5 Common Data Quality Challenges (and How to Solve Them)
.webp)
Especially in 2023, data teams are increasingly expected to deliver high-quality data their stakeholders can trust. With poor data quality, company leaders can’t make informed business decisions that ultimately improve financial outcomes.
Unfortunately, data quality challenges attack from all angles—spanning both machine and human errors. In this context, it’s imperative that data teams employ data quality management tools, strategies, and processes that empower them to deliver on their mandate of fixing data quality issues before they compound and preventing them where possible.
In this blog post, we break down the top five most common data quality challenges we see at data-driven companies today and offer potential solutions for mitigating them.
1. Software sprawl and data proliferation create more to manage
Over the past 10 years, there has been an explosion of technology. In fact, the average company now uses more than 200 different apps and collects data from over 400 data sources to support business processes. As the number of tools leveraged by companies increases, the data team’s responsibilities become more complex and harder to execute.
Making matters worse, data engineers must manage not only their own tools, such as Snowflake, dbt, and Looker, but also keep tabs on how downstream apps, like Salesforce, Marketo, and Gainsight, as well as downstream use cases like machine learning, evolve over time.
Data engineers are asked not only to prevent data quality issues, but also pull the threads from these disparate sources together into a tapestry of useful insight—a time-intensive and, arguably, dangerous pursuit. The thing is, each tool brings a greater risk of errors being introduced into the company’s data pipelines, and makes it harder for data teams to spot and stop data quality issues.
Ironically, the solution may be investing in more tools. Just as we lean on automation to help us collect company data, we can leverage data observability tools to help us detect, investigate, and solve data quality issues before they reach business users.
2. Data quality issues come from outside the data team
The data team may bear responsibility for the company’s data, but they’re rarely the ones who create it.
The reality is that most data is extracted from humans, who manually enter it into their company’s core systems. Consider, for example, the medical office receptionist who enters a patient’s insurance information during check-in. They probably don’t think of themselves as a data creator, but their choice to abbreviate California as “Calif.” instead of the standard “CA” compromises the company’s data quality. (That’s to say nothing of unintentional typos.). Not only does an inaccurate data record have impact on an individual, but in an industry like healthcare there is even a potential risk of a lapse in regulatory compliance.
Despite this, most data consumers don’t realize that their company’s data quality is determined long before it reaches the data team. The problem is a lack of data literacy—something that 62% of business leaders believe has a negative impact on the value they get from their data technology, according to a 2021 study by Experian.
To mitigate this data quality issue, we recommend that you:
- Create and enforce data entry standards across your organization. Upfront education about the consequences of low-quality data can help you reduce preventable data entry errors.
- Use data validation rules inside the tools your data creators use. For example, you could set up fields with a drop-down menu of approved selections instead of allowing open string fields. Validation at the source leads to greater data consistency and data accuracy throughout the data value chain, such as by flagging duplicates in a UI to preserve uniqueness of unique IDs.
- Establish a data governance initiative in collaboration with stakeholders to first identify data quality metrics that matter to your company, then codify those metrics as explicit data quality measures, and finally set data quality rules to programmatically catch appearances of bad data.
Both of these strategies will help your team achieve a higher standard of data quality.
3. Data engineers aren’t effective stewards of every individual number
Data creators and consumers aren’t the only ones who need education. Data engineers must learn a great deal about the data they serve up. Otherwise, they risk failing to deliver what business users expect.
Because data engineers aren’t the consumers of their company’s data, they rarely have the context needed to understand what each metric should look like, or which business rules should be applied. Given the scale and speed at which data moves through their pipelines, it simply isn’t feasible for them to keep up with every number on their own.
That’s why it’s important for data engineers to have regular conversations with data consumers, whether they are data science or data analytics or business teams. To improve data quality, data teams must first understand each metric they deliver to downstream business intelligence dashboards and reports, including why it matters. Only then can they ensure the right numbers appear.
4. Data teams don’t have the (meta)data they need to do their jobs effectively
Every team in an organization depends on quality data to do their jobs. Ironically, real-world data teams often operate in the dark—relying on assumptions to accomplish their goals. For example, data engineers often have gut feelings about which dashboards and reports are used most frequently, or which tables and columns these downstream assets depend on. And they prioritize their work based on these assumptions, despite lacking the data they need to inform their decisions-making processes
Metadata, or data about data, is the answer. Without it, data engineers must try to solve data quality problems without adequate direction. If you’ve ever sifted through a database in search of the source of a broken dashboard, and still come up empty handed, you know what we’re talking about. Data cleansing without context is a challenge. Metadata provides that crucial context, pointing you toward where you should look and what you should look for.
