Snowflake Spend Monitoring in Metaplane
Read more about Metaplane's newest feature for Snowflake users who are trying to get the most out of their data stack.
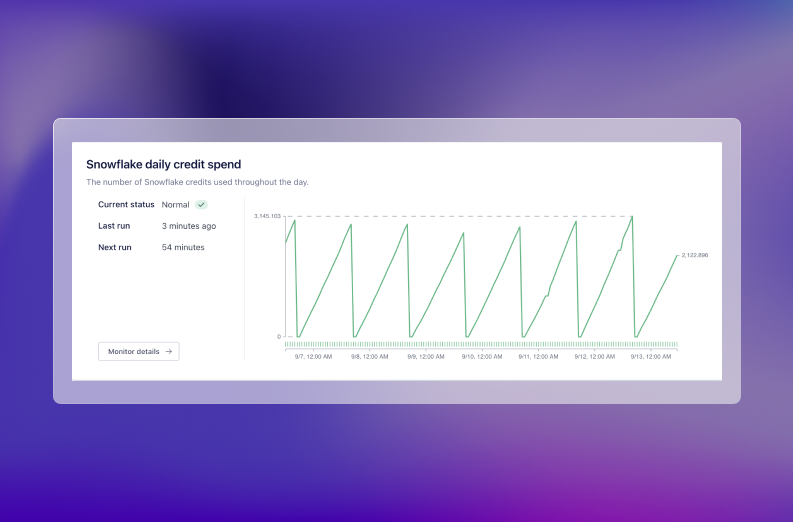
Imagine this: you’ve just taken the proverbial wrapping paper off of your shiny, new Snowflake warehouse. The team is overjoyed with how easy and cheap it’s been to store almost unlimited amounts of data, and how easy it’s been to scale the power of Snowflake to accommodate long running queries. Even better, the team’s hard work in implementing Snowflake to serve more requests has been noticed, and the data department is now an essential part of all critical decision making processes.
Then, one day, you take a look around and realize:
- You’ve grown from three compute warehouses in your initial implementation to 15, segmented by different workloads
- You now have dozens of users in Snowflake, some of which are service accounts
- You have thousands of queries running daily, with the majority being automated through dbt or other tools
Your data team has helped grow the business—in large part because of the success of implementing Snowflake. But now the business is reaching a point where efficiency becomes an important consideration. You start to see the abbreviations “TCO” and “ROI” on slides in your goal-setting decks and written all over internal docs.
You start to look at improving how you’re spending money with Snowflake. But you want to do it while also being conscious of your growing business, ensuring that any workloads you optimize don’t have unintended negative effects—you don’t want to end up in a situation, for example, where you’re blindly changing join types without understanding the underlying table structure.
Metaplane for Snowflake spend
Luckily, your team is already using Metaplane, which means that you have access to one of our latest features - Snowflake Spend Analysis. With this tool, you can immediately see your daily total credit spend, a 30-day spend aggregation, and your daily spend broken down by warehouse and user.
