What are Data Products?
As the use of data has evolved, so has its importance to the business, creating the term "data as a product" or "data products". Learn what that means for you and your team in the post below!
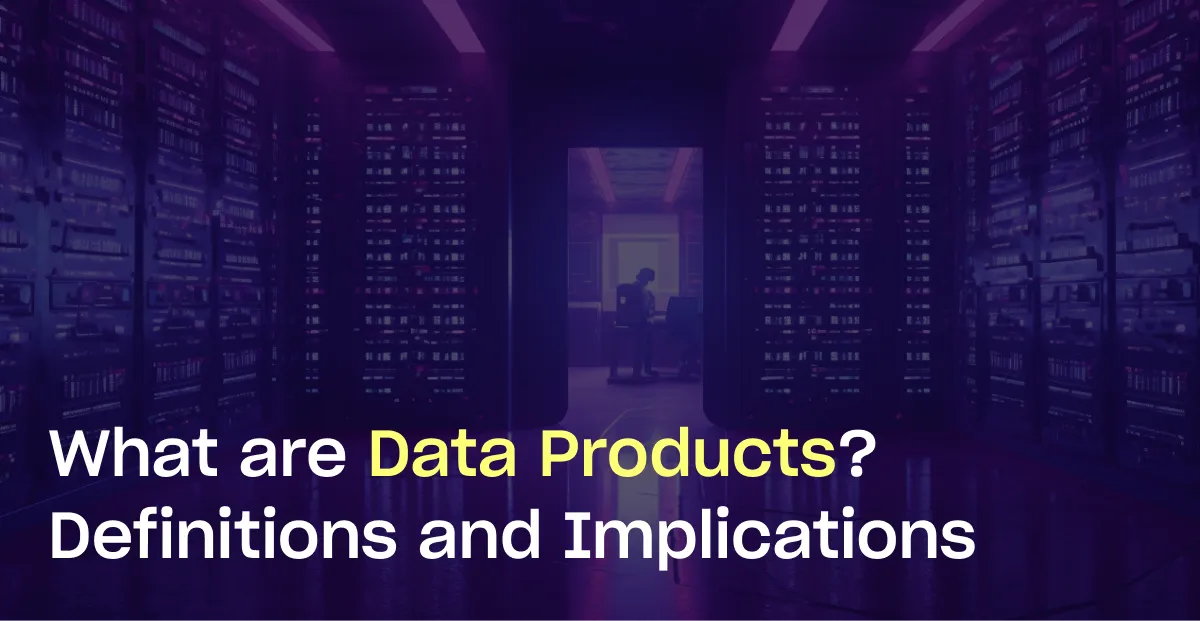
What are Data Products?
On the surface, the simple answer is “the use of data for decision making or problem solving”. That answer, however, leaves us looking for context, tangible examples, or implications that the post exists to address.
Data's Role in Our Lives
Even on an individual scale, we interact with data every day, albeit in small quantities. We rely on data to make decisions, whether it's choosing where to cross a road or deciding what food to eat. These choices contribute to our internal data storage, enabling us to optimize our routines, rank preferences, and ultimately make better decisions. We have all experienced the benefits of data-driven decision-making, and businesses, comprised of multiple individuals with a need to measure and report results, have reaped these advantages as well. This leads us to the most prominent application of data in businesses today: analytics.
The First Data Product: Analytics
Reports and dashboards have long been essential to data teams. With accurate data, decisions related to revenue can yield significantly higher results compared to relying on gut feel alone. Data teams, including data engineers, data scientists, and data analysts, are frequently tasked with building reports and dashboards to address common questions and enable data-driven decision-making; an example of this would be product analytics to decide upcoming roadmap work.

These are common questions either you or others have asked and tasked data teams with building out. You’ll likely find that these questions will come up again and again, increasing the importance of these reports and dashboards created to address them, and creating necessary maintenance work down the line. Much like any other product, updates are needed to keep pace with the market, potentially including:
- Adding or replacing data sources, such as new business applications
- Creating filters for drilldowns that stakeholders are interested in
- Deprecating or updating aspects of your dashboards to reflect the changing nature of questions
Moving Beyond Analytics: Expanding Possibilities with Data Engineering
Advancements in data engineering have propelled data products beyond traditional analytics, and changed the landscape of what data platforms look like. Technologies such as Hadoop file storage systems and scalable storage and compute in cloud warehouses enable the storage and processing of vast datasets, leading more and more data sources to be included as part of the larger data strategy. The rise of data lakes, coupled with frameworks like Spark, Beam, and Flink, has further expanded the use cases, encouraging "automated data decision making", and the proliferation of data science initiatives.