So, how do you access this metadata? One option is to dedicate a significant number of engineering hours to building and maintaining a custom-built, in-house solution. If you’re looking for an easier and faster solution, you could instead invest in an out-of-the-box data observability tool. The right one will capture all four pillars of data observability: it will describe the internal and external characteristics of data, trace lineage to describe data dependencies, and record how the data system interacts with the outside world. Only with these four pillars is it possible to track all relevant dimensions of data quality.
5. Companies struggle to recruit experienced data engineers
Despite no clear pathway for becoming a data engineer, the role is highly in-demand at organizations across the United States. In fact, the number of open data engineering jobs grows by 35% year over year, according to LinkedIn’s 2020 U.S. Emerging Jobs Report. As a result, hiring can be a slow and arduous process—leaving most companies short-handed.
The unfortunate consequence is that many data teams are doing triple duty: maintaining data infrastructure from ETL to transformation within data lakes and data warehouses to activation, responding to inbound requests to integrate new data sources and data sets, and putting out data quality fires. This leaves little time for higher-value projects that proactively address recurring problems.
As demand for quality data grows within your company, you can’t afford to rely on labor-intensive methods for completing your core responsibilities. While new tooling isn’t the key to solving your staffing woes, the right data stack can buy you back time. Data observability tools, for example, shorten the time it takes to detect, investigate, and resolve data quality issues by an average of eight hours per week.
Quell your data quality challenges with data observability
Data quality challenges result from both machine and human errors, and therefore must be addressed with a combination of people, processes, and technology. Once you've implemented data quality improvement policies and processes, you should consider investing in an end-to-end data observability platform.
With real-time anomaly detection, usage analytics, and lineage features, data observability tools enable you to detect, investigate, and resolve data quality issues before data consumers flag them—the first step toward improving stakeholder trust. They also provide the critical metadata you need to do your job, and free up the data team to work on higher-priority projects, such as building new models and reducing data debt.
Ready to get started? Sign up for Metaplane’s free-forever plan, or test our most advanced features with a 14-day free trial. Implementation takes under 30 minutes.
Table of contents
Tags
...

...

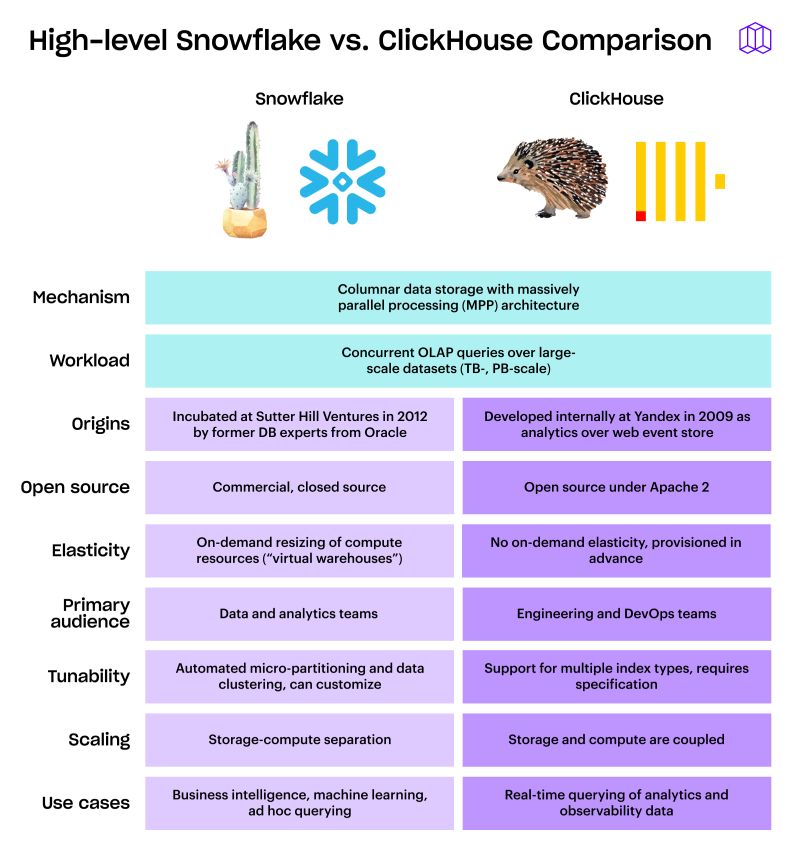


