A Framework to Understand How Poor Data Quality Hurts Business Performance
Low-quality data is a perennial topic, but more relevant than ever in 2023. Lapses in data quality costs organizations an average of $13 million per year, and its impact varies depending on its use in different industries. Therefore, it is crucial for data leaders to ensure data quality by understanding its role in their specific business context, setting up data management and governance practices, and using the right tools to prevent and troubleshoot data quality issues before they affect business performance.

The specific cost of data quality problems varies from business to business and vertical to vertical. But, on average, low-quality data costs organizations around $13 million a year (Gartner, 2021).
That’s a number that should make data leaders (and the C-suite leaders they support) sit up and take notice.
While the negative impacts of poor data quality can unite data leaders across verticals, the cause of those issues is as unique as each product or service that the data team supports.
As Tolstoy said, “Every happy family is the same, but every unhappy family is unhappy in its own way.” I think about data quality the same way. Every negative business outcome is negative in its own way.
As a data leader, it’s your responsibility to contextualize data quality in a way that makes sense to business stakeholders within the scope of your business’ KPIs and goals. After all, with great power comes great responsibility, and that includes making sure that low-quality data doesn’t hurt the reputation and bottom line of your business.

Two things to consider when thinking about the impact of your data’s quality
Aside from that big scary number up top, how should you think about the way data quality impacts your business? The answer depends on two things:
- The quality of the data itself: While perfect data doesn’t exist in the real world, you should be able to identify what “good enough” data looks like for your company.
- How the organization uses data overall: There’s no single “right way” to use data. Rather, you should be creating data management and data governance best practices that make sense for your unique use cases.
For example, incorrect customer data in a B2B SaaS company could lead to mistakes like sending someone an irrelevant product recommendation at the wrong time. This hurts your reputation for being customer-centric and can cost you revenue in the long run. Incorrect customer data for companies in the healthcare industry, however, could lead to prescribing a drug that triggers a fatal reaction, seriously harming the patient and sparking a lawsuit as well as a flurry of bad publicity.
From this example, we can see that poor data quality in one context (B2B) is important, but not fatal. In other words, there’s more margin for error. Data teams working with data in an industry regulatory compliance, on the other hand, need considerably more strict data quality guardrails. This is to say: Not all data quality consequences are equal. Context matters.
Below, I’ll explain:
- The four main ways companies use data
- The three-part framework you can use to identify how data quality impacts business performance in your organization
- How to prevent and troubleshoot data quality issues (before they impact your business)
The four main ways companies use data
Most companies use data in one of four ways: ignoring it, using it for operations, using it to inform strategy, or selling it as a product.
With the exception of ignoring it (there’s no “good” way to NOT use data), data quality impacts all of these approaches. High-quality data is a competitive edge, while poor quality data is a hindrance at best. Let’s take a closer look at what each of these data uses entails:
- Not using data at all. This is surprisingly common despite the hype around “data-driven” organizations (and the competitive advantages afforded by high-quality data). There isn’t a good way to not use data at all, so this is an outlier on the list.
- Using data for operational purposes. This includes internal operations like allocating ad spend and external operations like customer communications. High-quality data helps your marketing team target people most likely to buy, helps your logistics team move product efficiently, and helps your customer success team provide the personalized service that creates raving fans. Applied well, high-quality data can maximize revenue while minimizing costs.
- Using data to influence internal product decisions and market strategies. The competitor with the best data gets the first crack at opportunities. With reliable, high-quality data, product teams can track trends and be the first to develop features customers want. They’ll also have foresight when the winds are changing and it’s time to change course. Good data helps your company make market moves when they’re most advantageous.
- Using and selling data as the product. If you are in the business of selling third-party data, the importance of data quality is obvious. A clean, secure, reliable product improves customer satisfaction and retention, reduces legal liability, and keeps you on the right side of regulatory authorities.
When you boil it down, every business in every vertical does three things: spend money, make money, and take risks. The moment you tether data to one of these activities, the quality of that data becomes paramount.
If your business uses data for anything, data quality impacts business performance. The only question is how.
A three-part framework to identify how data quality impacts your business
It’s easy to see how poor data quality impacts other companies.
In 2021, problems with Zillow’s machine learning algorithm led to more than $300 million in losses. About a year earlier, limitations on table rows caused Public Health England to underreport 16,000 COVID-19 infections. And of course, there’s the classic cautionary tale of the Mars Climate Orbiter, a $125 million-dollar spacecraft lost in space because of a discrepancy between metric and imperial measurement units.
It can feel more challenging to see how poor data quality can impact your own company (especially if you’re trying to improve data quality before there’s a big public issue with it). I find it helpful to think about data quality using a three-part framework:
- You don’t use data at all, so data quality doesn’t matter. There are two kinds of companies at this level of the framework - those who don’t use data and those who don’t use data (yet). Businesses in the first camp are rapidly disappearing. Those in the second have at least one thing going for them: You can bake data quality assurances into your business data strategy from the very beginning.
- You use data to fuel business decisions, which means the cost of bad data quality is the cost of a bad decision. For example, low-quality market data could lead you to open new locations in a region where the median income isn’t high enough to support them.
- You use data to inform business operations, which means the cost of incorrect data is the cost of time inefficiencies and money against your bottom line. For example, incomplete customer data leads your marketing department to target the wrong buyers, spending the advertising budget on a campaign that fails to convert.

