Data Quality Fundamentals: What It Is, Why It Matters, and How You Can Improve It
Data quality has a massive impact on the success of an organization. In this blog post, we highlight what it is, why it matters, what challenges it presents, and key practices for maintaining high data quality standards.
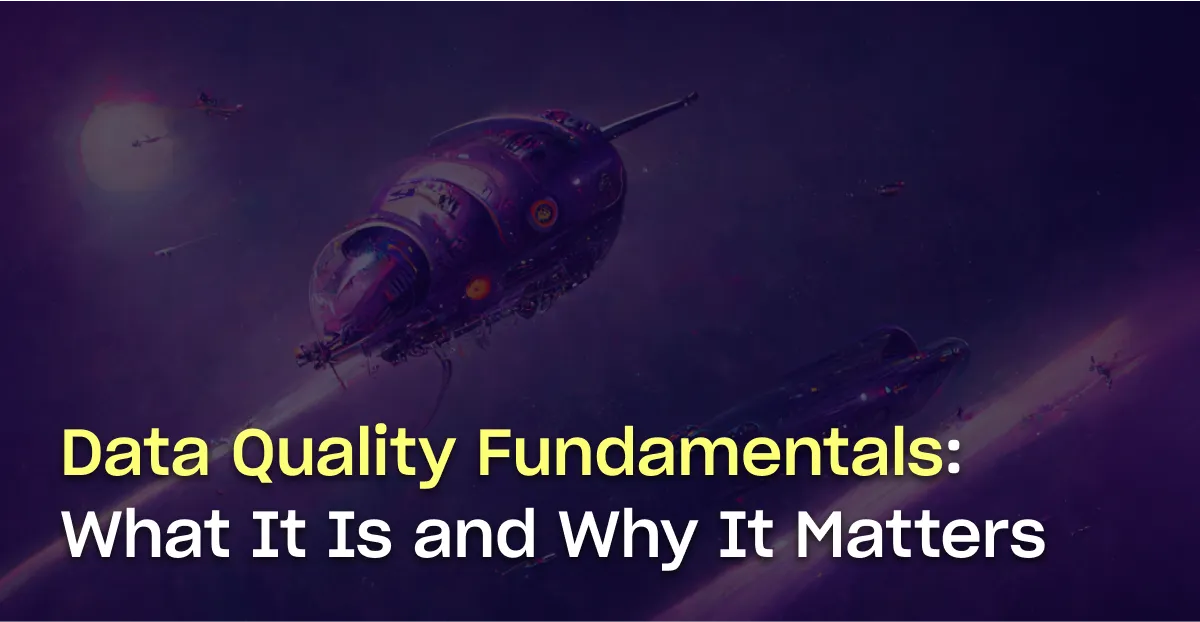
Data quality matters. In 2021, problems with Zillow’s machine learning algorithm led to more than $300 million in losses. A year earlier, table row limitations caused Public Health England to underreport 16,000 COVID-19 infections. And of course, there’s the classic cautionary tale of the Mars Climate Orbiter, a $125 million spacecraft lost in space—all because of a discrepancy between metric and imperial measurement units.
It’s real-world examples like these that emphasize how easily inconsistent data and poor data quality can impact even the biggest organizations. No wonder data teams are in such high demand. But supplying quality data stakeholders can trust isn’t easy. In reality, it requires that data teams master key data quality standards and concepts. Only then can they deliver on their mandate.
In this article, we highlight the fundamentals of data quality: what it is, why it matters, and how to measure and improve it.
What is data quality?
Before we get started, we need to understand a few key definitions:
- Data quality is the degree to which data serves an external use case or conforms to an internal standard.
- A data quality issue occurs when the data no longer serves the intended use case or meets the internal standard.
- A data quality incident is an event that decreases the degree to which data satisfies an external use case or internal standard.
There are ten dimensions of data quality that exist across two categories: intrinsic dimensions and extrinsic dimensions.
Intrinsic dimensions are independent of use cases, and include data integrity, accuracy, completeness, consistency, freshness, and privacy/security.