Automated Data Decision Making: Internal vs. End-Customer Facing Use Cases
Automated data decision making can be categorized into two main use cases with different targets: internal-facing and end-user facing. Internal-facing use cases, also known as operational analytics, involve transforming data, including cleaning and internal calculations for new metrics, and feeding it directly into business applications without manual intervention. This streamlines existing stakeholder workflows and enhances ease of use.In these scenarios, data quality must be extremely high, as there are less manual checkpoints in the lifecycle of the data flows prior to reaching downstream data consumers. The data architecture, including data pipelines and expanding beyond the data warehouse, needs to be monitored closely for smooth delivery.
Examples of Data Products
Internal Facing: A marketing dashboard monitoring campaign spend or a simple report matching sales reps to quota attainments can qualify.
External facing products: One example of this would be using user interactions with your software, combined with marketing materials they might have read, as proxies for their engagement with your overall brand, combined with values assigned to each action, to create a scoring system for how likely they are to renew their contract for next year.
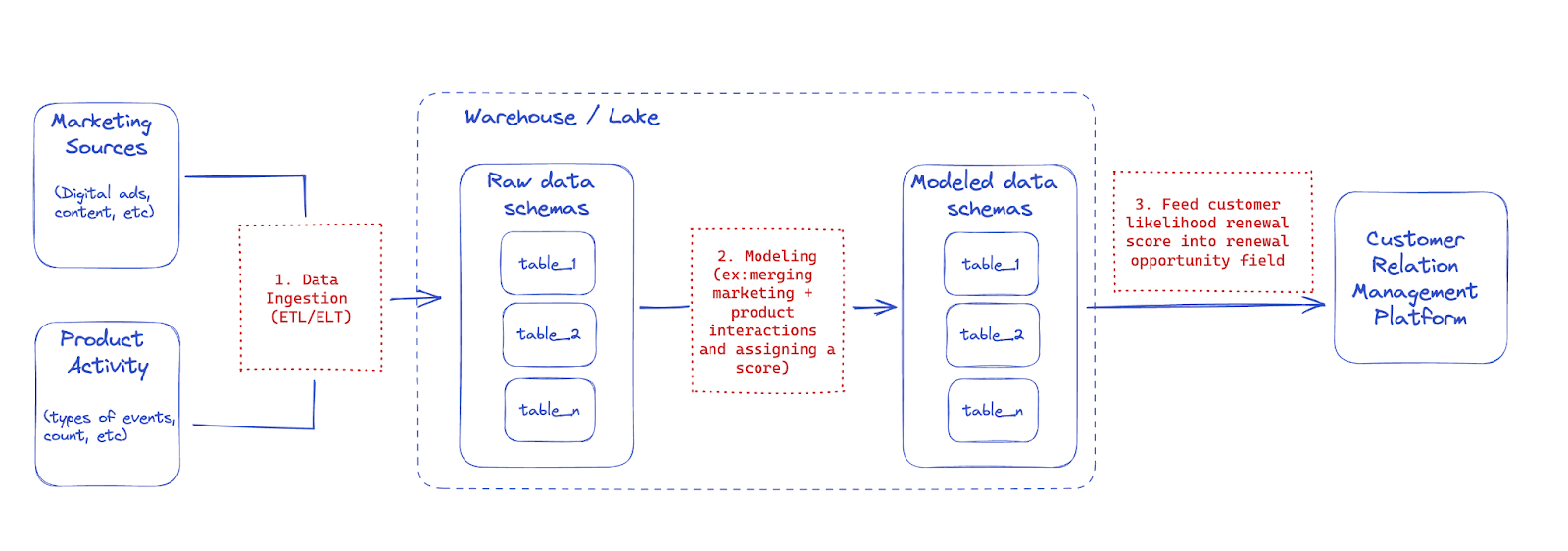
The Expanding Definition of Data Products and the Rise of Data Product Management
The definition of "data product" continues to expand rapidly. The remarkable rise of machine learning tools such as ChatGPT, owned by OpenAI and supported by Microsoft, exemplifies how major companies recognize the potential of products leveraging data. Companies today are employing more and more data scientists in a race to develop new algorithms and data models taking advantage of the massive power of some of these machine learning and AI APIs. As technology advances and new opportunities arise, the scope of data products will inevitably grow.
