Data Reliability Engineering: A Guide to Ensuring Data Quality in the Modern Data Stack
In this blog post, we'll define what data reliability engineering is, discuss its importance, and cover best practices, benefits, challenges, and pitfalls.
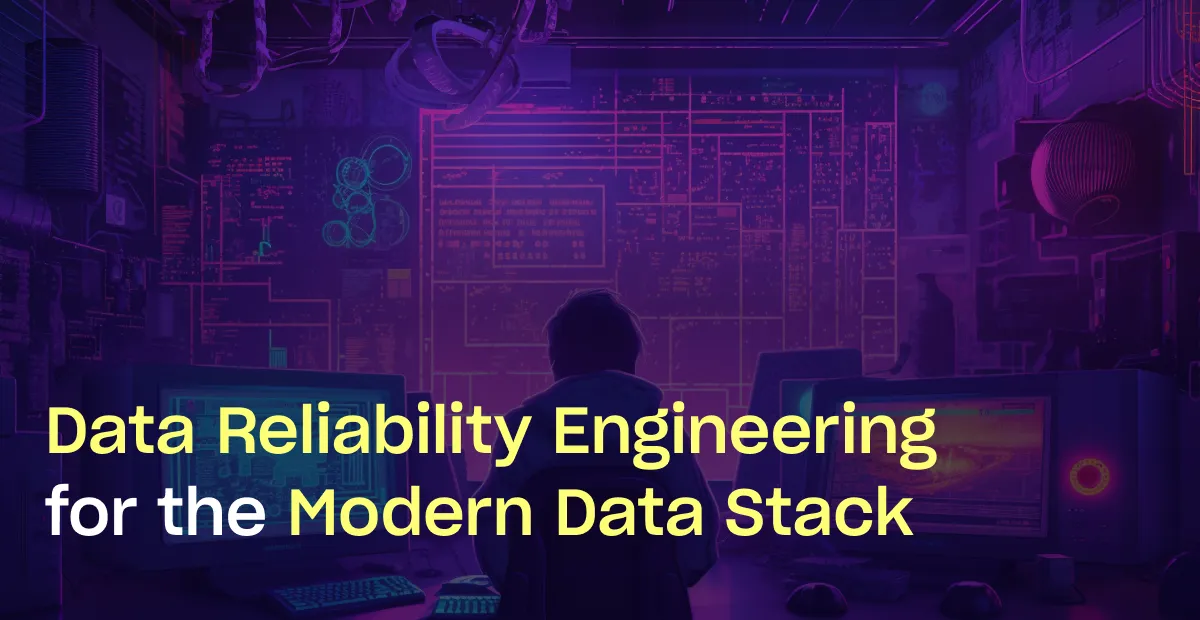
As data becomes more integral to business operations, it's increasingly important to ensure that the data being used is accurate, reliable, and high-quality. This is where the emerging field of data reliability engineering comes in. To help us understand, let's say that you're Head of Data at Wordle Industries, the international conglomerate expanding Wordle into international markets and with 3 and 4 letters, and you're dealing with an `activated_users` table used to send marketing e-mails.
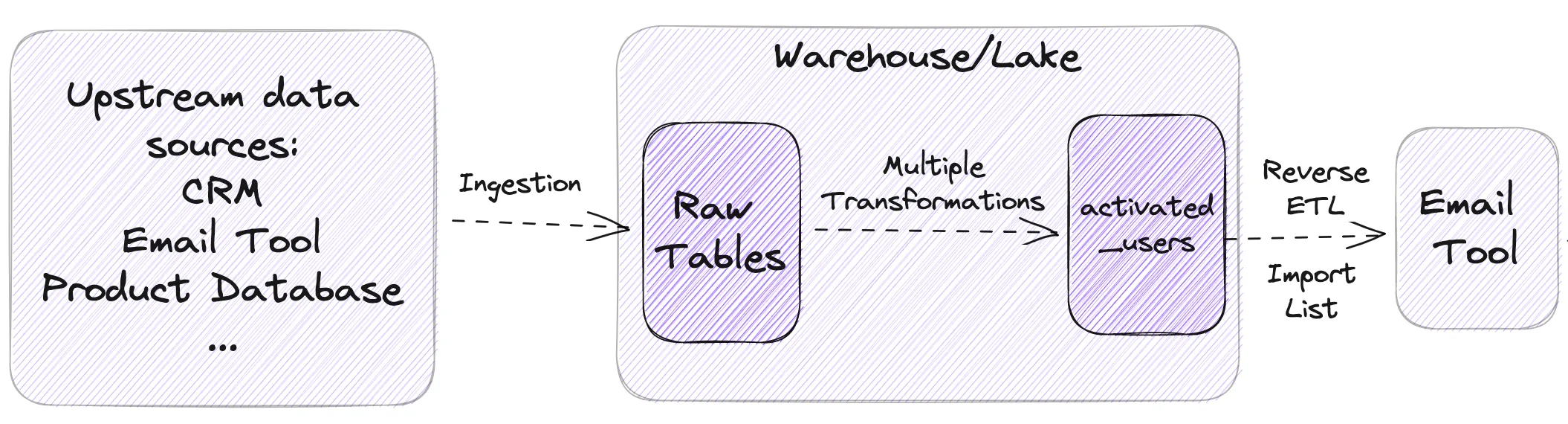
What is Data Reliability Engineering?
