The Future of Business Intelligence: 5 Transformative Shifts in the Data Landscape
What if you could answer any question about your business with a few clicks? What if you could identify opportunities and risks before they become critical? What if you could collaborate with your team in real-time without worrying about data quality issues? These possibilities are the future of business intelligence, and they are already happening right now.

1. From Monolithic to Modular: The Rise of the Modern Data Stack
The modern data stack is a set of best-of-breed tools for collecting, storing, processing, and visualizing data, integrated via APIs and connectors in a modular fashion. To help us understand, let's say that you're the VP of Data at Rainforest, an e-commerce company that sells hydroponic aquariums to high-end restaurants. You use Fivetran for ELT, Snowflake for warehousing, dbt for transformation, and Looker for BI. You have a daily_revenue table that the VP of Sales uses to make decisions.
The legacy stack (e.g., Oracle, SAP, IBM) was monolithic, expensive, and slow to adapt to new data sources and formats. It was also prone to errors, silos, and biases, since data quality and lineage were hard to track and audit.
The new stack enables faster innovation, higher flexibility, and greater scalability, since each component can be swapped or upgraded independently. It also allows for more transparency, collaboration, and democratization, since data ownership and responsibility can be shared among different roles and functions.
2. From Static to Dynamic: The Rise of Data Observability
Data observability is the practice of monitoring, troubleshooting, and improving the quality and performance of data pipelines and workflows, using automated tools and metrics. You start to notice that the daily_revenue table has some discrepancies, and you suspect that they are caused by a change in the ETL pipeline. You use a data observability tool like Metaplane to inspect the pipeline and identify the root cause.
Data observability is crucial for the modern data stack since it helps to ensure data trust, reliability, and agility. It minimizes the risks of bad data, data downtime, and data breaches, and maximizes the benefits of data insights, data products, and data collaborations.
Data observability consists of three main components: data monitoring, data tracing, and data profiling. Each component provides different perspectives on data quality and performance and can be customized to fit different needs and contexts.
3. From BI to AI: The Rise of Embedded Analytics
Embedded analytics is the practice of integrating analytical capabilities and workflows into business applications, portals, and processes, to provide context-sensitive and actionable insights to users. You realize the user_info table creates user context, such as company industry, from a 3rd party source that you provide customer emails to. This can be used to generate new features for a machine learning model that predicts the demand for each product category. You embed the ML model into the Rainforest website and use it to recommend personalized products to each customer.

Embedded analytics enhances the user experience, reduces the user's cognitive load, and increases the user's productivity by providing relevant and actionable insights without leaving the context of the task. It also improves the data quality and availability since it encourages users to contribute feedback and corrections.
Embedded analytics requires careful planning, design, and testing to ensure that it aligns with the user's goals, preferences, and data needs. It also requires a solid foundation of data governance, security, and privacy to prevent misuse or abuse of data.
4. From Binary to Probabilistic: The Rise of Decision Intelligence

