Mistakes to avoid when implementing data observability software
Use our guide to understand where and how to make the highest impact with your brand new data observability tool so that you can accelerate trust in your data.
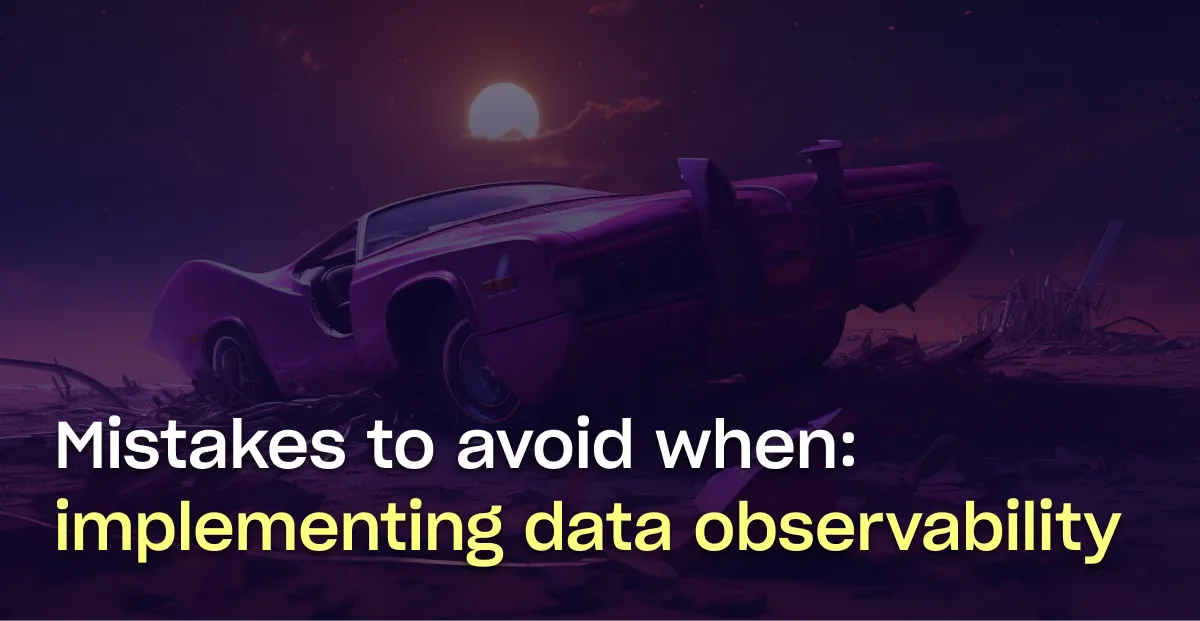
How To Implement Data Observability Software Properly
Let’s take a look at two scenarios. In both of the scenarios below, the company is using Metaplane for data observability, which means that they have access to our freshness and row count monitors. Freshness and row count monitors can inuit whether data is stale on a given table and whether the correct volume(s) were updated.
- Scenario A: ACME Company puts the freshness and row count monitoring on tables with “raw data” (i.e. non-aggregated data normalized into a tabular format from an API endpoint)
- Scenario B: BCME Company places freshness and row count monitoring on materialized views created by dbt model(s)
Who did it right?
Ignoring the fact that there’s a severe lack of information, both situations could be the right approach for either company, but the opposite could be true as well. Monitors are often a good place to start with thinking about implementing data observability, because it’s often the first use case that users begin with: “How will I get alerted the next time <type of data quality issue> happens?”
That type of reasoning leads directly to questioning what types of data quality metrics are available for data quality monitoring and where to place those monitors, but although anomaly detection IS a core piece of data observability, focusing only on monitor placement is the first pitfall during implementation.
A Brief Introduction Into Data Observability Tools
Data observability is a rapidly growing space with most vendors (including Metaplane) being relatively new to the data field. As a result, you’ll notice that different vendors have different capabilities, but regardless of which solution you implement, the first step always begins with outlining all relevant features, so that you can begin to plan for your implementation process.