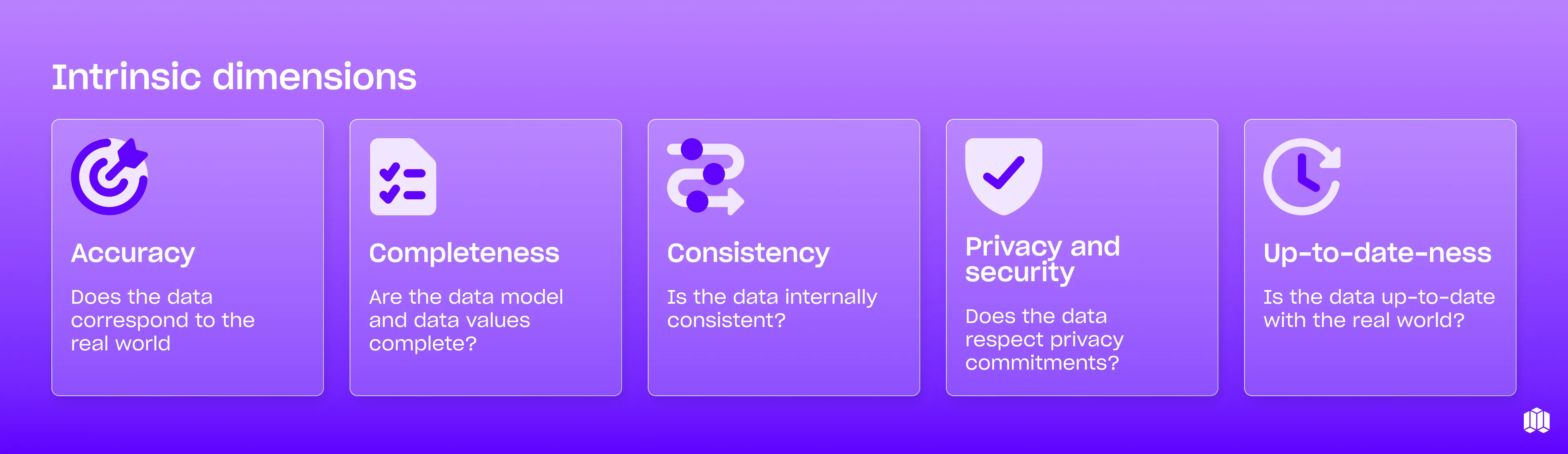
Extrinsic dimensions, on the other hand, are dependent on use cases, and include relevance, reliability, timeliness, usability, and validity.
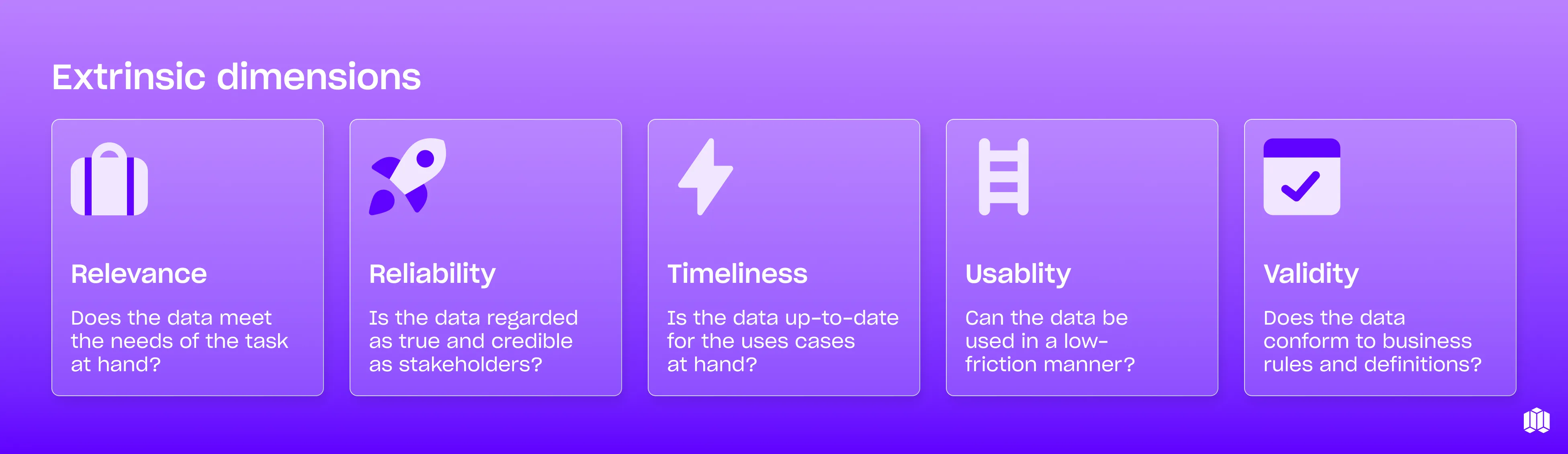
Why is data quality important?
Data quality matters because it directly impacts your business performance. High-quality data helps you make better decisions and perform better, leading to increased revenue, decreased costs, and reduced risk. Low-quality data has the opposite effect, resulting in poor profitability and an increased risk that your business will fold prematurely.
