The Importance of Data Quality for PLG Companies
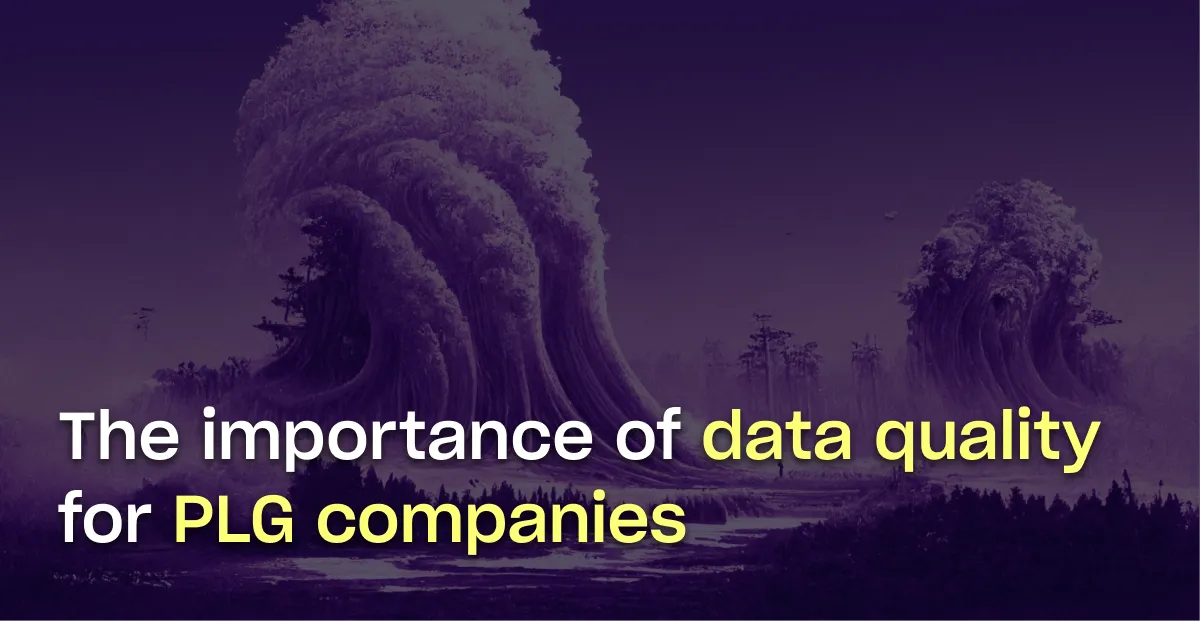
Driven by success stories like Slack (which reached a $7B valuation in just five years 🤯), Datadog, and HubSpot, product-led growth (PLG) has become a hot topic in the software world.
Defined by OpenView as “an end user-focused growth model that relies on the product itself as the primary driver of customer acquisition, conversion, and expansion,” PLG often better reflects consumer buying behaviors, even in enterprise sales cycles. In fact, as Harvard Business Review reported in Feb. 2022 that 43% of B2B buyers prefer to buy directly, without speaking to a salesperson.
PLG initiatives don’t just help folks talk with salespeople less (no offense sales folks), but often results in other major benefits over more traditional sales- and marketing-led growth:
- Often, the product is better. Product quality is top-of-mind and more resources are allocated to product development and testing, rather than sales and marketing.
- Prospects are motivated and qualified when they enter the sales funnel. They aren’t making a purchase decision after being wined and dined by a salesperson—they’re genuinely interested in the product itself.
- Stronger unit economics. With word-of-mouth and a funnel of customers who are already familiar with the product, marketing and sales teams don’t require as much effort to acquire new customers.
So what does the importance of data quality for PLG mean for data leaders? Short answer: Data is gold, and the data team are the stewards and keepers of that gold. Not only is high-quality data pivotal to the success of PLG companies, but this push toward data-driven business practices puts data teams in the driver seat.
As they say, with great power comes great responsibility.

How data quality uniquely impacts PLG companies
Product-led companies are fundamentally data-driven. Data about the product, customers, and users facilitates every part of the PLG business and the product behind it. PLG companies often use product analytics data to automate marketing campaigns, inform sales outreach, and customize user experiences.
Given the central role of data, problems with data quality–both human error (typos, inconsistent variables, etc.) and machine error (syncing delays and broken APIs)–can have massive impacts on revenue, customer sentiment, team efficiency, and strategy and forecasting.
Data quality can make (or break) personalization efforts
Consumers expect a huge degree of personalization and customization today. In fact, more than 75% of consumers get frustrated when companies don’t personalize their interactions. We’ve become so used to this personalization that we hardly notice it until it goes badly. Imagine logging into Spotify tomorrow, but instead of seeing recommendations based on your listening, you see completely generic playlists. I suspect we’d see a big uptick in Spotify’s user churn.
