Best Data Catalogs: An Evaluation Guide
Have you ever struggled to find the right data when you need it the most? You're not alone. The sheer amount of data that companies generate can make finding the right data at the right time a daunting task. This is where data catalogs come in. In this post, we'll explore what data catalogs are, why they're important, and how to evaluate them to find the best one for your data needs.

What are Data Catalogs?
A data catalog is an organized and searchable inventory of the data assets in an organization. It provides a comprehensive view of the available data, its lineage, and its metadata. The catalog also includes information on who owns the data, its usage, quality, and relationships with other data assets.
The importance of data catalogs cannot be understated. A data catalog provides a single point of truth for data, making it easy for data engineers, analytics engineers, and data leaders to search, discover, and access various data assets in a timely and efficient manner. This saves valuable time and prevents redundancy in data engineering and analytics tasks.
To illustrate the value of data catalogs, let's consider an example scenario. A company wants to develop a new machine learning model to improve the churn prediction of its customers. However, the data scientists don't know which data sets to use to train the model. They can use a data catalog to search and filter all customer-related data sets, check their lineage, and select the most relevant and reliable ones for their project.
Evaluating Data Catalogs
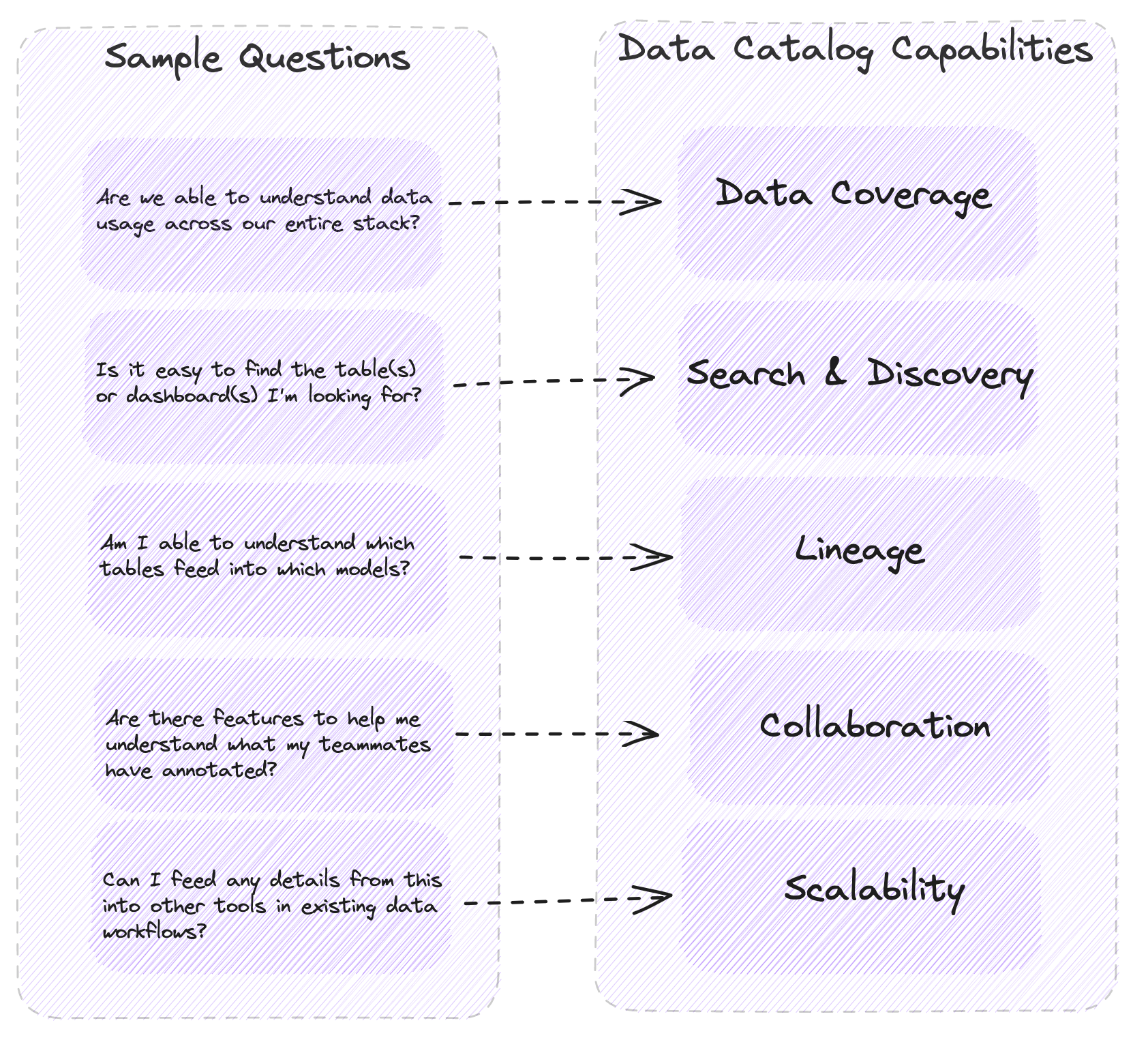
When it comes to evaluating data catalogs, you need to consider several factors to ensure that you get the most value from the catalog. Here are some key factors to consider:
Data Coverage
The first factor to consider is the data coverage of the catalog. How comprehensive is the catalog? Does it cover all data assets in your organization, or just a subset? Does it include both structured and unstructured data? A good data catalog should provide an extensive coverage of data assets, regardless of their type and origin.
Search and Discovery
The second factor to consider is the search and discovery capabilities of the catalog. Can you search for data assets using different criteria, such as keywords, data type, owner, or lineage? Can you preview the data and assess its quality before accessing it? A good data catalog should provide flexible and user-friendly search and discovery features.
Data Lineage and Metadata
The third factor to consider is the data lineage and metadata management capabilities of the catalog. Can you track the origin, transformation, and lineage of each data asset? Does it provide comprehensive metadata information, such as data profiling, data quality, and data privacy? A good data catalog should provide robust and reliable metadata management and lineage tracking capabilities.
Collaboration and Community
The fourth factor to consider is the collaboration and community aspect of the catalog. Does it enable collaboration and sharing of data, metadata, and insights among the users? Does it have a community of users who can contribute to the data assets and the catalog itself? A good data catalog should foster collaboration and community among users, making it a hub of data expertise and knowledge.
Scalability and Integration
The final factor to consider is the scalability and integration capabilities of the catalog. Can it scale up and down to meet the changing needs of the organization? Does it integrate with other data tools and platforms, such as data lakes, ETL, BI, and ML? A good data catalog should be scalable, flexible, and interoperable, to integrate seamlessly with a variety of data tools and platforms.
3 Top Data Catalogs in 2023