As data products grow both in scope and volume, we’ll begin to see data product managers as a career field grow too, handling the product lifecycles with the traditional product thinking we see applied to traditional products.
Implications of Data Products
When launching a product, various steps must be taken, including market research, user testing, supporting go-to-market efforts, and incorporating feedback and market changes into future iterations. Those experienced in product development understand the intricacies and investments involved. Therefore, it is exciting to see the term "data product" gain prominence. Promoting data to the level of a product offers several benefits:
Market Research
Formalized research with stakeholders enables the creation of a highly useful "product" that goes beyond initial requirements, solving a broader range of use cases.
User Acceptance Testing
Both data teams and stakeholders share responsibility for the success of a product created to meet customer needs. User acceptance testing becomes crucial in ensuring alignment and effectiveness.
Go-to-Market Strategy
Data products represent a substantial investment, signaling an internal commitment by a company to drive adoption. This shifts the paradigm from neglected dashboards with minimal usage to actively promoting data products to prevent wasted investments.
Iterative Updates
Similar to ad-hoc reports addressing new questions, existing dashboards often become neglected. Treating data products as products emphasizes the need for regular review and improvement, ensuring their continued usefulness to the business.
Conclusion
Data products play a crucial role in modern businesses, enabling data-driven decision-making and problem-solving. By incorporating data engineering and product management principles, organizations can unlock the full potential of their datasets. As the definition of data products expands and we see new concepts (e.g. data mesh) gain prominence, it is essential for businesses to adapt and embrace these trends to remain competitive in the data-driven landscape.
Table of contents
Tags
...

...

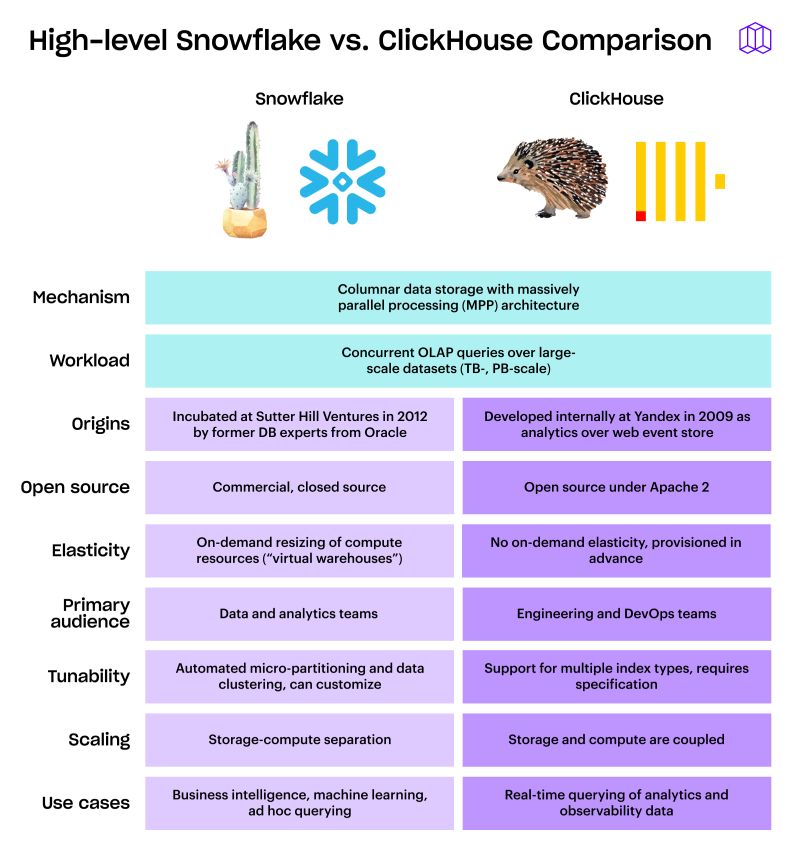


