Data quality fundamentals: What it is, why it matters, and how you can improve it
Data quality has a massive impact on the success of an organization. In this blog post, we highlight what it is, why it matters, what challenges it presents, and key practices for maintaining high data quality standards.
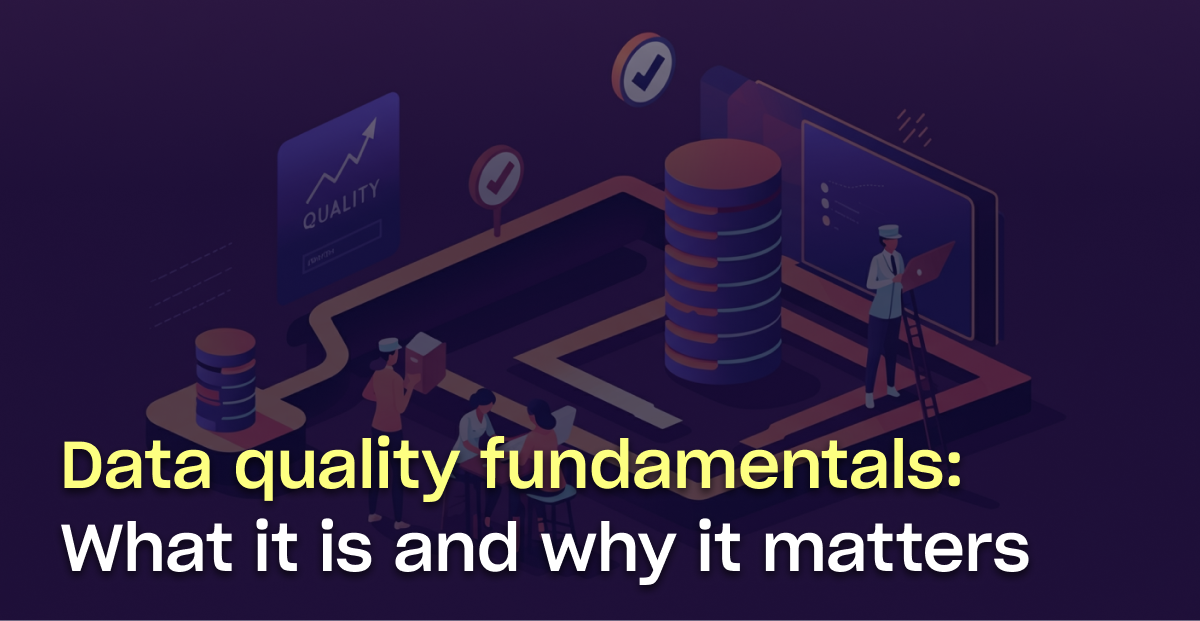
Data quality matters. In 2021, problems with Zillow’s machine learning algorithm led to more than $300 million in losses. A year earlier, table row limitations caused Public Health England to underreport 16,000 COVID-19 infections. And of course, there’s the classic cautionary tale of the Mars Climate Orbiter, a $125 million spacecraft lost in space—all because of a discrepancy between metric and imperial measurement units.
It’s real-world examples like these that emphasize how easily inconsistent data and poor data quality can impact even the biggest organizations. No wonder data teams are in such high demand. But supplying quality data stakeholders can trust isn’t easy. In reality, it requires that data teams master key data quality standards and concepts. Only then can they deliver on their mandate.
In this article, we highlight the fundamentals of data quality: what it is, why it matters, and how to measure and improve it.
What is data quality?
Before we get started, we need to understand a few key definitions:
- Data quality is the degree to which data serves an external use case or conforms to an internal standard.
- A data quality issue occurs when the data no longer serves the intended use case or meets the internal standard.
- A data quality incident is an event that decreases the degree to which data satisfies an external use case or internal standard.
What makes this definition powerful is its flexibility—it acknowledges that data quality isn't a one-size-fits-all concept. The same dataset might be high-quality for one use case and inadequate for another. Context matters.
Data quality vs. related concepts: Untangling the data reliability web
If you've spent any time in data circles, you've probably noticed terms like "data quality," "data accuracy," and "data integrity" being tossed around—sometimes interchangeably. But here's the thing: these concepts, while related, aren't identical twins. They're more like cousins in the data reliability family, each with distinct responsibilities.
Let's break down these differences so you can speak the same language as your stakeholders (and avoid those painful "we're talking about different things" moments in meetings).
Data quality vs. data accuracy
Data accuracy is laser-focused on one question: "Does this data correctly represent reality?" It's about whether the values in your database match what's true in the real world.