It’s worth noting not all downsides have dollar signs attached. Low-quality customer data also costs you in reputation and relationships. Nearly half of people who responded to a ParcelLab study said they're frustrated when brands’ poor data quality results in recommendations for products they’ve already bought, and almost a quarter said they would never buy again from a brand that sent them irrelevant messages.
By focusing on data quality initiatives across the organization, from data profiling to data cleansing, you reduce the risk of bad decisions in business processes, which helps you make smarter strategic calls, delight customers, and gives stakeholders more confidence in data-based decisions.
So how do you move the needle when it comes to data quality? Let’s take a look.
How to prevent and troubleshoot data quality issues before they impact your business
To prevent and troubleshoot data quality issues before they impact the business, you need to dig down and figure out the why behind data issues. By identifying and troubleshooting the root cause of data problems (I know, easier said than done), you prevent the cascade of downstream data errors.
Let’s say an e-commerce company uses data for day-to-day operations, and the data is delayed. Data that should be readily available in almost real-time is now taking taking five hours to fetch. This means customers aren’t getting order confirmations in a timely manner, which, in turn, results in more support calls to your customer service team and longer wait times for everyone overall.
Unfortunately, it’s not enough to find the issue after customers are already annoyed. Mitigation of this problem needs to have happened before the business and customers felt the impact.
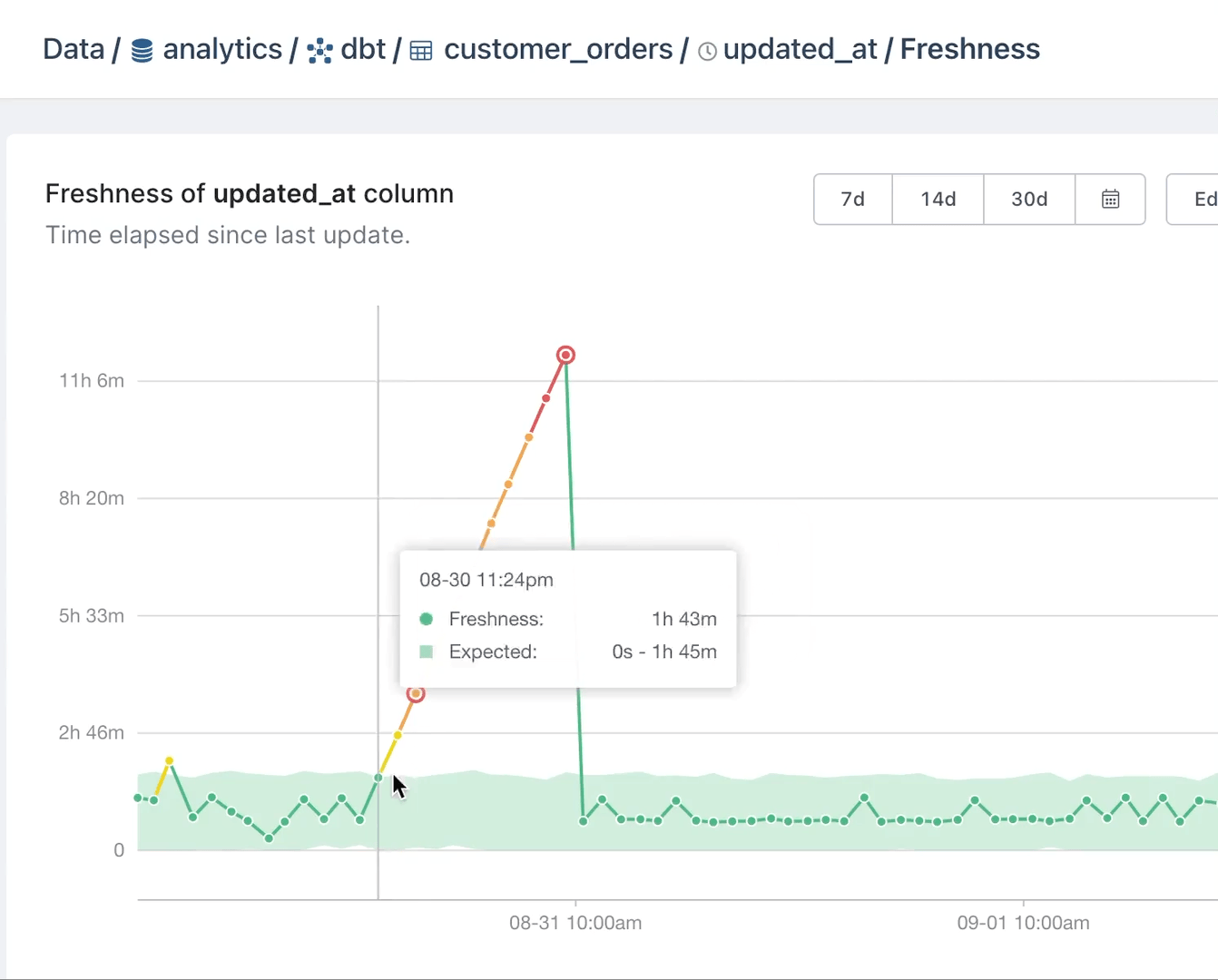
Enter data observability tooling. When your data observability tool alerts you that data coming through Fivetran is delayed, you can take action to correct the problem before the effects snowball. Or when the uniqueness of data values in a primary key column is compromised, you can kick off a deduplication process.
So, what do you do once your observability tool alerts you to an issue that's impacting business intelligence dashboards used for decision-making (and gives you insights into what the root cause of the issue is)?
Unfortunately, unlike software engineering, you can’t just shut off your pipelines to fix an issue and turn them back on again (at least, not without some serious data downtime and data loss). Data has weight and history.
Instead, data stewards need to balance keeping data online with the compounding nature of data quality issues by prioritizing data issues against two metrics:
- Time-to-detection: how long the problem exists (and potentially compounds) before anyone knows it’s there
- Time-to-resolution: how long it takes to fix the problem once it becomes apparent
Data quality management tools help you cut down on time-to-detection by alerting you earlier to data issues, and shortening time-to-resolution by giving you all the information you need to effectively troubleshoot problems.
With a data observability tool in your data stack, you can monitor all dimensions of data quality, like data accuracy, data consistency, data integrity, across your data sets from data sources to consumption. And, when issues arise, metadata analysis provides data teams a breadcrumb trail to follow to trace data issues to their source.
As the data team spends less and less time chasing down the effects of bad data, you and your team can invest in guardrails and business processes to prevent data issues moving forward (meaning you can prevent inaccurate data vs spend time reacting to it).
Table of contents
Tags
...