Data reliability engineering is the intersection of data engineering and site reliability engineering, with a focus on ensuring data quality and reliability instead of infrastructure reliability. It's a relatively new field that's driven by the increasing importance of data to businesses, as well as the increasing complexity of the modern data stack. Data reliability engineers work to ensure that data is accurate, reliable, and high-quality, and that it meets the needs of the organization. They also work to ensure that data is available to those who need it, and that it's protected from unauthorized access.
Data reliability engineering differs from other roles in the data sphere, such as data quality engineering, data architect, and data engineering. Data quality engineering focuses on ensuring the quality of data, while data architect designs and manages the structure of data. Data engineering focuses on the technical aspects of data management, such as ingestion, storage, and retrieval. Data reliability engineering focuses on ensuring data quality throughout the entire data stack.
Best Practices for Data Reliability Engineering
To ensure data quality throughout the entire data stack, data reliability engineers should follow best practices such as monitoring, alerting, testing, and documentation. Monitoring involves setting up systems to detect when data is inaccurate or unreliable, while alerting involves notifying the appropriate parties when issues are detected. Testing involves using automated tests to ensure that data is accurate and reliable, while documentation involves keeping documentation up to date so that others can understand how the data is being used and maintained.
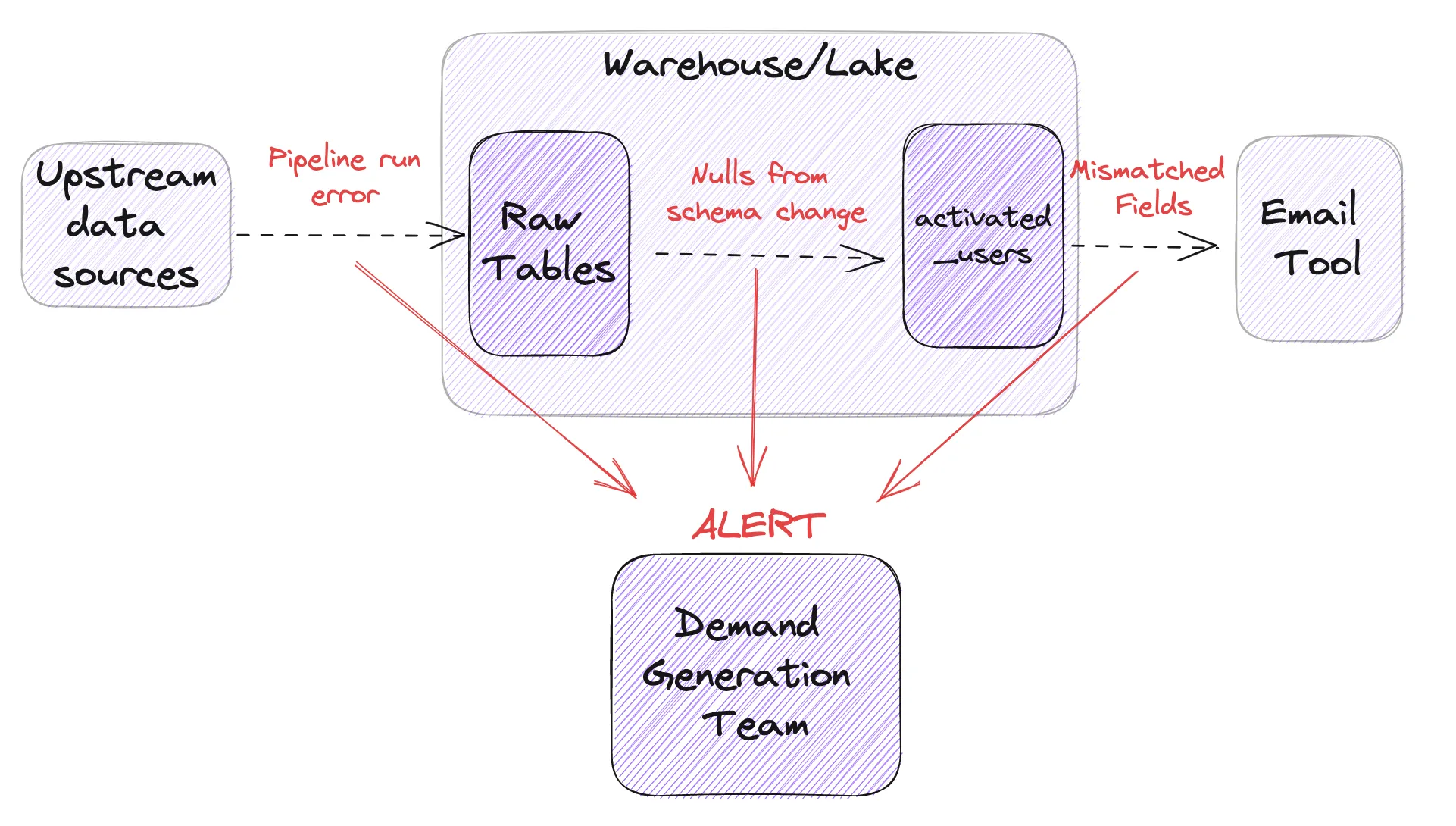
These best practices can be implemented in the modern data stack through the use of tools such as Metaplane, which provides monitoring and troubleshooting tools to ensure data quality throughout the entire stack. The Head of Data at Wordle Industries uses Metaplane to monitor the `activated_users` table, ensuring that it's always up to date and accurate.