For example, if your system shows a customer made 3 purchases last month, but they actually made 5, that's an accuracy problem.
Data quality is the umbrella concept that includes accuracy but goes much further. It encompasses all ten dimensions we covered earlier—from consistency and completeness to usability and timeliness. Think of data accuracy as just one vital organ in the overall health of your data body.
When to focus on which:
- If you're building financial reports or compliance documentation → Accuracy is your primary concern
- If you're designing data products or analytics dashboards → You need to consider the full spectrum of data quality
Data quality vs. Data integrity
Here's another common mix-up. Data integrity is specifically about the structural soundness of your data: Are relationships between tables maintained? Are constraints being respected? Do foreign keys actually point to existing records?
In database terms, integrity focuses on:
- Entity integrity (primary key constraints)
- Referential integrity (foreign key constraints)
- Domain integrity (value constraints)
Data quality again takes a broader view, concerning itself with both the technical correctness AND the business usefulness of data.
A practical example: You could have perfect data integrity (all your joins work flawlessly) but terrible data quality (the information is outdated, irrelevant, or incomplete for your business needs).
Data quality vs. data governance
Data governance is about the people, processes, and policies that manage your data assets. It's the rulebook and organizational structure that determines who can access what data, how it's used, and who's responsible when things go wrong.
Data quality is more about the actual condition of the data itself. Good governance should lead to good quality, but they're not identical.
Think of it this way:
- Data governance is like establishing traffic laws and driving schools
- Data quality is the actual safety record of drivers on the road
You need both, but solving one doesn't automatically solve the other.
Data quality vs. data observability
Here's where things get interesting for data teams today. Data observability is about gaining visibility into the health and behavior of your data systems. It's your ability to understand what's happening with your data at any given moment and detect anomalies.
Data quality defines the standards your data should meet, while observability gives you the tools to monitor whether those standards are being met in real-time.
The relationship works like this:
- You define what good data quality means for your organization
- You implement observability to continuously monitor for deviations
- When observability detects a quality issue, you take action
This is why modern data teams are investing so heavily in observability—they serve as the nervous system that tells you when your data quality is at risk, before business decisions are affected.
The ten dimensions of data quality
There are ten dimensions of data quality that exist across two categories: intrinsic dimensions and extrinsic dimensions.
Let me create a clean, easy-to-scan table comparing the intrinsic and extrinsic dimensions of data quality.
Data quality dimensions at a glance
Intrinsic dimensions (independent of use cases)
Intrinsic dimensions are the foundational qualities that any dataset should possess, regardless of how it's being used:

- Data accuracy: Does the data correctly represent the real world? For example, if your data shows a customer made 5 purchases but they actually made 7, that's an accuracy problem.
- Data completeness: Are the data model and values complete? Missing values can significantly impact analysis outcomes.
- Data consistency: Is the data internally consistent? For instance, if the same customer is listed in multiple systems with different attributes, that's a consistency issue.
- Data freshness (or up-to-date-ness): Is the data up-to-date with the real world? Outdated information can lead to incorrect decisions.
- Privacy and Security: Does the data respect privacy commitments? This dimension has become increasingly important with regulations like GDPR and CCPA.
