What is data validity? Definition, examples, and best practices
What exactly is data validity, and how can you ensure that you're working with valid data? In this post, we'll explore the definition of data validity, its importance in data analytics, and best practices for measuring and maintaining data validity.

How confident are you that the data you're working with is actually valid?
Valid data is crucial for both operational and decision-making purposes. When data is valid, businesses can make accurate and informed decisions that can ultimately impact the bottom line in a significant way. For example, a sales leader might make regional expansion decisions based on revenue data.
What is data validity?
Data validity refers to how accurately your data reflects predefined business rules, constraints, or definitions. Essentially, valid data aligns with expectations and real-world scenarios. Invalid data, conversely, misrepresents or incorrectly records key information, leading to faulty analytics, incorrect business decisions, and lost revenue.
Data validity is one of the ten dimensions of data quality, which also include data completeness, data timeliness, data integrity, and data consistency, among others. Ensuring data validity is essential for maintaining overall data quality, which is critical for any data-driven business. Data reliability is fundamental in data analytics, ensuring the consistency and dependability of data over time.
Definition of data validity
Data validity is the extent to which data accurately represents the real-world entities, events, or measurements it is intended to describe. It involves verifying that the data conforms to predefined standards, rules, or constraints, ensuring that it is reliable, consistent, and accurate.
Data validity is a critical part in data management, as it directly impacts the quality and integrity of databases, and ultimately, the decisions made based on that data. When data accurately represents the intended metrics, businesses can trust their data analysis and make informed decisions with confidence.
Why data validity matters (with real consequences)
Valid data isn't just nice to have—it's essential for both operational efficiency and strategic decision-making. Here's what happens when your data validity breaks down:
- Operational failures: Your marketing team targets what they think are high-value prospects based on predictive lead scores, but the model was trained on invalid data—so they waste resources on poor conversion opportunities.
- Flawed decision-making: You launch a new product line based on survey data showing high customer interest, but the survey questions were worded to lead respondents toward positive answers—giving you invalid insights that don't reflect actual market demand.
- Damaged trust: After several reports containing invalid data mislead executives, every subsequent analysis faces increased scrutiny and skepticism—even the perfectly valid ones.
It's easy to draw the line from data validity to poor decision making and bad financial outcomes, but consequences can go far beyond that. imagine if a healthcare analytics team incorrectly mapped diagnostic codes that drove treatment decisions and assigned the wrong illness categories. It would lead to the wrong treatment recommendations, and consequences far beyond financial impact— affecting patient outcomes.
Types of data validity
There are several types of data validity, each affecting the quality and usability of the data in distinct ways. These include:
- Face validity: The most basic level of validity, where the data appears to be valid at face value, without rigorous statistical checks.
- Content validity: Ensures that the data collected adequately covers the research domain of interest.
- Construct validity: Concerned with whether the data measures the theoretical construct it is designed to measure.
- Internal validity: About the integrity of the experimental design in causal relationships.
- External validity: Deals with the generalizability of the data to other settings or populations.
- Statistical conclusion validity: Focused on the degree to which conclusions derived from the data are statistically valid.
- Ecological validity: Examines how well the data and its interpretations apply to real-world conditions.
Understanding these types of data validity helps businesses ensure that their data is robust and fit for purpose, ultimately leading to more accurate and reliable data analysis.
Common causes of invalid data
Even with the best intentions, data validity issues creep into our pipelines. Here are a few common ways it happens.
1. Measurement errors
The very instruments and methods we use to collect data can introduce validity problems. Your web analytics might double-count sessions due to incorrect tag implementation, particularly on pages with iframes or multiple load events.
This extends beyond digital tools, too. Survey questions with ambiguous wording lead respondents down different interpretative paths, creating data that reflects confusion rather than actual opinions. And physical measurement devices aren't immune either—temperature sensors can drift over time, producing readings that gradually deviate from reality without throwing obvious errors.
2. Sampling bias
Ever made a confident decision based on data only to discover you were missing a crucial segment? That's sampling bias in action.
When your customer satisfaction metrics only capture feedback from people who completed purchases, you're blind to the frustrations that drove cart abandoners away. Similarly, product analytics often over-represent power users while missing the casual user experience entirely. This creates a skewed understanding that can lead product teams astray.
Market research suffers from this too. Many surveys over-sample certain demographics while under-representing others, creating insights that don't actually reflect your target population.
3. Processing and transformation errors
Data's journey from collection to analysis is fraught with transformation hazards:
- Aggregation gone wrong: ETL jobs might combine incompatible measures (like summing percentages) or apply incorrect grouping logic
- Join problems: Improper join conditions create duplicate records that silently inflate your metrics
- NULL handling surprises: When your calculations treat NULL differently than zero, unexpected results quickly follow
I've seen entire quarters of financial reporting thrown into question because of a single transformation error that went undetected. And the most dangerous part? The data often "looks" perfectly reasonable at a glance.
4. Definitional misalignment
This might be the most insidious cause of all—when different teams or systems have fundamentally different ideas about what the data represents.
"Active users" becomes a battleground metric when the product team defines it as "logged in at least once" while marketing counts only users who performed specific actions. "Revenue" in some reports includes refunds while other reports exclude them. And time periods become a special kind of chaos when some systems use calendar months while others use 30-day rolling windows.
Without aligned definitions, you end up with technically correct data that leads to spectacularly wrong conclusions. I've witnessed entire meetings derailed when two executives brought conflicting reports using the same metric names but different underlying calculations.
Examples of invalid data
Invalid data can be caused by a variety of issues, such as data entry errors, system glitches, or even intentional falsification. Here are a few examples of how invalid data can negatively impact business analytics:
- Data Entry Errors: Imagine you're a sales clerk that's accidentally scanned an item twice. This issue make its way into the downstream warehouse, inflating the total revenue number for the day.
- System Downtime: Using the example above, the POS system has gone down, leading to an inability to get revenue numbers for the day, leading to incorrect revenue numbers for the month.