All companies rely on data to determine their product roadmaps and deliver exceptional customer experiences. That’s precisely why high-quality data (and therefore data quality) is important absolutely crucial for making the right decisions for the right people at the right time. Among other things, it can:
- Make (or break) personalization efforts
Did you know that more than 75% of consumers get frustrated when companies don’t personalize their interactions? That’s a startling statistic, but there’s a lot of merit behind it. Just imagine logging into Spotify tomorrow, and instead of seeing recommendations based on your listening, you see completely generic playlists. I suspect we’d see a big uptick in Spotify’s user churn.
- Support or sabotage product development decisions
Decision-making is only as good as the data it’s based on. Something as simple as duplicate database rows could overstate the correlation between a feature’s performance and customer churn, leading the development team to prioritize a feature that doesn’t matter to many users. Without data to reflect what’s a “good” or “bad” feature, business teams (and the data teams that support them) are left blindly guessing at the needs of their users.
- Optimize or bloat sales and marketing campaigns
Even outside of “traditional” sales conversations, high-quality data impacts product sales by determining how accurate (or not) copy on product pages, pricing plans, and in-product is to the customer’s needs. And the same holds true for marketing teams, who rely on customer and usage data to help them build out user segments on platforms like LinkedIn and Facebook, understand the unique personas using the product, and create top-of-funnel content that speaks to those users' pain points.
- Build up or break down organizational trust in data
Data is only helpful if decision-makers trust it. And as of 2021, only 40% of execs had a high degree of trust in their company’s data (we can only hope it’s increased since then). But when business stakeholders catch data issues and have to go back to data teams for answers, it degrades their trust in their organizational data. Once that trust is lost, it’s hard to build back.
The top data quality challenges
Data quality problems span both machine and human errors. On the machine side, data teams often struggle with software sprawl and data proliferation. They also tend to lack the metadata they need to perform their jobs efficiently and effectively.
On the human side, data creators inevitably make typos and other data entry issues that reduce data quality, whereas data teams themselves have little context around business metrics. That makes it tough to spot problems in the data.
It’s difficult for data leaders to hire experienced team members, which results in reduced capacity for addressing data quality issues. Check out the below webinar to learn more about the most common data quality challenges businesses face.
Whatever your challenge is, resolving it starts by conducting a thorough data quality assessment (and a good data observability tool like Metaplane can help you with that).
How to measure your data quality
Measuring your data quality is easiest when you start with the proper guardrails. Here’s a simple data quality management framework to get you started.
- Nail down what matters
What are you measuring, and why? Does your organization use data for decision-making purposes, to fuel go-to-market operations, or to teach a machine learning algorithm? Most likely, getting to this answer will involve a combination of talking with the business stakeholders that use the data your team prepares and using metadata like lineage and query logs to quantify what data is used most frequently.
💡Pro tip: A feasible starting point is to focus on the most important and impactful use cases of data at your company today. Identify how data is driving toward business goals, the data assets that serve those goals, and what quality issues affecting those assets get in the way.
- Identify your pain points
Do you struggle with slow dashboards or stale tables? Perhaps it’s something bigger, like a lack of trust in your company’s data across the organization. The main takeaway here is that pain points vary widely depending on the nature of your business, the maturity of your data infrastructure, the specific use cases for your data, and more.
Remember that the data team is neither the creator nor the consumer of company data. We’re the data stewards. While we may think we know the right dimensions to prioritize and the right metrics to track, we need input from our teammates to make sure we’re not wasting our time with extrinsic dimensions that aren’t timely, or intrinsic dimensions that aren’t relevant to use cases.
- Make metrics actionable
From these causes of recent trouble, which data quality dimensions are relevant and how can you measure them?
Say, for instance, you’ve implemented consistent data metrics to track how many of the sales team’s issues are related to data consistency. In response, you can develop a uniqueness dashboard of primary keys' referential integrity to check if deduplication is needed. But if the dashboard data isn’t connected to the completeness issues faced by the data team, the team won’t understand these numbers or why they’re important to each team’s initiatives. Make that obvious from the get-go.
4. Measure, then measure some more
Measuring data quality metrics is the last step in the process. Take data accuracy, as an example. You can measure this by comparing how well your data values match a reference dataset, corroborate with other data, pass rules and thresholds that classify data errors, or can be verified by humans.
And if you’re thinking, “But there are so many ways to turn these metrics into numbers!”—you’d be right. Despite what you may think, there is no single right way to measure data quality. But that doesn’t mean we should let perfect get in the way of the good.
How to manage data quality incidents
No matter the people, processes, and technology at your disposal, data quality issues will inevitably crop up. To manage these incidents, you can follow our six-step data quality management process: prepare, identify, contain, eradicate, remediate, and learn.