Extrinsic dimensions (dependent on use cases)
Extrinsic dimensions vary based on the specific business context and use case:
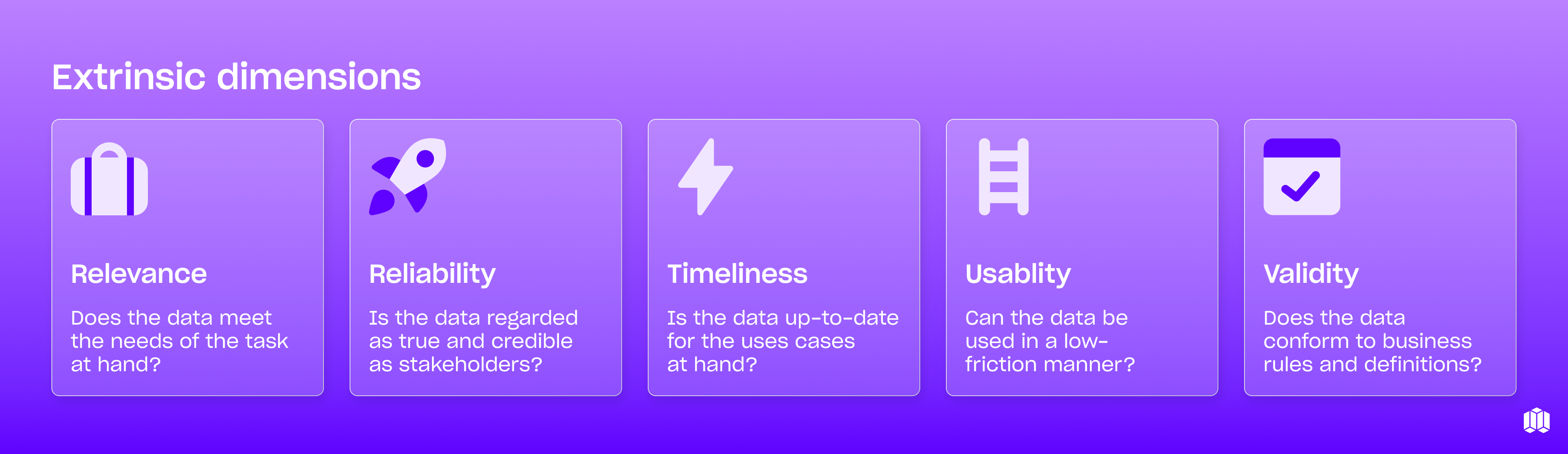
- Data relevance: Does the data meet the needs of the task at hand? Different business questions require different data points.
- Data reliability: Is the data regarded as true and credible by stakeholders? Trust is essential for data-driven decision making.
- Data timeliness: Is the data available when needed for the use cases at hand? Even perfect data is useless if it arrives too late.
- Data usability: Can the data be used in a low-friction manner? Data that's difficult to access or understand won't drive value.
- Data validity: Does the data conform to business rules and definitions? Each organization has its own standards for what constitutes valid data.
Extrinsic dimensions, on the other hand, are dependent on use cases, and include relevance, reliability, timeliness, usability, and validity.
Why is data quality important?
Data quality matters because it directly impacts your business performance. High-quality data helps you make better decisions and perform better, leading to increased revenue, decreased costs, and reduced risk. Low-quality data has the opposite effect, resulting in poor profitability and an increased risk that your business will fold prematurely.
All companies rely on data to determine their product roadmaps and deliver exceptional customer experiences. That’s precisely why high-quality data (and therefore data quality) is important absolutely crucial for making the right decisions for the right people at the right time. Among other things, it can:
Make (or break) personalization efforts
Did you know that more than 75% of consumers get frustrated when companies don’t personalize their interactions? That’s a startling statistic, but there’s a lot of merit behind it. Just imagine logging into Spotify tomorrow, and instead of seeing recommendations based on your listening, you see completely generic playlists. I suspect we’d see a big uptick in Spotify’s user churn.
Support or sabotage product development decisions
Decision-making is only as good as the data it’s based on. Something as simple as duplicate database rows could overstate the correlation between a feature’s performance and customer churn, leading the development team to prioritize a feature that doesn’t matter to many users. Without data to reflect what’s a “good” or “bad” feature, business teams (and the data teams that support them) are left blindly guessing at the needs of their users.
Optimize or bloat sales and marketing campaigns
Even outside of “traditional” sales conversations, high-quality data impacts product sales by determining how accurate (or not) copy on product pages, pricing plans, and in-product is to the customer’s needs. And the same holds true for marketing teams, who rely on customer and usage data to help them build out user segments on platforms like LinkedIn and Facebook, understand the unique personas using the product, and create top-of-funnel content that speaks to those users' pain points.
Build up or break down organizational trust in data
Data is only helpful if decision-makers trust it. And as of 2021, only 40% of execs had a high degree of trust in their company’s data (we can only hope it’s increased since then). But when business stakeholders catch data issues and have to go back to data teams for answers, it degrades their trust in their organizational data. Once that trust is lost, it’s hard to build back.
The top data quality challenges
Data quality problems span both machine and human errors, creating a complex landscape for data teams to navigate. Let's explore the most common challenges you're likely facing in your organization.
Machine-related challenges
On the machine side, we're seeing unprecedented software sprawl across organizations. With the average company now using 130+ SaaS applications, data lives in dozens of disconnected systems. This fragmentation creates natural friction points where quality issues emerge as data moves between systems with different standards and structures.
Data proliferation compounds this problem—we're generating more data than ever before, but quantity rarely equals quality. Modern data warehouses can handle the volume, but data teams struggle to keep up with quality checks at scale. What's worse, most data assets lack the crucial metadata that teams need to understand context. Without proper documentation about what fields mean, where they originated, and how they've been transformed, quality assessment becomes a guessing game.
