlike most data tools, MDTs frequently only access and store metadata and summary statistics, not sensitive information, which reduces the security risk of bringing on an MDTs. In terms of timing, does the MDT fit into your organization’s priorities and maturity model?
How do you get started?
Getting started with data discovery and data observability tools depends on the needs of your organization. Specifically, MDTs are available as both open source and commercial offerings, and it’s entirely possible to build them in-house. To decide which path to go down, a good first step is identifying your constraints. Which requirements do you need to fulfill, how quickly do you want to solve your problems, and what costs are you willing to pay? Expanding on the cost point, it’s important to not only consider upfront costs like the price of a commercial contract, but also the opportunity cost of not bringing on an alternative tool or investing your time elsewhere, and the ongoing maintenance cost of a tool.
For teams with formidable customization requirements (e.g. bespoke connectors) and the engineering resources to dedicate to both development and maintenance, it may be worthwhile to explore open source offerings or building in-house. In addition to customizability, these two routes give you complete control over how the software is deployed and transparency into the code being run.
Teams who are looking to solve problems quickly and do not necessarily have the capacity to build in-house or bring on an open source tool are probably better off exploring a commercial offering. Within the large number of vendors, it’s important to distinguish between sales-led vendors and product-led vendors. Sales-led vendors often engage with your organization from the top-down and have sizable contract values. In contrast, product-led vendors frequently offer self-serve products with approachable price tags that scale with how much value you get out of the product. For data discovery and data observability, two of the leading self-serve offerings are Secoda and Metaplane, both of which you can start using in less than 15 minutes.
Summary
- Metadata helps address problems like inbound requests and incident management.
- A People, Process, Technologies framework can be used to understand how to use metadata.
- The two main categories of metadata-driven tools (MDTs) are data discovery and data observability tools.
- When evaluating MDTs, it’s important to understand its degree of automation, depth and breadth of integration, and how it fits into your organization.
- Several MDT offerings exist, from open-source to sales-led and product-led commercial offerings.
Table of contents
Tags
...
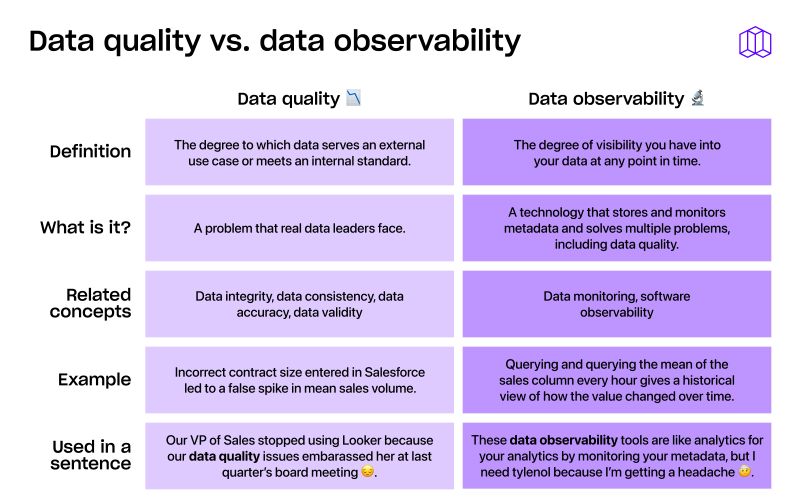
...
