What is Data Completeness? Definition, Examples, and Best Practices
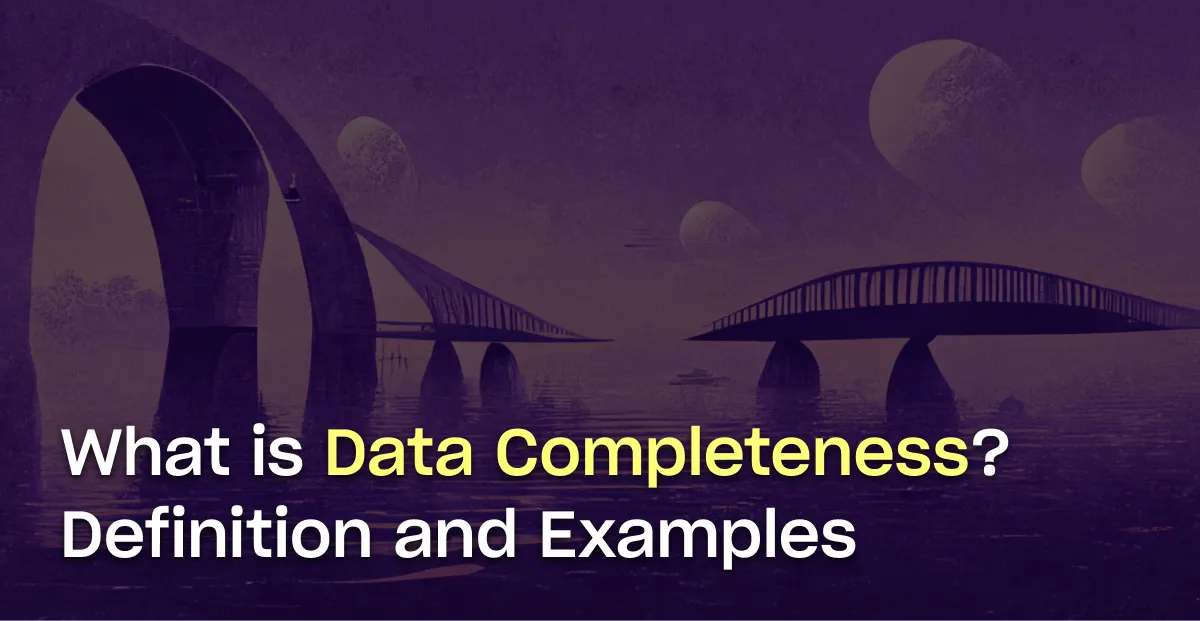
If you care about whether your business succeeds or fails, you should care about data completeness. Data completeness is important because it has a huge impact on your bottom line. Unfortunately, that impact often goes undetected—until it’s too late.
Say your business uses data for operational purposes, and your data is incomplete. You could fail to send re-engagement emails that help inactive free trialists realize value from your product, preventing them from converting into paying customers. The business impact would be less net new revenue than you had forecasted for the month.
If your business uses data for decision-making purposes, on the other hand, and your data is incomplete. If you know about the issue, it could prevent your executive team from making a time-sensitive decision. If you don’t, those same executives could analyze incomplete data, leading to an incorrect conclusion that adversely affects the business.
Now that you know why data completeness matters, let’s dive into exactly what it means. In this blog post, you’ll find a definition, examples, and four methods for measuring data completeness.
What is data completeness?
Data completeness is one of ten dimensions of data quality (which also includes timeliness and data accuracy). Data is considered complete if it is without missing information. There are at least two levels here. First, how complete is your data model? Second, within the data model you’ve constructed, how complete is the data itself?
What are some examples of incomplete data?
Imagine you’re a lead analytics engineer at Rainforest, an ecommerce company that sells hydroponic aquariums to high-end restaurants. Your data would be considered incomplete if the data warehouse was missing a table for a new product line of subscription fish food. The same would be true if within that table, the new prices of those aquariums were absent.
