The Most Common Misconceptions About Data Observability
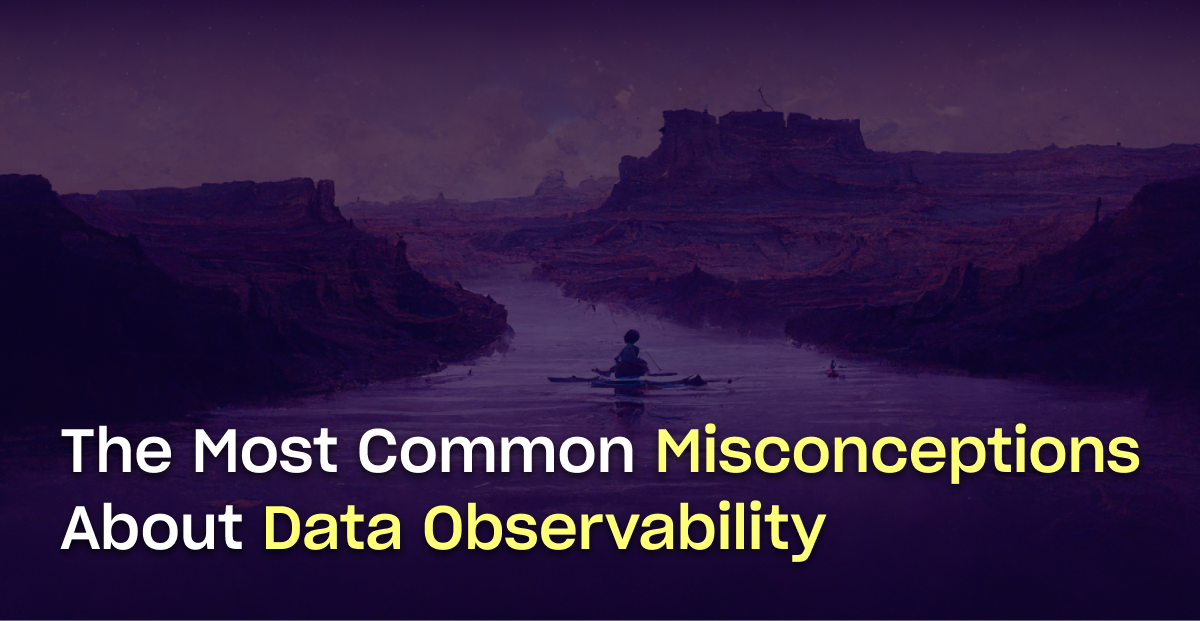
As the new kid on the block, data observability isn’t always understood—even by the most experienced data engineers. It’s not uncommon for data observability to be mistaken for software observability, for example. And even those who understand the concept jump to conclusions about how the technology functions, who benefits from it, how much it costs, and how long it takes to implement. In this blog post, we call out and correct the misconceptions about data observability we hear most often.
Myth #1: Data observability is only for big data
One common misconception is that you need a certain number of tables before data observability becomes relevant. That’s not the case. In fact, the size of your data has little impact on whether a data observability tool would be useful to your organization. The only question that matters is whether your data is being used by your company, either for operational or decision-making purposes. Every company that leverages its data has at least one use case for data observability.
Myth #2: Data observability is only for big teams
Another misconception we hear often is that your data team is too small to benefit from data observability.
The truth is that data quality issues don’t always correlate with team headcount or company size. Compare the importance of data to these two companies: Company A has 1,000 employees and a 10-person data team. Data supports strategic decision making, which has an indirect impact on the company’s bottom line. Company B has 100 employees and a one-person data team. Data fuels the company’s operations, which has a direct impact on the company’s bottom line. Which company has a more pressing need for data observability? Ultimately, the stakes are higher for Company B.
Small teams don’t have the resources to throw people at their problems, so they must maximize their productivity. To protect their time and energy, they need the metadata that reveals what data assets are being used, by whom, how frequently. This information empowers small teams to effectively prioritize their work. Bigger teams, on the other hand, often struggle to attract and retain senior data engineers. When onboarding new team members, they need end-to-end visibility into their data pipeline to give them a lay of the land and set them up for success.