Using Metaplane as an example, integrating your data warehouse, database, or data lake to find issues is a given, but after that, in our user onboarding you’ll see a mention to integrate Slack as well. Discovering a real incident and then letting it sit for hours could result in exponential magnification of negative impacts - which is why we recommend integrating a notification service such as Slack or Microsoft teams, so that you can act expediently on new issues. Of course, beyond Notification/Collaboration tools and your warehouse, we also recommend integrating the rest of your data stack to provide context for root cause analysis and uncover downstream impact.
We’ll continue using Metaplane’s capabilities as an example as we cover 5 common mistakes when planning for implementation.
Common Mistakes To Avoid When Implementing
Now, in our theoretical situation, you’ve already integrated your data stack to Metaplane, but haven’t started actively using it yet. Recall the earlier situation where you had a recent data quality issue in mind that you’d like to be able to capture next time - and now expand your recall a bit to include an even longer lookback period and being listing the
There’s a lot to take advantage of with your new data observability platform - beyond finding the issues that you are aware of, you can discover others that were previously unknown, use context to prevent mistakenly creating future issues, and even enable others outside the team on data.
1. Lack of Clear Objectives
Your company’s finance policy requires you to answer “How do we know your implementation was successful?” while requesting budget. The first, natural thought that comes to your mind is “If we catch a data quality issue.” But then you think about your morning commute - more specifically, waving goodbye to your family through the front door camera. You installed that device last week and haven’t caught any burglars, but have definitely slept better as a result because you know it’s pointed at the statistically most likely place your house is broken in from.
Drawing parallels from your security camera implementation, it can be helpful to break down your objectives in a similar way. You can think about installation (i.e. creating an account and integration) as your first step, and then think about where you’d want to place data quality monitors based on which objects are the most critical to your organization. If you want to artificially create an “incident” through data manipulation, you can add a column that’d trigger a schema change alert, which also has the added benefit of confirming “installation”. Continue breaking down the rest of your objectives in this way.
The last note here is to be cognizant of your timeline - you’ll want to ensure that any stakeholders have time to acclimate to their roles during the implementation period.
2. Neglecting Data Quality Issues
Begin with what you know but don’t constrain your thinking as a result of it. While it is important to understand how you’ll be able to capture “that” issue next time it occurs, it’s also important to remember how you got there. There’s a good chance that you already have unit testing set up - but maybe you weren’t testing for that particular type of issue, the test frequency had too large of a gap, or you simply didn’t have unit testing enabled for a particular table.
All of those considerations are meant to help with expand your scope beyond “find X issue on Y table”. In the hypothetical scenarios earlier, we talked about where to implement freshness and row count monitors. Many companies will choose Scenario A to implement freshness and row count monitors on “landing zones” (e.g. ingestion zones, bronze schemas) as they’re usually early indicators of data quality issues, and can often discover silent data bugs.
3. Overlooking Scalability Requirements
One of the downsides of simple setup is that eager users may choose to monitor for every single type of anomaly (20+ with Metaplane!) on every single table in the warehouse. While Metaplane does support automatically adding freshness and row count monitors for every object, we don’t recommend this for larger organizations with hundreds or thousands of tables, due to alert fatigue - It can be hard to cut through the noise. While you could find every potential data quality issue in your warehouse, an important part of implementation is being rigorous about what alerts count as incidents when considering the object in question.
Over time, you’ll want to be stringent about re-evaluating your monitor types and placement, both to ensure that new objects have coverage, but also to evaluate whether your old monitor placements are still relevant.
As a bonus, while evaluating your old monitor placements, consider how they’re sampling data - if it’s directly querying tables as a necessary step to sample values within a column, your warehouse compute will also be affected.
4. Ignoring User Training and Adoption
One often overlooked consideration is user involvement. Beyond familiarizing people with navigating the UI, users, even those that sit outside the data team, should be involved with improving data quality. The common ground between all teams is usually found at a place like Slack or MIcrosoft Teams.