Benefits of Data Reliability Engineering
The benefits of data reliability engineering are numerous. By ensuring data quality and reliability, data reliability engineering can lead to improved decision-making, increased trust in data, and faster time to resolution for data issues. For example, sales leaders at Wordle Industries make hiring decisions based on revenue data, which is made possible by the accuracy of the data provided by data reliability engineering.
Challenges and Pitfalls of Data Reliability Engineering
Despite its benefits, data reliability engineering is not without its challenges and pitfalls. One major challenge is maintaining consistency across the entire data stack, particularly when dealing with multiple systems and different data types. Another challenge is managing costs and resources, particularly when dealing with large amounts of data. However, these challenges can be mitigated through the use of tools and best practices, such as those discussed in section 3.
Summary
Data reliability engineering is a crucial aspect of ensuring the accuracy, reliability, and high-quality of data. By following best practices such as monitoring, alerting, testing, and documentation, data reliability engineers can ensure that data is accurate, reliable, and high-quality throughout the entire data stack. Metaplane can help data teams implement best practices and ensure data quality throughout the entire data stack, and is designed to be user-friendly and effective for the modern data stack.
Table of contents
Tags
...

...

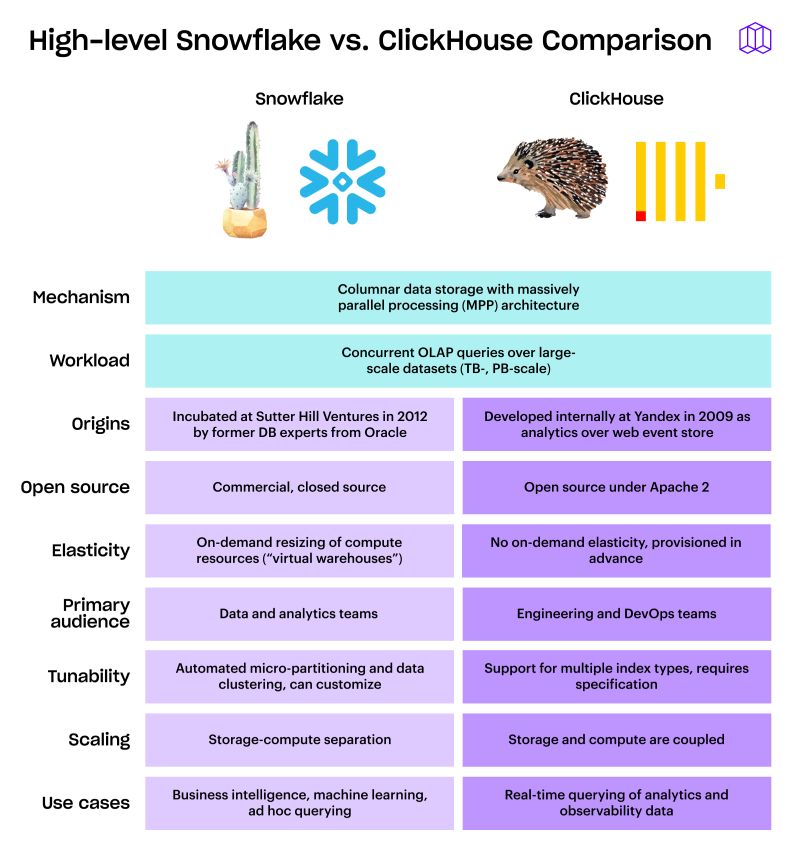