Our point is simple: Regardless of your size, if your company makes use of the data you work so hard to deliver, you deserve a data observability platform.
Myth #3: Data observability into one system is enough
Many people make the mistake of believing they can just monitor their warehouse. After all, it’s their data’s source of truth. However, this couldn’t be further from the truth.
Your warehouse is a destination, not the source of the data. When a data quality issue occurs in your warehouse, you’re detecting a symptom (stale data), not the cause (e.g., delayed ELT sync). It’s too late at that point; the problem has already spread. If you want to catch data quality issues before they have a chance to cascade and compound, you need to monitor your data from its entry point.
Another reason it is essential that you monitor your entire data pipeline, from upstream transactional databases to downstream business intelligence dashboards, is because without it you wouldn’t be able to conduct root cause or impact analyses—two critical investigations data observability tools make easier through features like usage analytics and lineage.
Myth #4: Data observability requires full test coverage
Many data engineers think they need full test coverage from day one. But perfect doesn’t have to get in the way of good. Marion Pavillet, Senior Analytics Engineer at Mux, recommends starting small when adopting data observability for the first time. The t-shape framework she follows encourages you to go deep on your most important data assets and broad to capture everything else. For example, you might add freshness and volume tests to every table in your warehouse but a whole suite of advanced tests to your most frequently queried tables.
Our point is that it’s okay to expand your test coverage over time to serve a greater depth and breadth of use cases. Data quality improvement can feel like pushing a boulder up a hill—the pursuit often has no endpoint in sight because data is dynamic by nature. As a result, delivering high-quality data can be a moving target. A good data observability solution will grow and move with you to ensure you hit the mark.
Myth #5: Data observability is so expensive it’s inaccessible
Many tools on the market are exorbitantly expensive, with starting prices that are higher than the cost of your warehouse. At Metaplane, we believe it should be affordable for even the smallest teams to bring on a data observability solution. After all, our mission is to help all companies trust their data. That’s why we offer solo data engineers a baseline of tests and other key features, like schema change detection, for free. We’ve also adopted a usage-based pricing model (similar to Snowflake and dbt) to ensure we grow with you as your team expands and needs evolve.
Myth #6: Data observability takes a minimum of one quarter to implement
Data quality initiatives have a reputation for being long and drawn out processes at large companies. They’re often quarterly, if not annual, projects with dedicated owners and resources. Even for small teams, it can take a long time to evaluate and implement data observability tools when lengthy sales processes are the norm. For these reasons, many data engineers expect implementing a data observability tool to take at least one quarter.
This misconception always pains us to hear because Metaplane is designed to be set up in an afternoon. The average customer implements our platform in under 30 minutes, and many customers do it in under 10 minutes. As a result, you’re able to start collecting metadata and detecting anomalies in your data right away.
Myth #7: Data observability equals data quality
In an ecosystem where data observability software companies publish endless amounts of content about improving your data quality, it’s no wonder why some data engineers mistake the two terms for synonyms. Data observability and data quality also get confused because they share at least one goal: to increase stakeholder trust in an organization’s data.
One important differentiator between the two terms is that data quality is a problem, whereas data observability is a solution. Data team leaders aren’t kept up at night thinking about data observability, but thoughts of data quality issues may in fact haunt them. They may dread the next morning, worrying that they’ll wake up to a WTF message from one of their key stakeholders, questioning whether they can trust their data.
Data observability is a technology that can solve data quality problems. That said, it’s not the only solution. Data quality issues can also be prevented through thoughtful applications of people and processes, or through data unit and regression testing. Similarly, solving data quality issues isn’t the only application of data observability tools. Valuable use cases include impact analysis, root cause analysis, spend monitoring, usage analytics, schema change monitoring, and query profiling, among others.
Myth #8: Data observability equals software observability
Data observability may be inspired by software observability, but they differ significantly. While the goal of data observability is to do for data teams what software observability did for software teams, software observability tools monitor systems, not data. Therefore, they’re not suitable for data engineers because they can’t serve important use cases like root cause analysis and impact analysis. It’s also worth mentioning that the pillars of software observability (metrics, traces, logs) are distinct from the pillars of data observability (metrics, metadata, lineage, and logs).