As a result, in addition to some training on platform usage for data team members, it’s also useful to create a clear notification channel strategy that also incorporates business users. This time of training can include: what slack channels receive alerts, what types of alerts are sent (e.g. freshness, schema change), and roles of people involved in the slack channel.
5. Neglecting Continuous Monitoring and Optimization
By default, your data quality monitors created through Metaplane will run at the frequencies that you specify in the platform, enabling continuous monitoring by default. While our machine learning models lead the industry in anomaly detection, no machine is at a point where it understands your business as well as you do. As a result, especially when a monitor is being setup for the first time (i.e. early after it’s finished its training period) or when you’ve changed how your data behaves or looks, it’s important to provide feedback to the model.
That feedback can be given to Metaplane’s machine learning models either through slack or the app itself by marketing particular data points as normal to inform the acceptable range.
Implementation Tips & Best Practices To Follow
A successful implementation of a data observability platform like Metaplane could be summed up with “we caught a revenue impacting incident on the first day.” But that’d be discrediting all of the work that you’ve done to get it to catch critical incidents.
Instead, following these implementation best practices can not only help you to capture those p0 incidents, but also help you outline your work:
- Outline capabilities
- Integrate your entire data stack
- Create clear objectives with a focus on critical objects and not root causes yet)
- Review rollout
- Review again as you continue to succeed and scale
Get started with Metaplane today!
Does data integrity matter to your organization? Does it matter to you? If you answered “yes” to both questions, then you should create a free Metaplane account. After setting up credentials for your Metpalnae account, use our documentation to integrate your data warehouse and/or notification tools like Slack. If you’re just looking to forecast what your experience would be like, pick one or a handful of the tables that have importance to your organization, let the machine learning models train, and then create some artificial errant values.
Have fun, and reach out to brandon@metaplane.dev or contact the broader team if you have any questions!
Table of contents
Tags
...

...

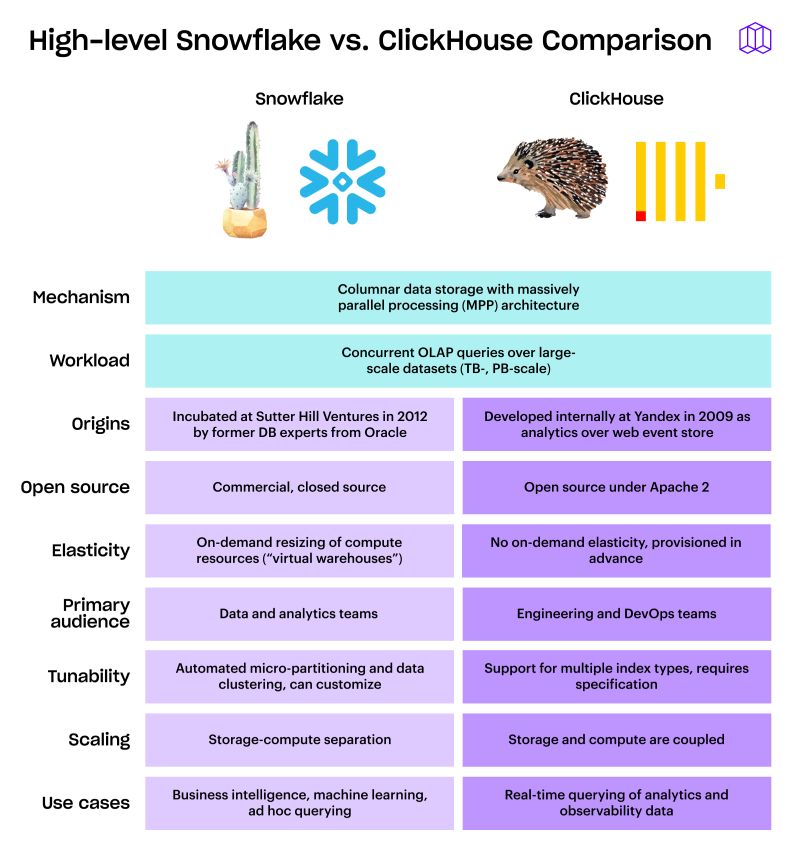