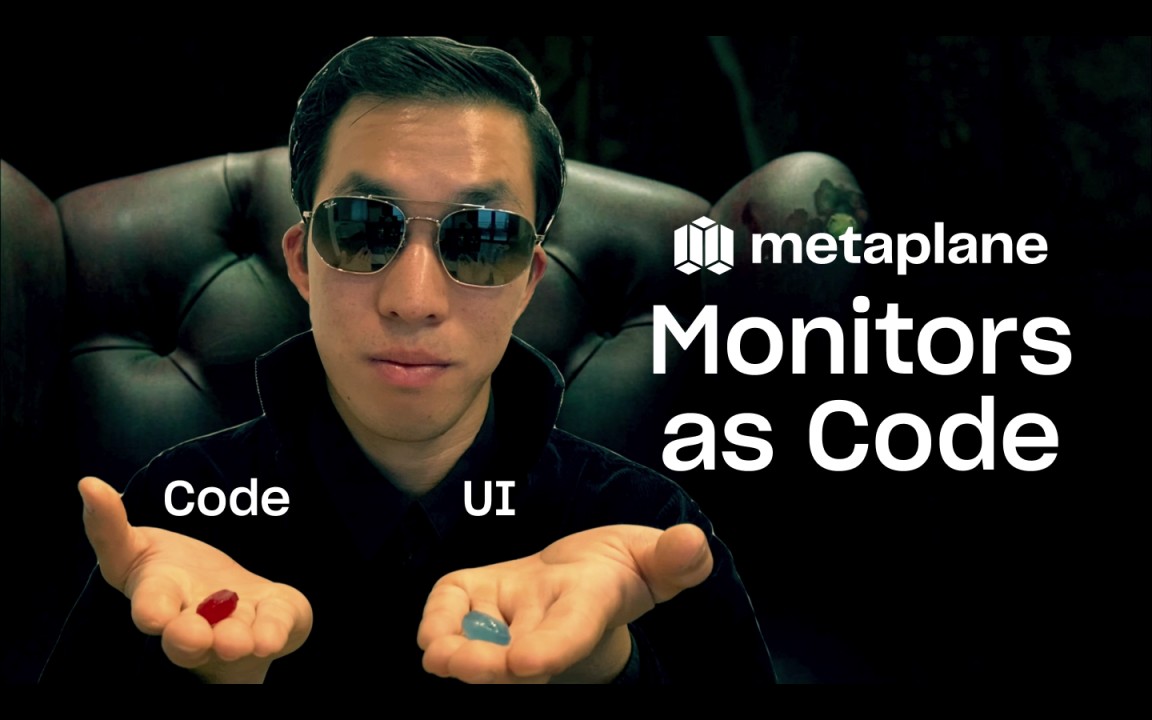

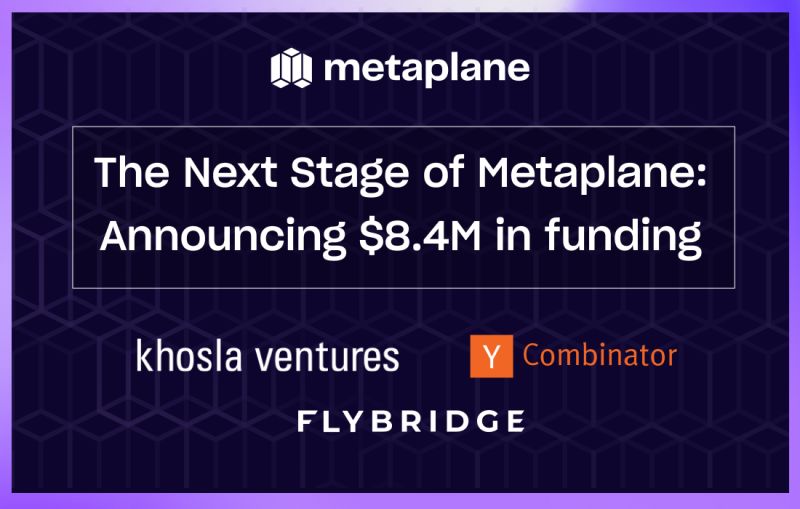