- Intentional Falsification: In the final scenario, a VP of Sales is responsible for the monthly revenue numbers, and manually changes an input to give the appearance of hitting the numbers. In this case, the reporting numbers given to the board may show success, but contain invalid data.
Addressing data validity issues is crucial for ensuring data quality and reliability. Businesses need to implement clear data standards and utilize data quality management software to regularly update validation rules and corrective measures. This helps maintain the integrity of business analytics and supports better decision-making in data-driven environments.
How do you measure data validity?
As with any aspect of data quality, it's essential to have metrics in place to measure data validity. Here are some real-world metrics that data teams commonly use to measure data validity:
- Completeness rate: The percentage of expected data that is present in a dataset.
- Accuracy rate: The percentage of data that is correct.
- Timeliness rate: The amount of time that elapses between the occurrence of an event and the data's inclusion in the dataset.
By tracking these metrics over time, data teams can identify trends or issues that may need to be addressed.
How to ensure data validity
There are several best practices that data teams can follow to ensure data validity, including:
- Use data validation rules: Implement a set of rules that data must meet before it can be input into a system. This can include things like field length requirements or data type limitations.
- Role of anomaly detection: Utilize anomaly detection tools to identify data points that fall outside the expected range. This can help identify data quality issues quickly.
Final thoughts
Data validity is a critical aspect of data quality that data teams must prioritize. Ensuring data validity can help businesses make informed and accurate decisions that can ultimately impact the bottom line.
Data observability tools like Metaplane improves data validity initiatives by continuously monitoring and validating data quality from the warehouse down to usage in the business intelligence tool, continuously retraining based on your actual data and processes.
Editor's note: This article was originally published in May 2023 and updated for accuracy and freshness in April 2025.
Table of contents
Tags
...

...

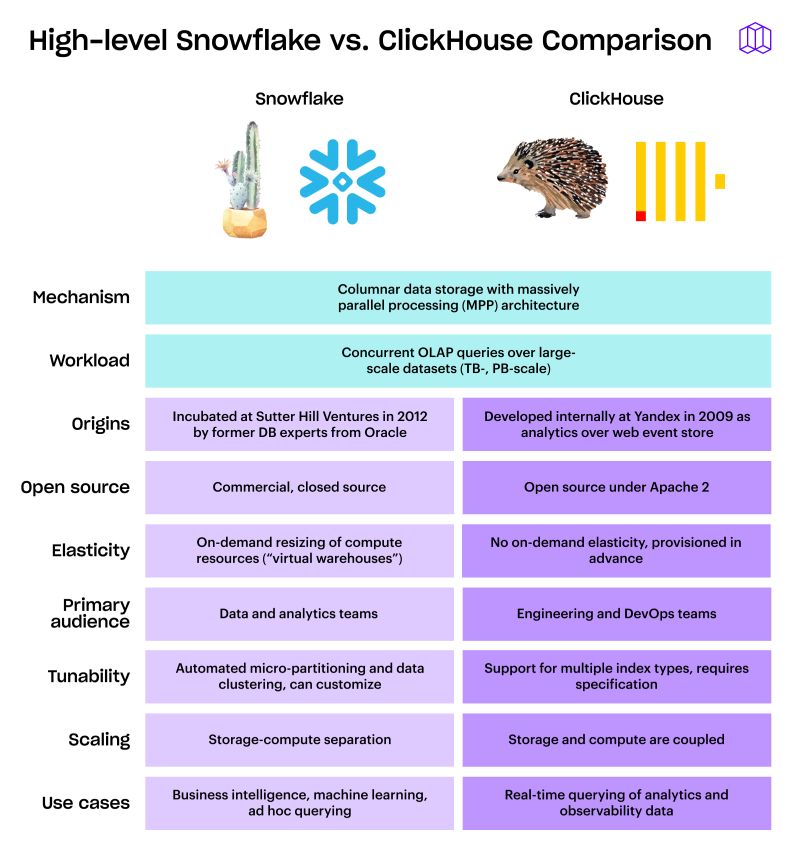