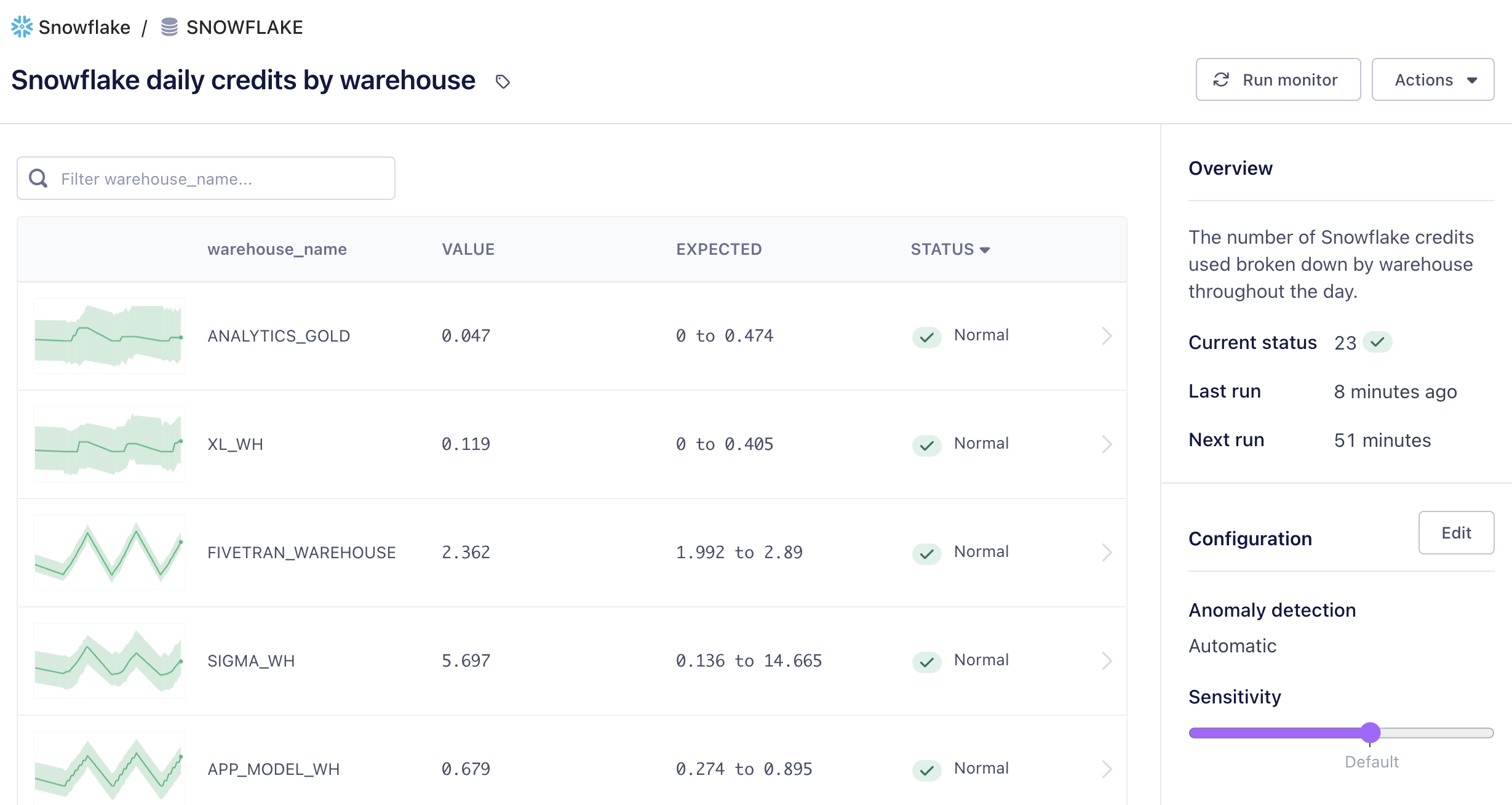
Moreover, Metaplane’s spend analysis dashboard is powered by the same machine learning capabilities that powers the rest of our monitors. This can help you catch and understand abnormal spikes or drops in credit usage. Your team will be able to:
- Capture upstream issues: For example, a misfiring pixel could lead to thousands of duplicate events in a table, directly increasing the length and credit usage of a modeling query merging that table. This is the sort of spike the spend analysis tool is made to capture.
- Confirm proper Snowflake configuration(s): Imagine that your team updated the AUTO_SUSPEND setting, but accidentally added an extra “0”, leading to longer active warehouses, and higher spend for that warehouse. Catching an issue like this is a perfect use case for spend analysis monitoring.
- Set up regular optimization efforts: Incorporate the dashboard into your optimization workflow to understand the largest contributors towards your Snowflake spend and discuss if those contributors are worth reviewing.
- Improve Total Cost of Ownership for your whole data stack: By splitting out credit usage by users and warehouses, your team is able to better understand which service accounts and/or “service” compute resources should be targeted for efficiency gains.
In short, our Snowflake Spend Analysis was built for you to get at-a-glance insights and alerts for where to focus your optimization projects.
What else does Metaplane do?