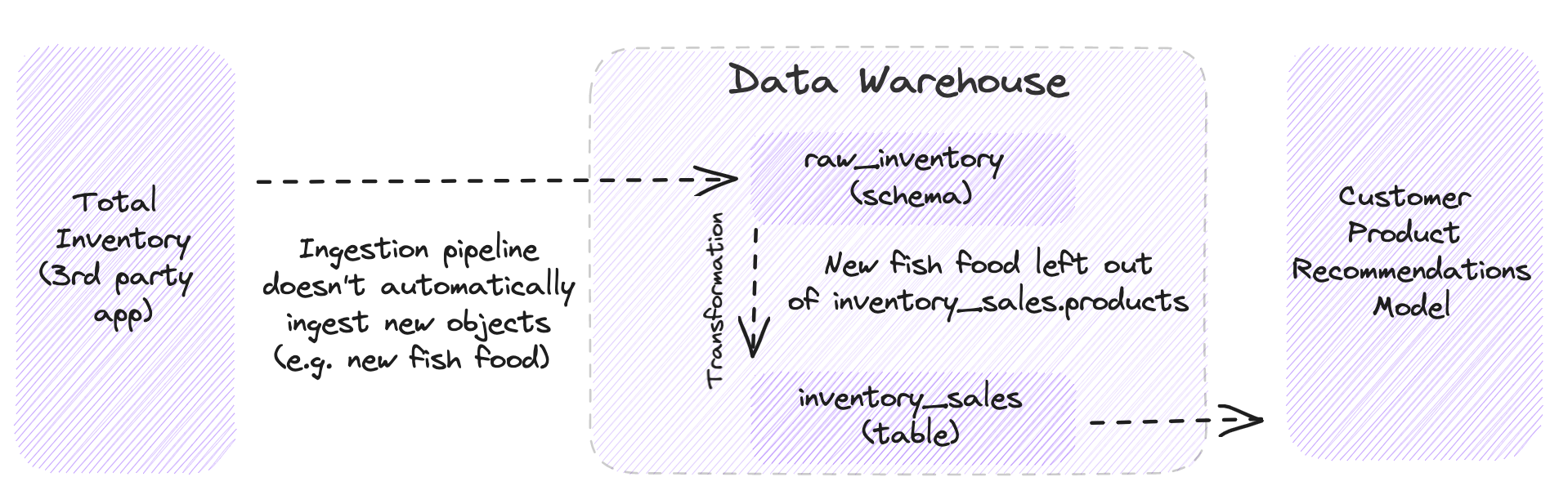
How do you measure data completeness
To test any data quality dimension, you’ll need to do some sort of data profiling; you must measure, track, and assess a relevant data quality metric. In the case of data completeness, you can measure the degree of validation against a complete mapping, the number of null data values or data elements or missing data, the number of satisfied constraints, or the degree of validation against an input mechanism.
How to ensure data completeness
One way to ensure data completeness is through anomaly detection, sometimes called outlier analysis, which helps you to identify unexpected values or events in a data set.
Using the example of prices absent from a table, anomaly detection software would notify you instantly when data you expect doesn’t arrive. The software knows it’s an abnormal state because its machine learning model learns from your historical metadata.
Anomaly detection helps countless data leaders detect incomplete data. Marion Pavillet, Senior Analytics Engineer at Mux, is one example. In her 2022 review of Metaplane, she wrote this: “Metaplane helps us stay ahead of data issues that we otherwise wouldn't detect. For instance, the pipeline runs correctly, but you are missing half the data.”
{{inline-a}}
Benefits of data completeness
To highlight the benefits of data completeness, let’s continue on with the scenario above. You’re pulling contact information (e..g phone number) from an upstream data source, to use in data analysis for purposes of understanding who to reach out to for an upcoming user group. If you had poor data integrity caused by poor data management, the ensuing data quality issues would lead towards inviting the wrong people for the user group. In a scenario where you’d like to use a user group to talk about the experience with your product, those data quality issues could result in a poor stakeholder experience, where the invited users have a significant lack of experience in the product itself.
To take anomaly detection for a spin and put an end to poor data quality, sign up for Metaplane’s free-forever plan or test our most advanced features with a 14-day free trial. Implementation takes under 30 minutes.
Table of contents
Tags
...

...

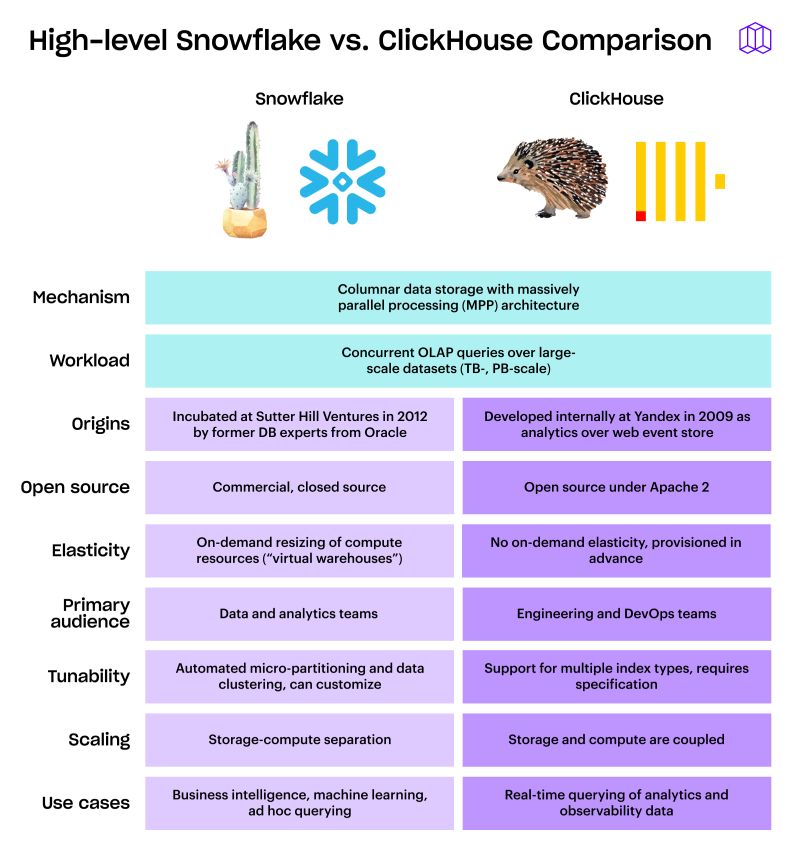