- Prepare: Get ready for the data quality incidents you’ll inevitably deal with in the future.
- Identify: Gather evidence that proves a data quality incident exists and document its severity, impact, and root cause.
- Contain: Prevent the incident from escalating.
- Eradicate: Resolve the problem.
- Remediate: Return systems to a normal state following an incident.
- Learn: Analyze both what went well and what could be done differently in the future.
How to improve your data quality
At its simplest, achieving superior data quality requires a applying a People, Process, Technology (PPT) framework. Let’s break it down:
- People: Your teammates can (and should!) help you develop data quality metrics from the ground up. Once these metrics are tangible, you’ll also need their help to hold the organization accountable for meeting those metrics and play their part in ensuring high quality data. For instance, a data quality engineer, data steward, or data governance lead could own the implementation and improvement of data quality metrics.
- Process: Think about what business processes should you put in place for data quality improvement. The lowest lift would be for the data team could perform a one-time data quality assessment. Or, for ongoing improvement with a higher lift, you can orient the entire organization around data quality. For instance, you could implement quarterly OKRs around data quality metrics; the marketing and sales teams could introduce data entry training initiatives around data accuracy and data validity; and the data team could implement playbooks for remediation.
- Technology: Technologies can be your biggest advantage when improving your data quality. For example, tools like Segment Personas and Amplitude can help you guarantee that your product analytics data is consistent and reliable, while ELT solutions like Fivetran and Airbyte and Reverse ETL solutions like Hightouch and Census use automation to check that all the data from your data sources is both up-to-date and timely in real-time. Plus, there are literally dozens of data quality tools meant for data quality measurement and data quality management—not to mention data cleansing tools for fixing data values.
Ultimately, you have to commit to best practices, like regularly conducting data quality checks and having a streamlined process for responding to data quality incidents. And, in tandem, you need to be proactive. Provide data creators with data entry training and periodically conduct data quality audits. Create the data infrastructure required to monitor your data quality as it ebbs and flows over time.
Carried out consistently, these practices, in conjunction with a data observability tool like Metaplane, can help you improve your data quality across your organization. Book a demo to see how Metaplane can help you build trust in your data, detect and fix problems quickly, and monitor what matters most to you.
Table of contents
Tags
...

...

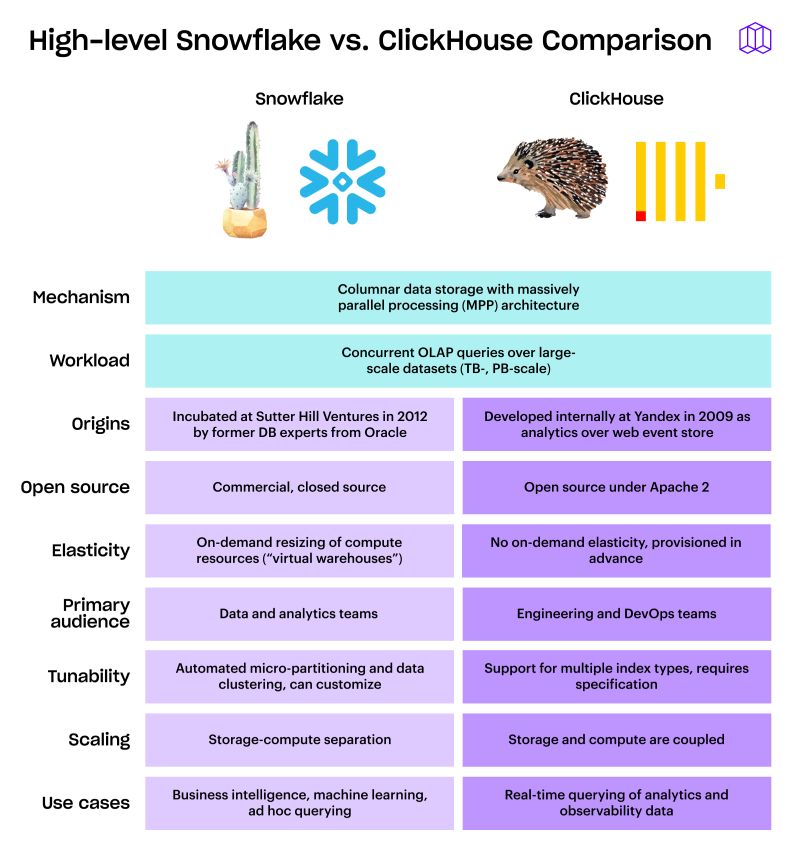