Creating personalized experiences requires data, and a high quality of the data at that. This is a challenge for PLG companies. Most of us wouldn’t answer even 40 questions about our musical preferences during the signup process, which means that companies like Spotify instead rely on product usage data to tell the story of what users prefer and dislike.
The expectation that our products “know” us without ever asking questions bleeds over into the B2B world, too. Only it’s no longer just about knowing what an individual user needs, but predicting what their team and organization will need as a result of individual behavior.
This means guessing wrong about a singular user’s needs isn’t just about that specific person anymore, it means potentially failing to serve the needs of everyone within their org.
For example: Say you were using a meeting scheduling software that suggested invitees for meetings based on the folks you met with most often. If there’s a delay in syncing user account data to the suggestion engine, the tool could start recommending a colleague who has since left the team. What started as a helpful feature becomes annoying pretty quickly when the suggestions aren’t useful.
When data quality is high (and data teams have the tools they need to ensure it stays that way), companies can delight B2B and B2C users alike by anticipating their needs and incorporating their product feedback quickly. When data quality suffers, it's difficult for business teams in the org to know their customer and, as a result, customers often feel the negative impact of subpar personalization and look to find a product or company that can meet their unspoken needs more effectively.
Data quality can support or sabotage product development decisions
Digital products can collect terabytes of usage and customer data every hour. That data, combined with product analytics tools like Segment, Snowplow, and Amplitude, carries huge possibilities for understanding user behavior. Teams are no longer forced to make development decisions based on months-long user interview projects or (worse) a product manager’s gut instinct. On the other hand, both the complexity of user journeys and the high volume mean that managing and analyzing the data is a challenge.
Take this common example: Should we invest in improving performance for feature A or building new feature B? Product usage data identifies that 20% of customers who churned had experienced slow performance with feature A. With those analytics, the development team can feel confident bumping a requested new feature to prioritize a performance fix.
However, that decision-making is only as good as the data it’s based on. Something as simple as duplicate database rows could overstate the correlation between feature A’s performance and customer churn, leading the development team to prioritize a feature that doesn’t matter to many users.
With PLG, providing a good product experience to users is at the heart of every decision. Without data to reflect what’s a “good” or “bad” feature, business teams (and the data teams that support them) are left blindly guessing at the needs of their users.
Understanding customer usage leads to better informed decisions across every team and better products as a result, but only as long as the data is good.

Data quality can optimize or bloat sales and marketing campaigns
At PLG companies, customer and behavioral data doesn’t just feed the product and customer success teams. It fuels personalized (there’s that word again 😉) marketing and sales efforts, as well.
Data quality is particularly important to sales and marketing teams within PLG companies for two reasons:
- The efficiency of targeting in both growth campaigns and branding is heavily impacted by insights from customer data.
- Many of these teams work in early stage companies where resources (time, money, and staff) are limited. Bad data quality = wasted budgets.
When data accuracy and quality is high, sales can go into every conversation with data to back up their understanding of the customer’s needs and goals, as well as outbound to prospective users with price messaging that demonstrates they truly understand the person on the other side.
Even outside of “traditional” sales conversations, high-quality data impacts sales of PLG products by determining how accurate (or not) copy on product pages, pricing plans, and in-product is to the customer’s needs.
The same holds true for marketing teams, who rely on customer and usage data to help them build out user segments on platforms like LinkedIn and Facebook, understand the unique personas using the product, and create top-of-funnel content that speaks to those users' pain points.
Let’s say, for example, that you’re building a productivity app for engineering teams. Good, accurate data would tell the sales and marketing teams what features and use cases you use most, which they would then highlight on the home page and pricing pages (alongside a free demo offer) to entice you to sign up and give the tool a try. When it came time for you to pivot to a paid plan, a healthy, data-driven PLG model would upsell you based only on the features you used most vs a generic plan.
PLG companies rely on data-driven sales and marketing tactics to drive business value, but inaccuracies or errors in data can result in lost revenue, low conversion rates, and wasted effort.
Data quality can build up or break down organizational trust in data
Data is only helpful if decision-makers trust it. One survey from 2021 revealed only 40% of execs have a high degree of trust in their company’s data. When business stakeholders catch data issues and have to go back to data teams for answers, it degrades their trust in their organizational data. Once that trust is lost, it’s hard to build back.
For every organization, no matter their GTM model, a lack of trust in data is problematic and often leads to poor strategic business decisions and wasted time and money.