In this scenario above, your team didn’t even originally purchase Metaplane to optimize Snowflake spend. Instead, Metaplane was originally implemented to improve a different aspect of Snowflake: the quality of the data itself.
Similar to the spend analysis monitors, Metaplane has several monitor types that use machine learning, trained by your data, to understand what acceptable data quality metric thresholds should be. In this way, you can find data quality issues, without needing deep domain expertise or manual analysis of what constitutes an issue, and can avoid the maintenance associated with re-calculating acceptable thresholds when your data changes. On top of that, Metaplane also helps users resolve issues more quickly by finding the origin of a problem with column level lineage maps, and helps you avoid future issues by integrating directly into your CI/CD workflows.
If you’re interested in a future where your team can optimize Snowflake while improving your confidence in your data, set up a Metaplane account or talk to our team today!
Table of contents
Tags
...
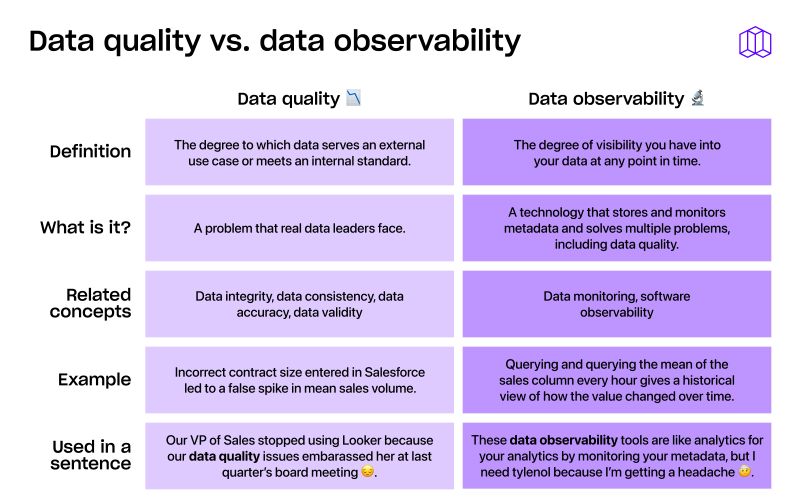
...
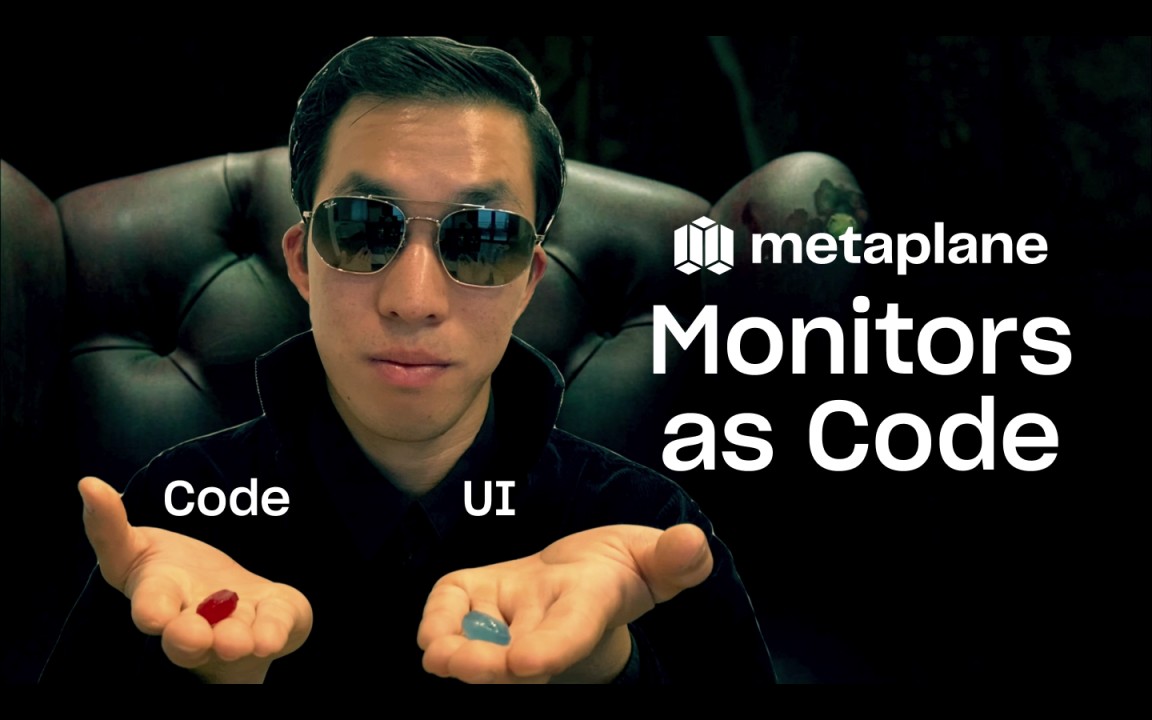

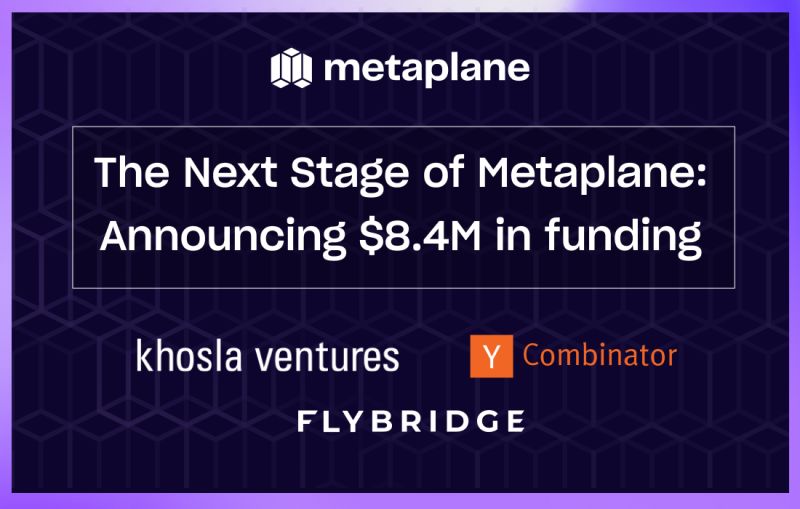
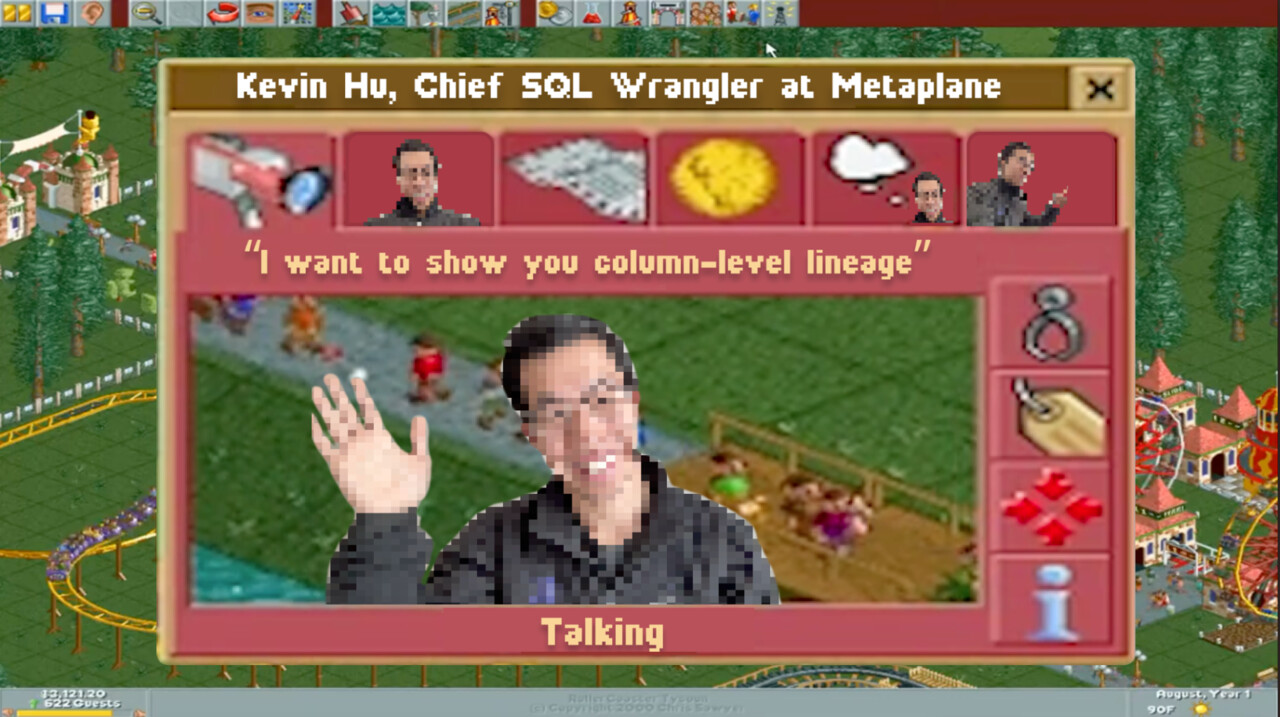
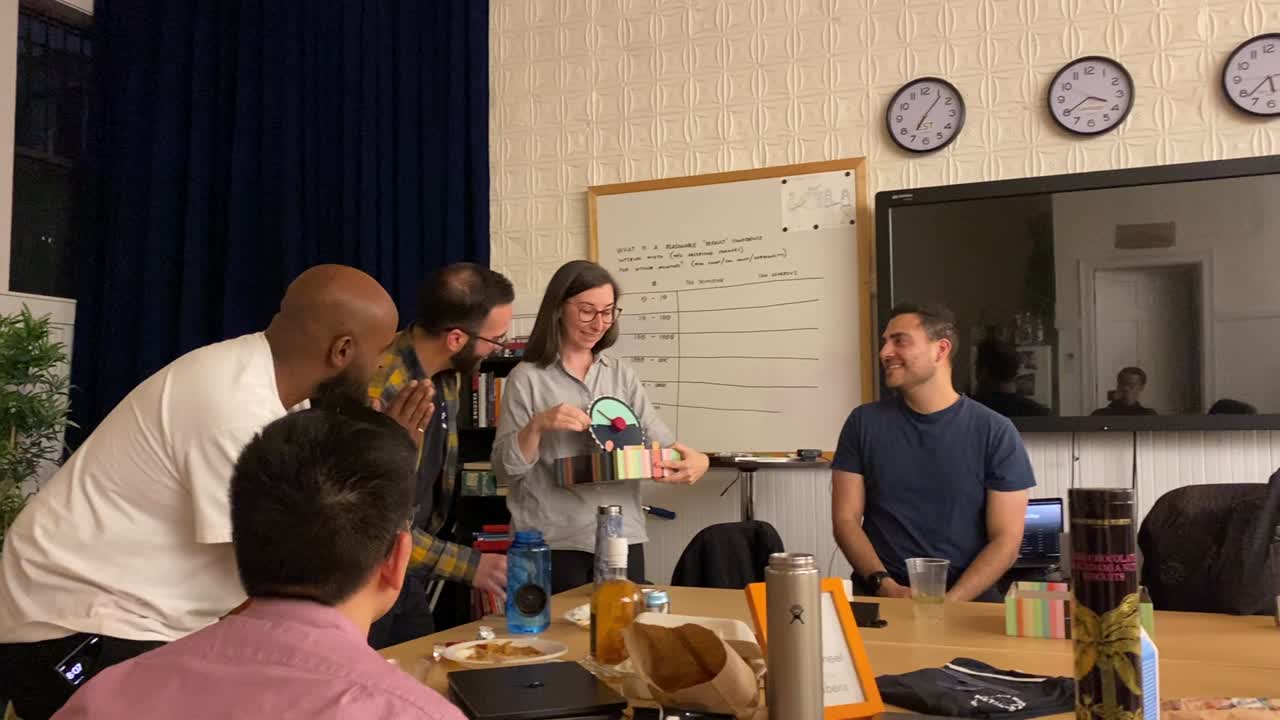