What is Data Relevance? Definition, Examples, and Best Practices
Do you know how much data your company collects? Nowadays, data is an essential part of businesses. However, not all data is created equal, not all data is relevant, and every company needs relevant data to make informed decisions. In this blog post, we'll cover the definition of data relevance, why it's important, and best practices for ensuring data relevance in your business.
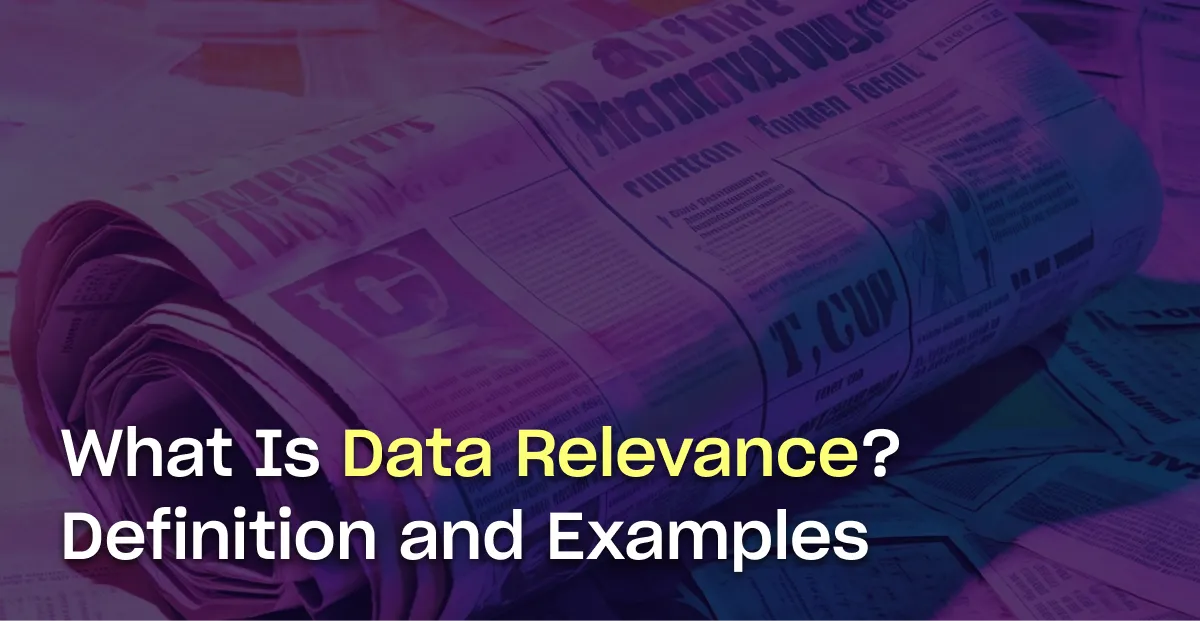
In one scenario, the activated_users table is used to send marketing e-mails. By leveraging data from this table, you could optimize your message timing or content to reduce churn and keep users longer in your platform.
In another case, the daily_revenue table is used by the VP of Sales to make decisions. You can use this table to measure the effectiveness of sales tactics across organic, paid, and email channels to identify opportunities for growth.
Both examples above outline target tables, and how particular rows, especially when split out by specific segments, can be relevant data depending on the downstream use case(s).
What is Data Relevance?
Data relevance is the degree to which data provides insight into the real-world problem or purpose being addressed and contributes to the overall understanding of the business. Data relevance is one of the ten dimensions of data quality, which also includes completeness, consistency, accuracy, and timeliness. Data relevance is critical because it is what sets the context of the issue at hand.
The distinction between relevance and other dimensions of data quality is important because relevance ensures your data is actionable and aligned with business goals. If you use irrelevant data, you'll generate inaccurate insights, make poor decisions, and damage your company's reputation.
Examples of Irrelevant Data
Irrelevant data occurs when data doesn't meet the criteria for relevance. Here are three examples of how irrelevant data can cause negative impacts on business analytics:

- Redundant Data: Data that repeats the same information multiple times is irrelevant because it doesn't add new insights. For example, if you're tracking daily revenue, you don't want to include multiple rows with the same information (i.e. two inputs for one day), as it would skew the results.
- Outdated Data: Data that's no longer accurate is irrelevant because it can lead to poor decision-making. For example, if you're using data from 2018 to make decisions about the current market, it's likely to be outdated and create incorrect insights.
- Incomplete Data: Data that's missing vital information is irrelevant because it hinders decision-making. For example, if you're trying to evaluate customer behavior but only have data on website traffic, your insights will be incomplete and less useful.
How Do You Measure Data Relevance?
The following are metrics and processes that teams can use to measure data relevance:
- Data usage: the amount of data that gets used in the decision-making process. By keeping track of data that gets used, data teams can identify patterns in the data that is used most frequently, leading to data relevancy insights.
- Time to analysis: the time it takes to analyze data reduced to an acceptable level. This metric is an indicator of data quality as well as the effectiveness of the data pipeline.
- Feedback: feedback provided by users that can help identify gaps in the data or the pipeline's quality. Feedback can also identify specific needs or features that the user requests.
How to Ensure Data Relevance
One way to ensure data relevance is to identify what user's needs are and collect data that provides context to the issue at hand. For example, you can use data from a total_activated_users table to inform user acquisition strategy. By monitoring this table's trend over time, you can identify which channels or tactics have high growth rates and invest more in those channels.
Data observability can also support data relevance initiatives by constantly monitoring and assessing the relevance and usefulness of data, using warehouse metadata including query and downstream BI tool usage, to help you more properly assess relevant data.
Summary
In conclusion, data relevance is vital because it ensures that the data you collect is up to date, reliable, and useful. By measuring data availability, quality, timeliness, processes, documentation, value, and cost, you can ensure that your data is relevant and aligned with business goals. By using a data observability tool like Metaplane, you can maintain data relevance, and create insights that generate higher ROI for your company.
Table of contents
Tags
...

...

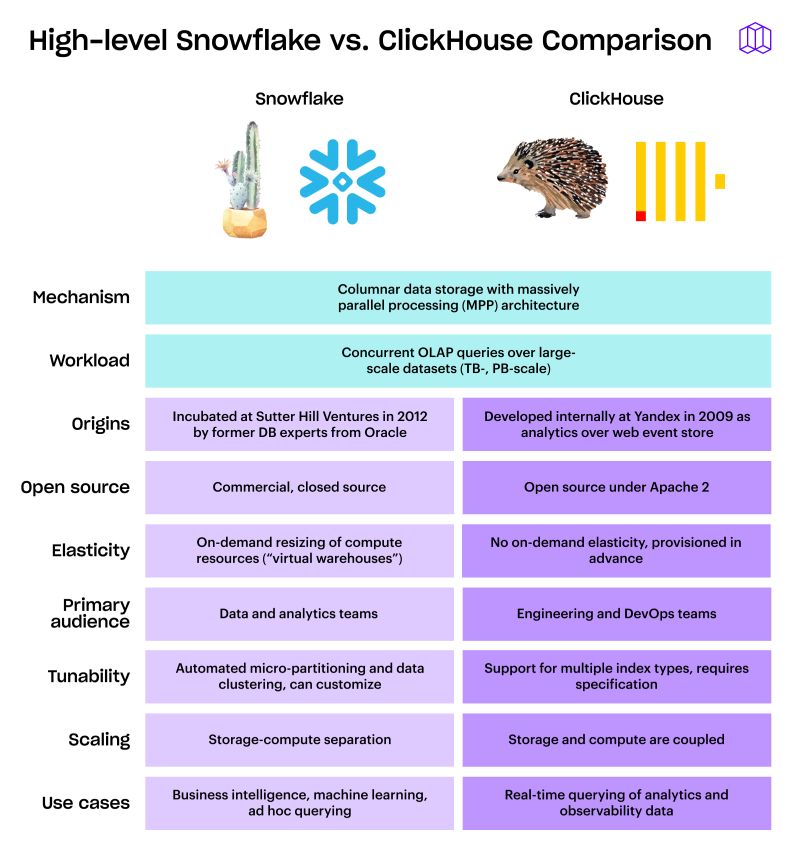