The following are ranked in alphabetical order.
Alation
One of the key strengths of Alation is its collaborative data catalog features. It allows users to contribute and share knowledge about data assets, including data definitions, usage guidelines, and business context.
Atlan
A key strength of Atlan is its strong focus on collaboration and providing a collaborative workspace for teams working with data. Atlan is one of major players in the space, and espouses strengths based on its usage of active metadata to programmatically generate results, such as lineage, for users.
Castor
Castor is a newer entrant to the market, and prides itself on an elegant interface, the locking of documentation between tools, the query history functionality, and the Autodoc functionality.
Data Catalogs vs Data Observability Tools
- Data catalogs focus on metadata management and discovery, providing a centralized repository for data assets, their attributes, and relationships, facilitating data reuse and governance.
- Data observability tools monitor and measure the quality, performance, and reliability of data pipelines, offering real-time visibility into data workflows, identifying issues, anomalies, and deviations.
- Data catalogs target data consumers, enhancing data discovery and collaboration, while data observability tools are used by data engineers and operations teams to ensure the reliability and efficiency of data processing and delivery.
Data catalogs and data observability tools both solve for data quality challenges and encourage greator adopton for more data use cases. In an (over)simplification, data catalogs show you what data you have, and data observability tools tell you what's wrong with the data you have.
Conclusion
Data catalogs are a must-have tool for any organization that wants to manage its data assets effectively and efficiently. By providing a centralized and searchable inventory of data assets, data catalogs save time and resources and enable data-driven decision-making. When evaluating data catalogs, it's essential to consider data coverage, search and discovery, data lineage and metadata management, collaboration and community, and scalability and integration. By choosing the right data catalog, you can unleash the full potential of your data assets and maximize their value.
Table of contents
Tags
...

...

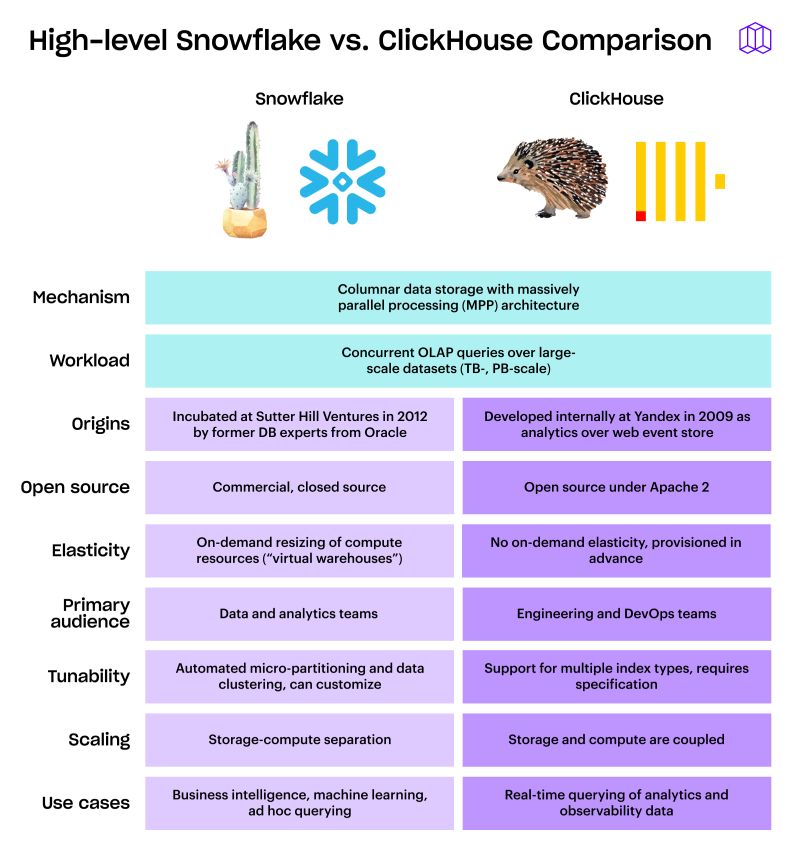