For PLG companies, the stakes are higher. When data consumers don’t trust the data available to them (or the insights from their data teams), they fall back on gut assumptions about what customers want and need from the product. But with most customers taking a self-serve route to product adoption, these consumers are missing out on data points that they could otherwise gather by talking to users 1:1 during their buying process.
In the absence of trust in data or the data team, stakeholders go rogue, collecting and managing their own data within their teams to measure against key metrics and make decisions. This results in data silos and a kind of data telephone that leaves everyone constantly chasing organizational truth.
On the other hand, with better data quality insights are accurate and readily available from a single source, and data consumers know they have a trusted ally in the data team. High-quality and reliable data means greater data integrity across the business.
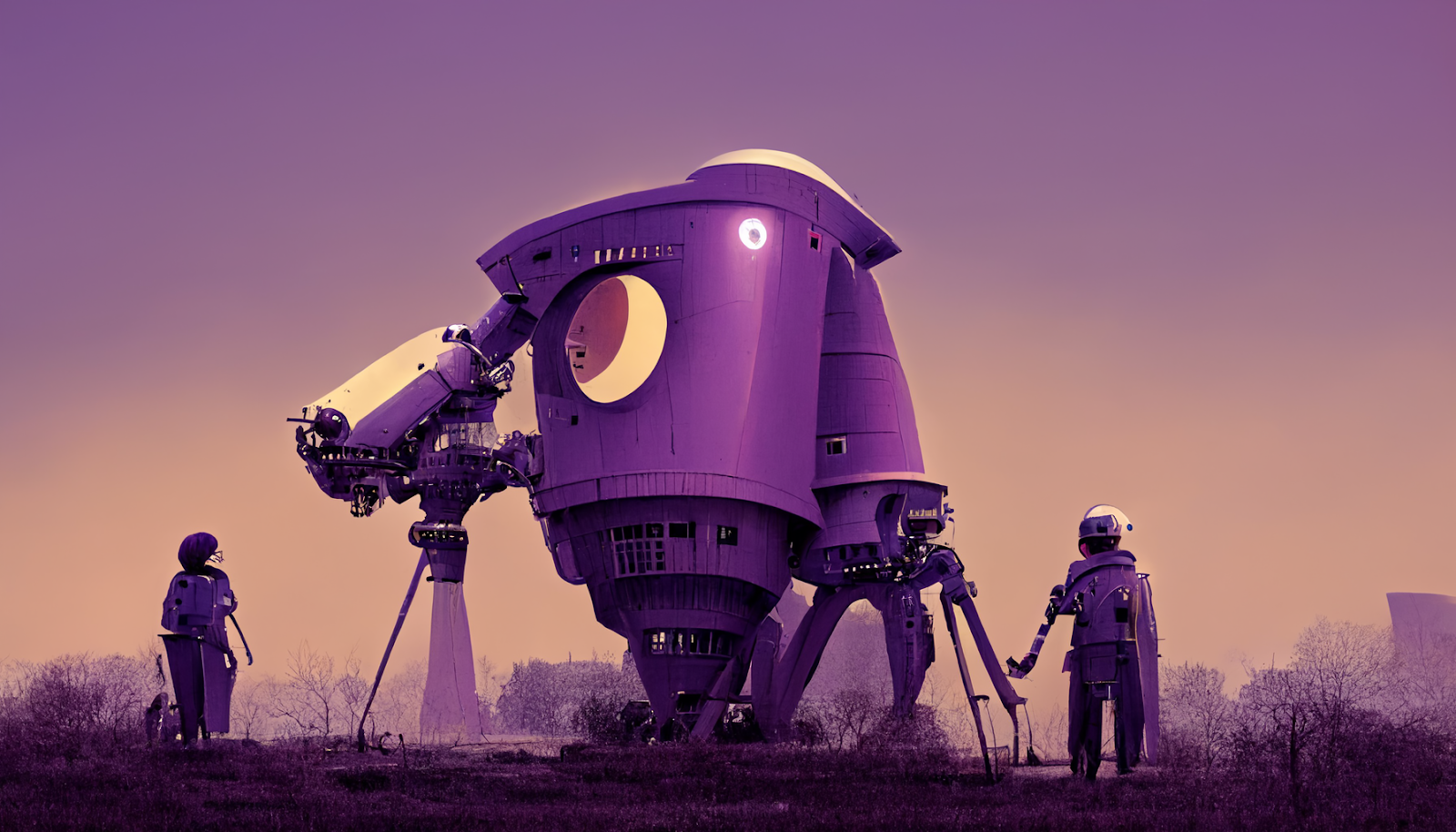
Data observability offers an ounce of prevention for data quality management
As the saying goes, “An ounce of prevention is worth a pound of cure.” As tired as it is, the principle holds true when it comes to data quality management. Unraveling the damage caused by poor-quality data can take days or weeks of effort. When data teams can identify the issue right away, and get insight into how to fix it, it makes it easier to troubleshoot and prevent that inaccurate data from affecting downstream decisions.