Decision intelligence is the practice of using data, algorithms, and human judgment to make better decisions by balancing accuracy, complexity, and uncertainty. You and the Head of Data at Rainforest use Sigma for BI and Hightouch for Reverse ETL. You notice that the activated_users table has some outliers, and you wonder whether they are caused by bots or humans. You use a decision intelligence tool to classify the users based on their behavioral patterns and adjust the marketing strategy accordingly.
Decision intelligence enables smarter, faster, and more ethical decisions by leveraging the strengths of both AI and HI (human intelligence). It also fosters a culture of experimentation, learning, and growth by encouraging constant feedback and iteration.
However, decision intelligence also poses challenges. Successful implementation of decision intelligence requires a deep understanding of the domain, the data, and the stakeholders, as well as a clear definition of the problem, the metrics, and the tradeoffs. Decision intelligence also requires a high level of transparency, accountability, and communication to ensure that the decisions are explainable, justifiable, and accepted.
The benefits of decision intelligence cannot be overstated. Decision intelligence enables strategic advantages, better results, and even competitive edge. Decision intelligence allows organizations to approach challenges more thoughtfully, iteratively, and dynamically. By leveraging data and human intelligence together, organizations can move beyond binary approaches to make smarter, more informed decisions.
5. From Reactive to Proactive: The Rise of Collaborative Intelligence
Collaborative intelligence is the practice of sharing and combining data, insights, and actions across teams, functions, and organizations to achieve common goals and solve common problems. Sharing the results of the ML model with the chief revenue officer (CRO) at Rainforest, who is responsible for the customer acquisition strategy, allows the CRO to see how the ML model can be applied to drive customer acquisition. The CRO shares the revenue forecast with the CFO, who is responsible for the budget allocation. The CFO shares the investment plan with the CEO, who is responsible for the company's vision and mission.
Collaborative intelligence enhances the collective intelligence, creativity, and resilience of the organization, by leveraging the diversity, expertise, and resources of all its members. It also fosters a sense of ownership, accountability, and belonging, by aligning the individual and the organizational goals.
However, successful implementation of collaborative intelligence requires a strong culture of trust, respect, and empathy, as well as a clear structure of roles, responsibilities, and incentives, to prevent conflicts, biases, and miscommunications. Collaborative intelligence also requires a high degree of data literacy, data ethics, and data governance, to ensure that the shared data is accurate, relevant, and secure.
Summary
In conclusion, the future of business intelligence is rapidly evolving. The modern data stack is changing the way we think about data collection, processing, and analysis, and empowering users at all levels to access and explore data on-demand in a self-service manner. Data observability is crucial for ensuring trust, reliability, and agility, and enables data teams to get the most from their data stack. Additionally, embedded analytics and decision intelligence are transforming the way we use data to make decisions, and collaborative intelligence is allowing organizations to combine the strengths of multiple teams and stakeholders to achieve common goals.
At Metaplane, we understand the challenges and opportunities of the modern data stack, and we are committed to helping data teams achieve their full potential. Our data observability platform is optimized for the modern data stack and designed to help data teams overcome the challenges in data quality. Get started in minutes for free, or book a demo to learn more about how we can help ensure trust in your data.
Table of contents
Tags
...

...

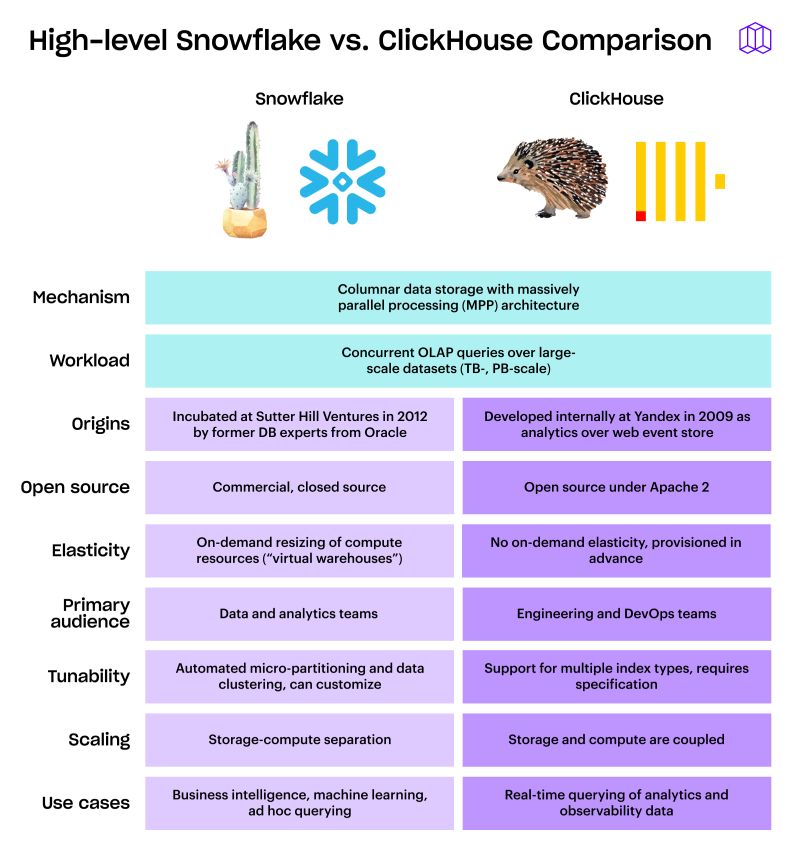