From misconception to course correction
So, what have we learned?
Data observability is distinct from both data quality and software observability. It benefits teams of all sizes, regardless of their volume of data. If your data is used for business purposes, you deserve a data observability platform.
When looking for a data observability tool, remember that monitoring your warehouse isn’t enough. You need end-to-end visibility into your data pipeline. On the other hand, full test coverage from day one is often unnecessary. It’s better to start small and gradually increase the breadth and depth of your tests.
Finally, not all data observability platforms are beyond your budget or desired timeline.
Ready to get started? Sign up for Metaplane’s free-forever plan or test our most advanced features with a 14-day free trial. Implementation takes under 30 minutes.
Table of contents
Tags
...
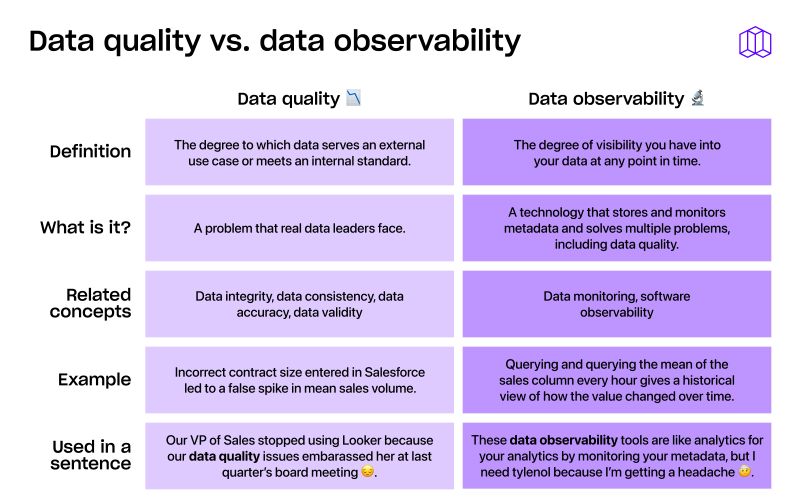
...
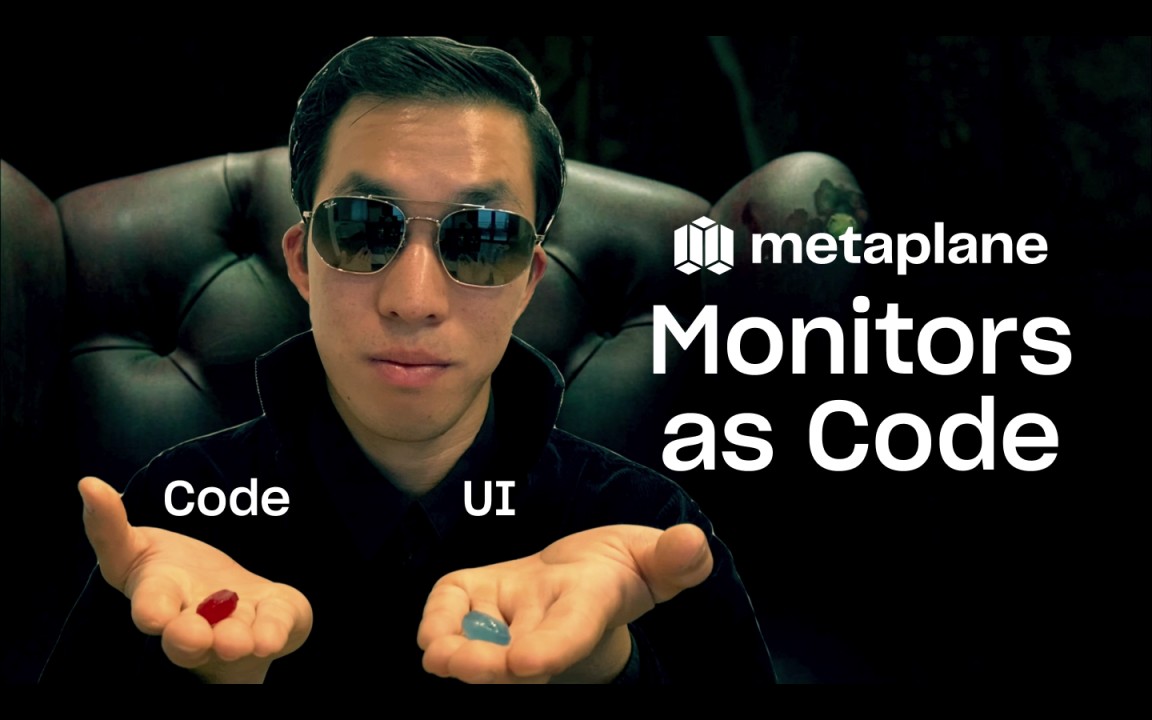

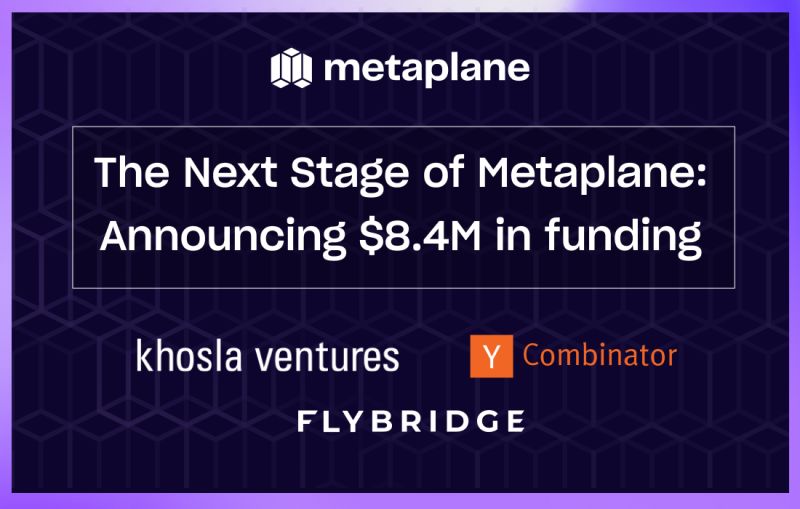
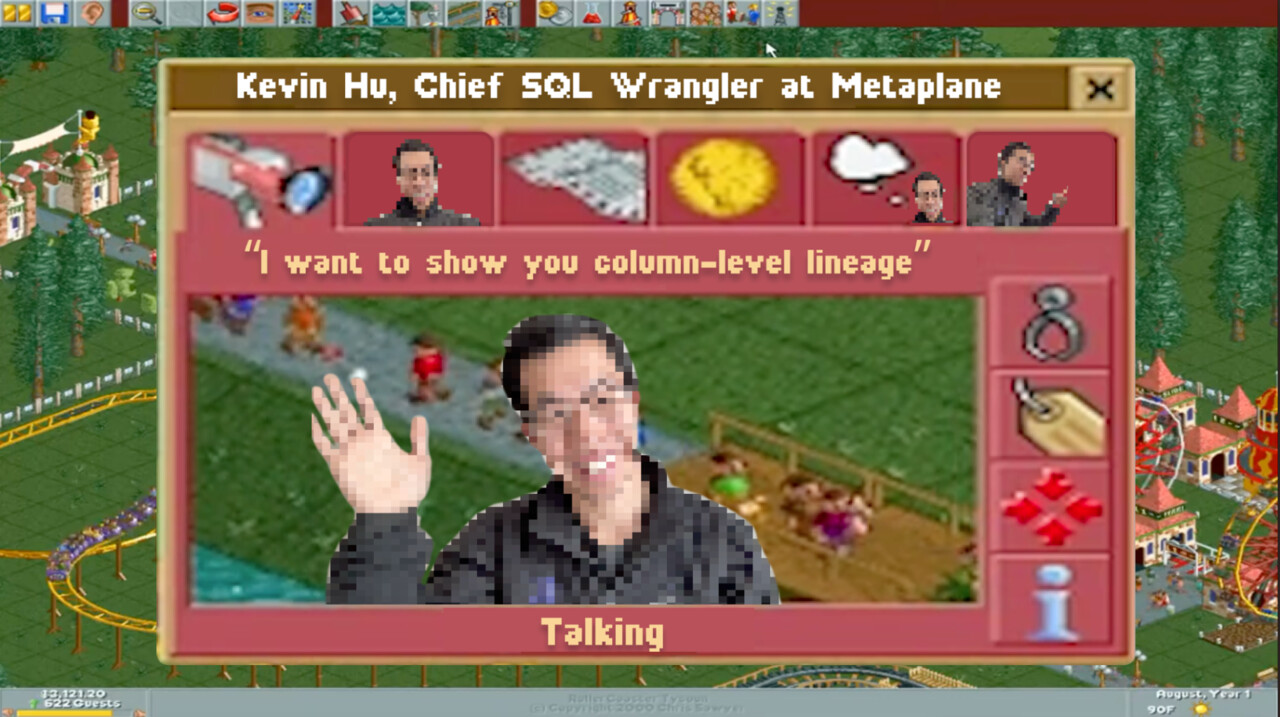
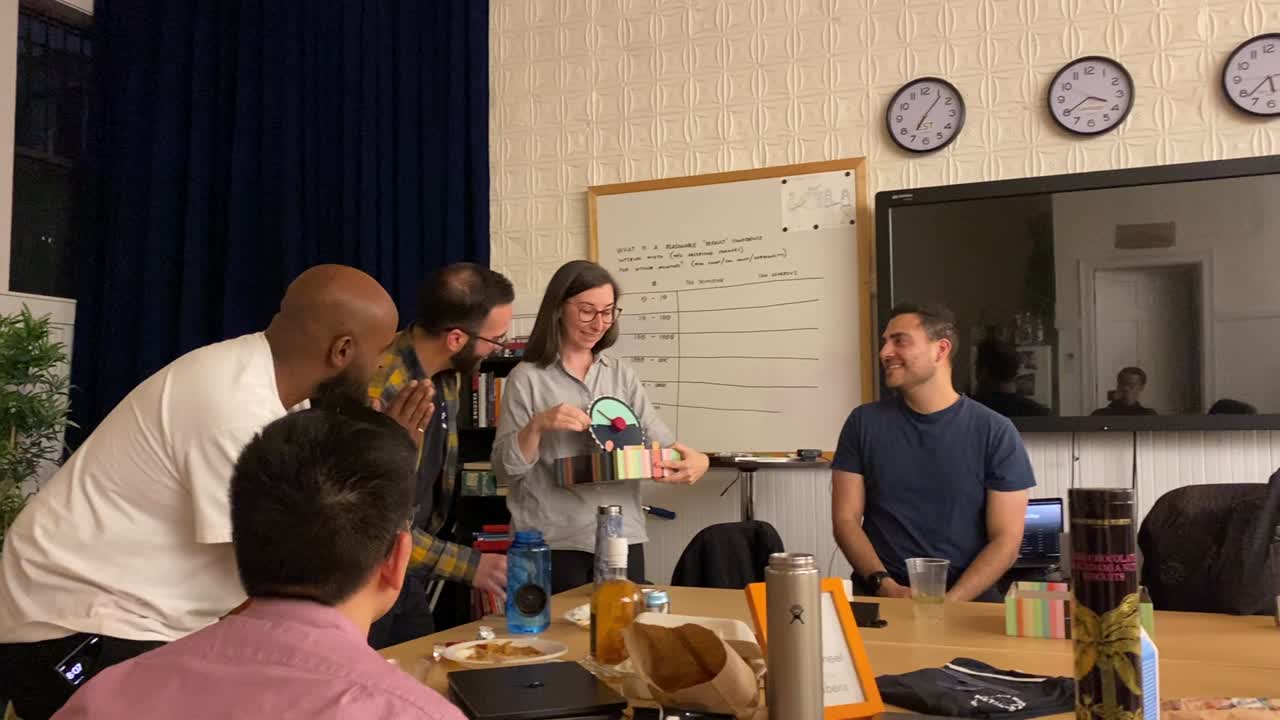