...

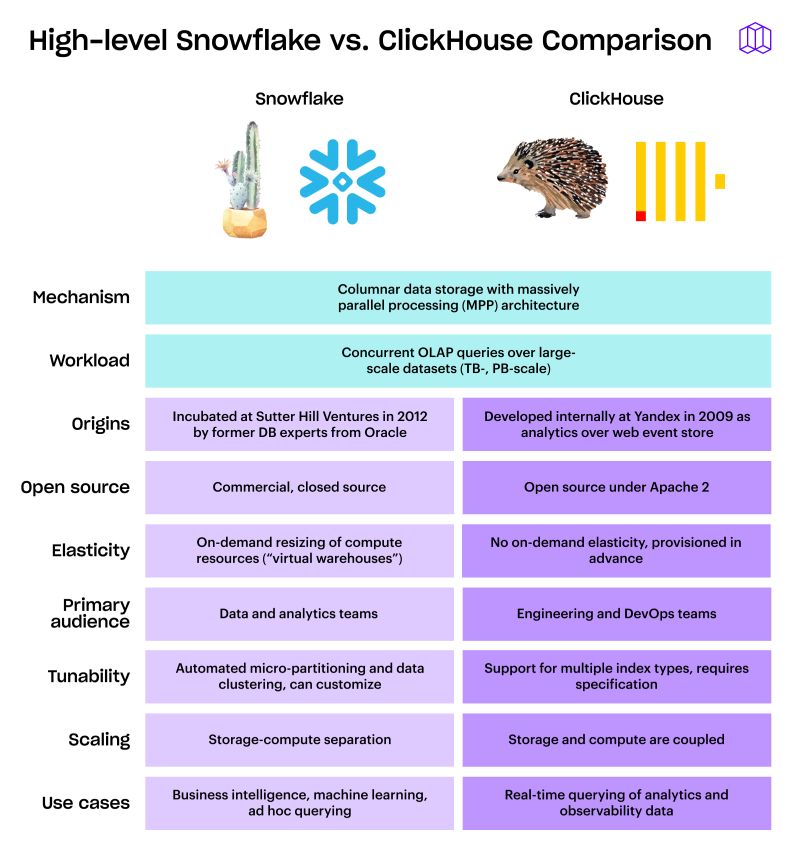