Unfortunately, bugs are inevitable in data just as in software. Developers have long included observability tooling in their stacks, and, as best practices from software engineering make their way into data governance best practices, are becoming a cornerstone of the modern data stack, too. That’s why we built Metaplane: To offer data teams the same level of data observability power that software teams enjoy from tools like Datadog.
Here are some of the features you should look for in data observability tools if you’re working on data teams within PLG companies to help ensure high data quality:
- The ability to identify and alert to data quality issues. Often data issues lurk until they break our pipelines or downstream reporting. Real-time observability tools give data teams the ability to not only monitor data easily, but receive error alerts and troubleshooting details in the channels they use most (Slack, PagerDuty, email, etc.).
- Easy-to-use anomaly detection based on historical metadata. You likely don’t have time to add more manual data work to your plate. A dedicated data observability tool should give you the ability to train anomaly detection models on historical metadata to easily identify outliers.
- Insights to help you triage data quality issues. Not all data quality issues are created equal. Data observability tooling gives you context through automated warehouse-to-BI lineage to help you see which data source errors impact important downstream destinations so you can act fast where it matters.
- Tests you can set up quickly without writing code. Time is one of a data team’s most previous resources. You shouldn’t spend it writing dozens (or hundreds) of tests. A dedicated observability tool latest you deploy code-based tests in minutes by supporting foundational (row counts, schema drift, etc.) and complex tests (nullness shifts, enum changes, etc.) out of the box.
Data observability tooling can help every data team build trust in their organizational data, but is particularly important for those embedded within PLG companies where high-quality data is paramount to the company’s success.
Metaplane provides critical tools for data observability, ensuring PLG companies can demonstrate the importance of good data quality to data consumers. If you’re just getting set up the modern data stack and wonder if you need an observability tool yet, check this resource out.
If you’re ready to learn more (or just need an expert ear to answer your data quality questions), try us out for free. We’re happy to help.
Table of contents
Tags
...

...

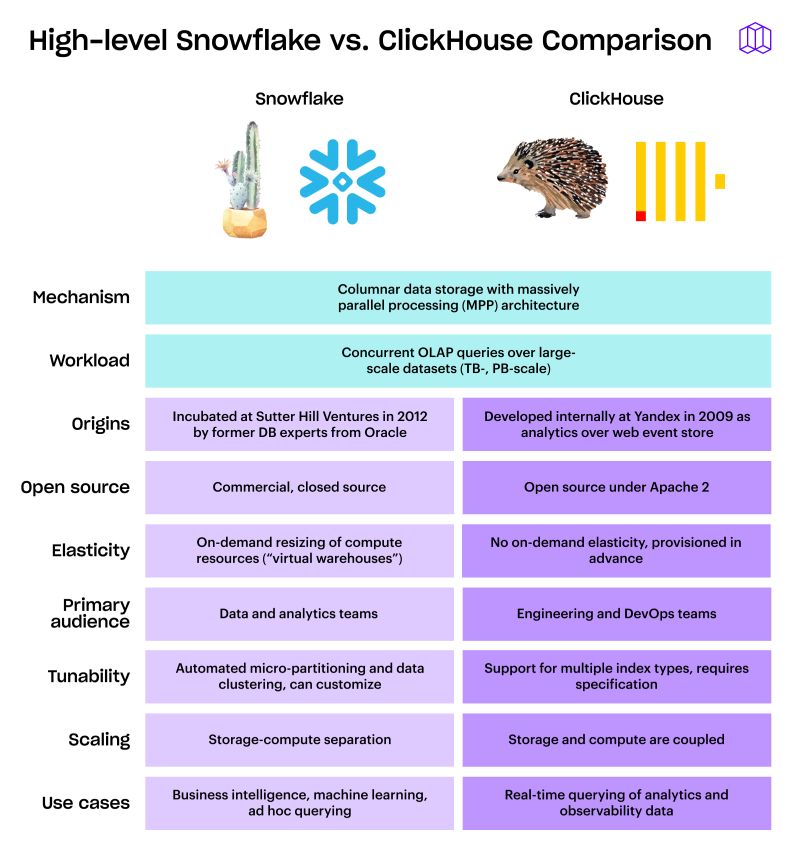