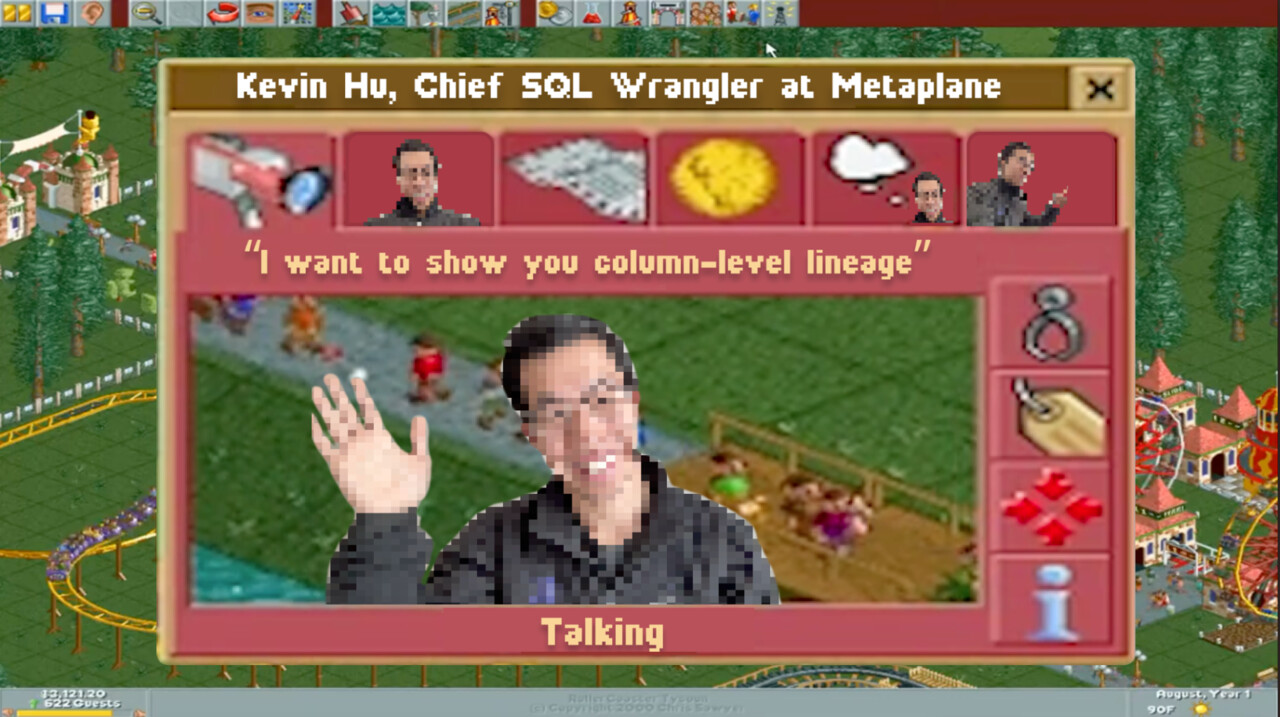
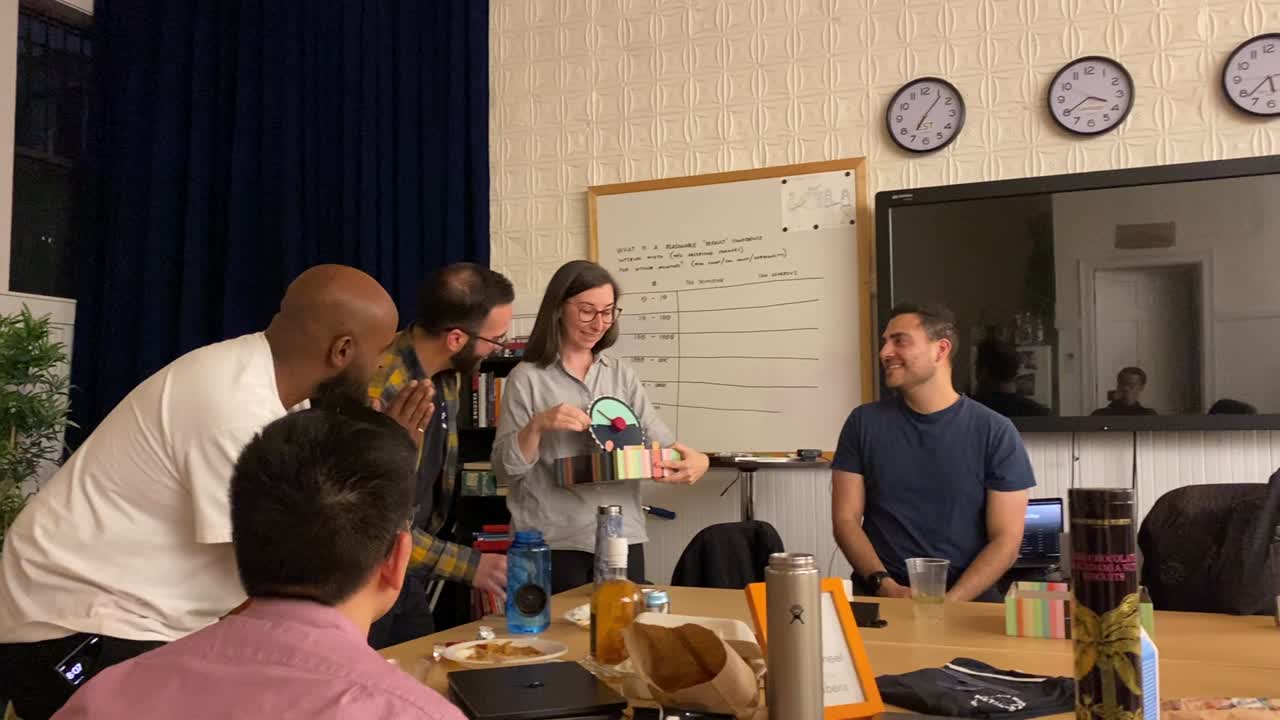