Human-related challenges
The human side of the equation is just as challenging. Data creators inevitably make typos, misinterpret form fields, or enter information incorrectly. We've all experienced the classic "United Stats" vs. "United States" problem that throws off aggregations. Meanwhile, data teams themselves often operate with limited business context about what the numbers actually represent in the real world. This knowledge gap makes it incredibly difficult to spot subtle quality issues that might be obvious to someone with domain expertise.
And let's be real about the talent situation—data leaders face an uphill battle hiring experienced team members who understand both the technical and business side of data quality. This skills gap directly translates to reduced capacity for addressing quality issues proactively. Many organizations end up in reactive firefighting mode, only addressing problems after they've impacted business operations. By that point, trust has already been damaged.
It’s difficult for data leaders to hire experienced team members, which results in reduced capacity for addressing data quality issues. Check out the below webinar to learn more about the most common data quality challenges businesses face.
Whatever your challenge is, resolving it starts by conducting a thorough data quality assessment (and a good data observability tool like Metaplane can help you with that).
How to measure your data quality
Measuring your data quality is easiest when you start with the proper guardrails. Here's a simple data quality management framework to get you started:
1. Nail down what matters
What are you measuring, and why? Does your organization use data for decision-making purposes, to fuel go-to-market operations, or to teach a machine learning algorithm?
Getting to this answer will involve a combination of talking with the business stakeholders that use the data your team prepares and using metadata like lineage and query logs to quantify what data is used most frequently.
💡 Pro tip: Focus on the most important and impactful use cases of data at your company today. Identify how data is driving toward business goals, the data assets that serve those goals, and what quality issues affecting those assets get in the way.
2. Identify your pain points
Do you struggle with slow dashboards or stale tables? Perhaps it's something bigger, like a lack of trust in your company's data across the organization.
Pain points vary widely depending on the nature of your business, the maturity of your data infrastructure, the specific use cases for your data, and more.
Remember that the data team is neither the creator nor the consumer of company data—we're the data stewards. While we may think we know the right dimensions to prioritize, we need input from our teammates to make sure we're focusing on what matters.
3. Make metrics actionable
From your identified pain points, which data quality dimensions are relevant and how can you measure them?
Say you've implemented data metrics to track how many of the sales team's issues are related to data consistency. In response, you might develop a uniqueness dashboard of primary keys' referential integrity to check if deduplication is needed.
But if the dashboard data isn't connected to the issues faced by the team, they won't understand these numbers or why they're important. Make that connection obvious from the get-go.
4. Measure, then measure some omre
Measuring data quality metrics is the final step in the process. For example, you can measure data accuracy by comparing how well your data values match a reference dataset, corroborate with other data, pass rules and thresholds that classify data errors, or can be verified by humans.
Despite what you may think, there is no single right way to measure data quality. But that doesn't mean we should let perfect get in the way of the good. Start measuring, learn, and refine your approach over time.
Best practices for data quality management
No matter the people, processes, and technology at your disposal, data quality issues will inevitably crop up. To manage these incidents, you can follow our six-step data quality management process: prepare, identify, contain, eradicate, remediate, and learn.
- Prepare: Get ready for the data quality incidents you'll inevitably deal with in the future.
- Identify: Gather evidence that proves a data quality incident exists and document its severity, impact, and root cause.
- Contain: Prevent the incident from escalating.
- Eradicate: Resolve the problem.
- Remediate: Return systems to a normal state following an incident.
- Learn: Analyze both what went well and what could be done differently in the future.
Improving data quality through the PPT framework
At its simplest, achieving superior data quality requires applying a People, Process, Technology (PPT) framework:
People: Promoting a data quality culture
Your teammates can (and should!) help you develop data quality metrics from the ground up. Once these metrics are tangible, you'll also need their help to hold the organization accountable and play their part in ensuring high-quality data.
Consider establishing roles like:
- Data quality engineer
- Data steward
- Data governance lead
These roles can own the implementation and improvement of data quality metrics across the organization.
Process: How you approach data quality management
Think about what business processes you should put in place for data quality improvement:
- Low lift: The data team could perform a one-time data quality assessment.
- Medium lift: Implement quarterly OKRs around data quality metrics.
- High lift: Orient the entire organization around data quality with initiatives like:
- Training for marketing and sales teams on data entry accuracy
- Implementation of playbooks for remediation
- Regular data quality audits
Technology: Implementing data quality tools
Technologies can be your biggest advantage when improving data quality. Here are some of the different types of data quality management tools at your disposal and what they can help with.
- Data collection tools: Solutions like Segment and Amplitude help guarantee that your product analytics data is consistent and reliable.
- Data pipeline tools: ELT solutions like Fivetran and Airbyte ensure data is properly transformed and loaded.
- Operational tools: Reverse ETL solutions like Hightouch and Census use automation to check that all data from your sources is both up-to-date and timely.
- Specialized tools: There are dozens of data quality tools specifically designed for measurement, management, and cleansing.
How Metaplane helps with data quality management
At Metaplane, we live and breathe data quality so you don't have to lose sleep over it. We've built our data observability platform after seeing firsthand how data teams struggle with the same challenges over and over:
- That sinking feeling when your CEO asks why yesterday's revenue numbers look weird
- The frustration of diagnosing why a dashboard suddenly broke overnight
- The endless Slack messages asking "is the data correct?" that eat up your productive time
We're not just another tool in your stack—we're your early warning system for data quality issues. Think of us as the smoke detector for your data house. We monitor your data assets around the clock, watching for patterns and anomalies that humans might miss until it's too late.
Our platform connects to your data warehouse and automatically:
- Profiles your data to understand its typical patterns and behavior
- Monitors for unexpected changes in freshness, volume, schema, and distribution
- Alerts the right people when something looks off
- Helps you trace issues to their root cause through lineage visualization
The best part? We do this with minimal configuration, so you can get up and running in minutes, not weeks.
Final thoughts
Ultimately, you have to commit to best practices, like regularly conducting data quality checks and having a streamlined process for responding to data quality incidents. And, in tandem, you need to be proactive. Provide data creators with data entry training and periodically conduct data quality audits. Create the data infrastructure required to monitor your data quality as it ebbs and flows over time.
Carried out consistently, these practices, in conjunction with a data observability tool like Metaplane, can help you improve your data quality across your organization. Book a demo to see how Metaplane can help you build trust in your data, detect and fix problems quickly, and monitor what matters most to you.
Editor's note: This article was originally published in October 2022 and updated for accuracy and freshness in March 2025.
Table of contents
Tags
...

...

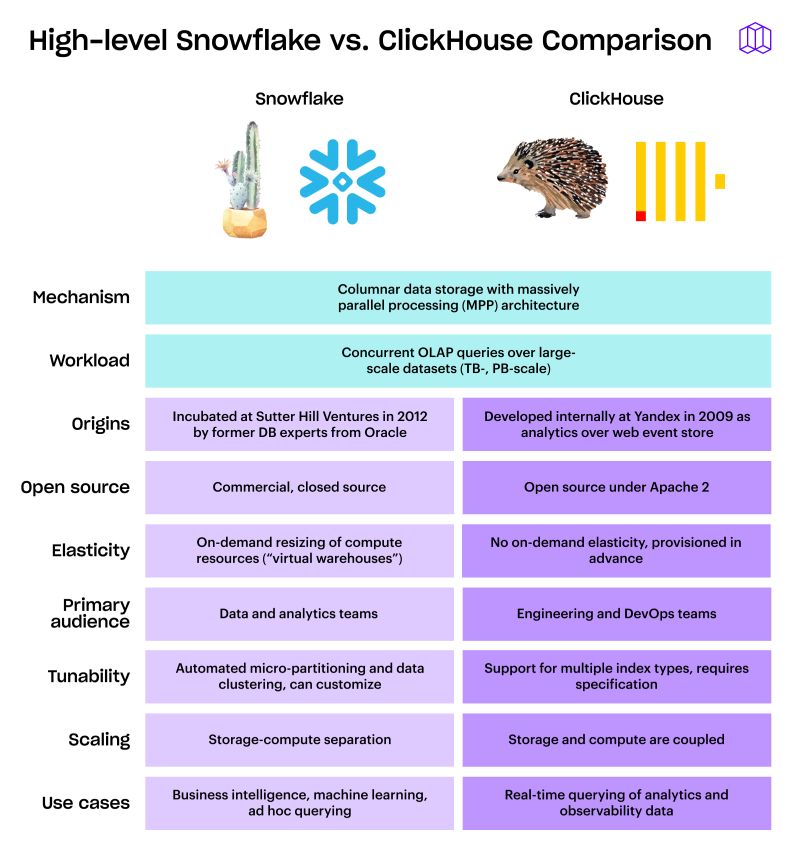


